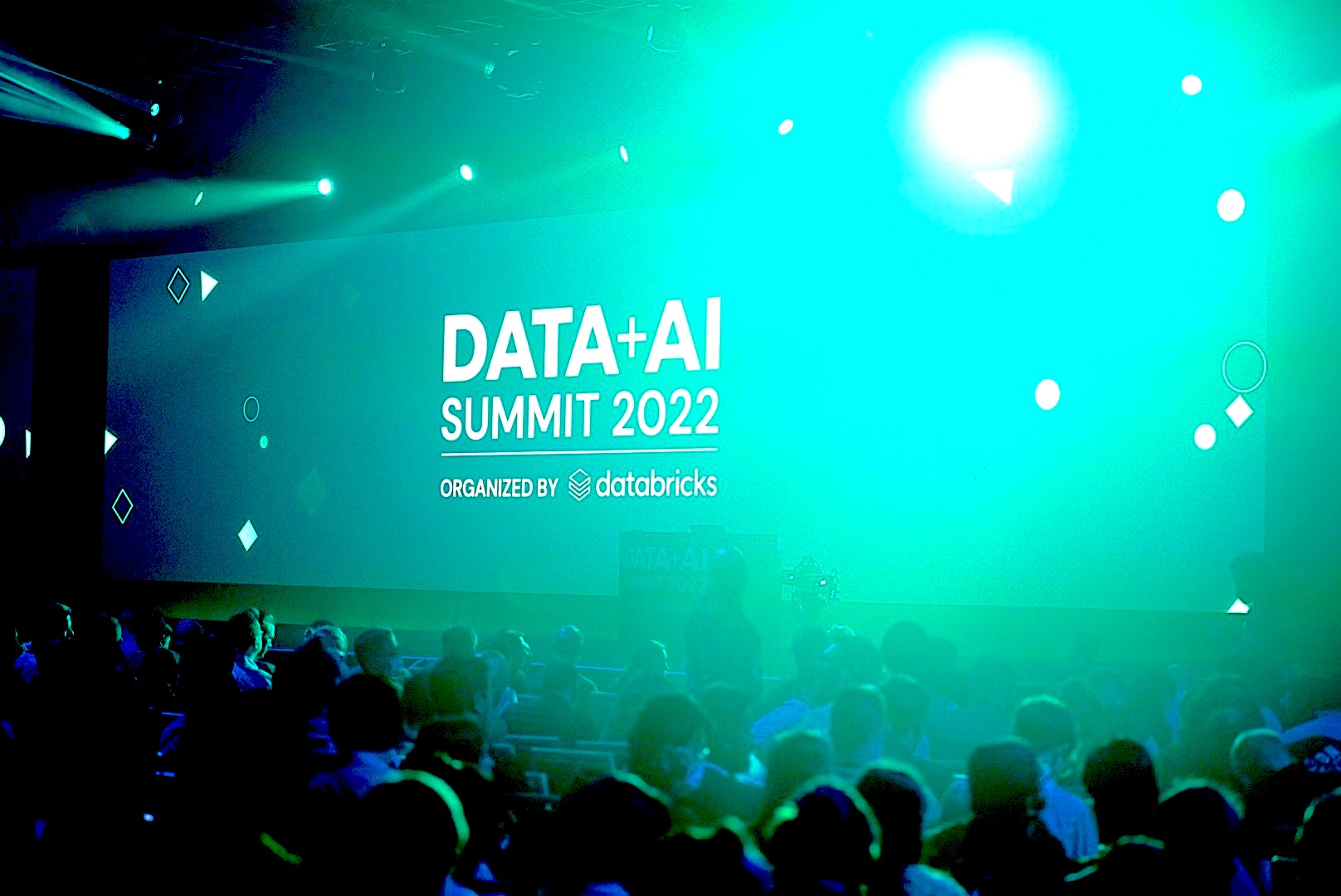
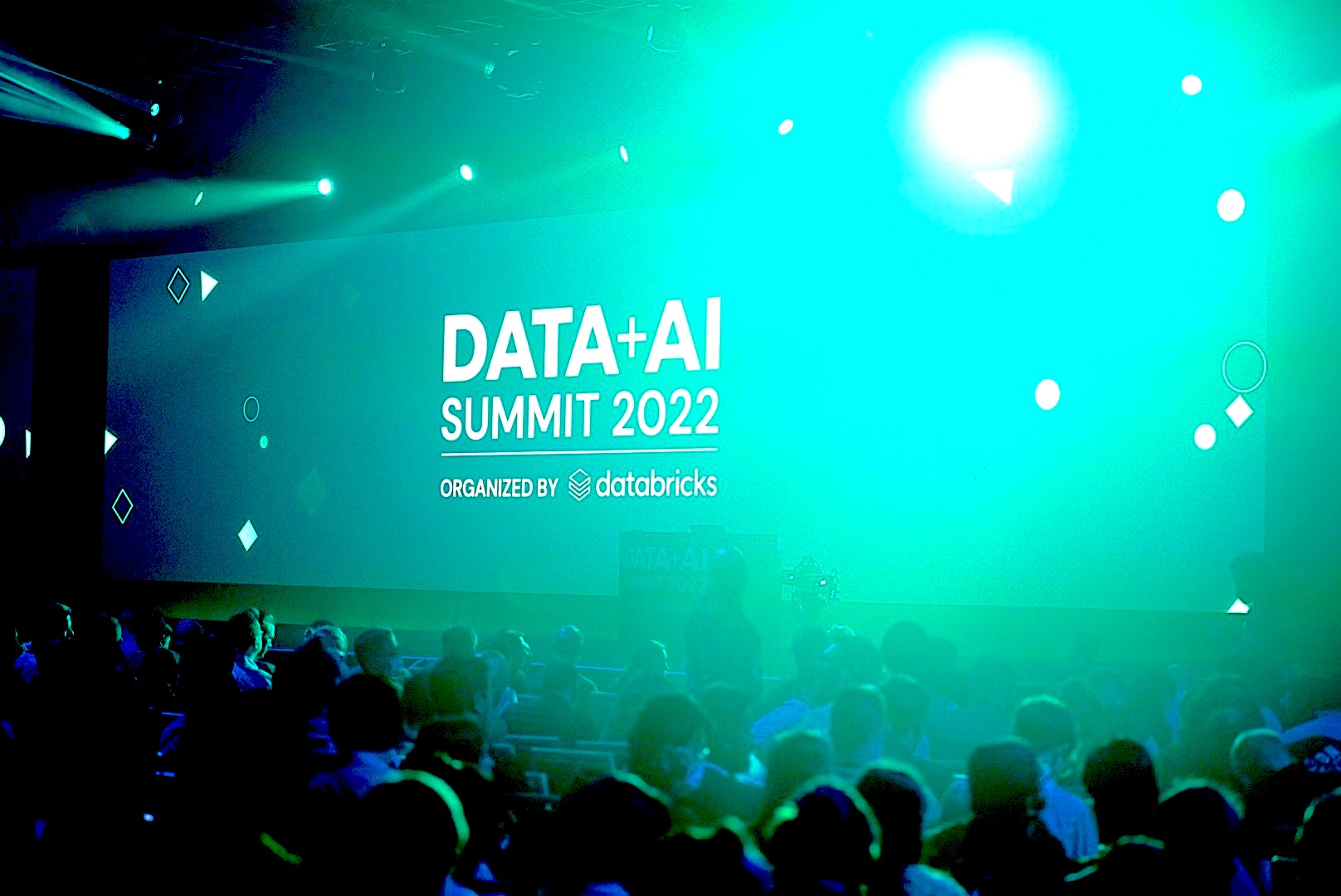
Our analysis and end-user discussions continue to demonstrate that a new modern data stack is emerging along with sophisticated data-oriented “personas,” such as data analysts and data scientists.
Discussions with data professionals in leading organizations show that Databricks Inc. is heavily influencing these emergent personas. We believe Databricks is embarking on a mission to broaden the adoption of artificial intelligence-powered applications and at its Data + AI Summit, we expect the company to unveil plans to simplify its data stack, setting up further explosive growth.
Databricks recently revealed it grew 60% year-over-year, with sales topping $1 billion, and the firm’s data warehouse offering surpassing $100 million in annual recurring revenue. As well, in an effort to reshape AI infrastructure, the company announced the acquisition of Rubicon Inc., an AI storage specialist founded by former Google LLC and Dropbox Inc. engineers.
Databricks has been a powerful force, catalyzing a group of data leaders who are building a new breed of applications to leverage the intelligence embedded within all the data they’ve collected. Notably, these are not off-the-shelf applications and are, in many cases, the organization’s intellectual property. Proprietary data is what differentiates these systems, and we believe Databricks is in a good position to leverage this dynamic and solidify its leadership.
Customers we speak with want to consolidate or gain control over the data flowing into their organizations. Although consolidating all the data into one solution is still, technically speaking, a “data pipe dream,” larger, integrated data silos are achievable. Usually this forces organizations into various types of warehouses or data lakes.
The components of the modern data stack comprise data sources, integration, warehousing, transformation, preparation, visualization, security and governance. Typically, these activities are supported by many products supplied by more than one company. Moreover, each activity might have multiple vendors or open-source components needed to create a complete picture of the data to answer the question.
With the rise of data engineers and data product manager personas, building applications off first-party data, combined with in-house modeling, is becoming the dominant data-product approach. Moreover, building applications from many different data sources or nested data products is becoming the norm.
And the ability to repurpose that data without making multiple copies is mandatory. Further adding to the complexity are privacy regulations such as the EU’s General Data Protection Regulation, the California Privacy Rights Act, the California Consumer Protection Act and Virginia Consumer Data Protection Act. These regulations impact every company, irrespective of physical location.
Organizations are prioritizing the avoidance of intellectual property leakage and avoiding the use of commercial large language models or LLMs. Building their own systems is the only way to ensure a competitive advantage. Databricks is positioned with a solution for this objective and is poised to continue their leadership position in this area.
Databricks seamlessly integrates with popular tools and frameworks used in the data science ecosystem, including programming languages such as Python, R, Scala and SQL, as well as libraries and frameworks such as TensorFlow, PyTorch and scikit-learn. This makes the integration with existing data science workflows a breeze.
At a recent end-user event for Snowflake Inc. and some of its partners, it was notable how little discussion focused on AI or LLMs. This was not to say that these companies, many which were a mix of established companies and startups, were not building and using AI. Some had version one of their “modern data stacks” deployed. Many were discussing revisiting certain aspects of their data stacks.
It’s more that Snowflake is not seen as the place for AI operations, or AIOps, even with its Snowpark product extension. In contrast, all the talk at the last few Databricks end-user events has been about AI, and many of these were before the explosion of ChatGPT. Databricks is really good for specific data workloads, as is Snowflake.
That’s why we see through our partner Enterprise Technology Research that the overlap in accounts with 36% of Snowflake customers also having Databricks. Snowflake is a SQL-first data warehouse at its core. The business analyst persona and ad-hoc queries tend to be the majority of applications built on top of Snowflake, such as business intelligence or inventory analysis, whereas Databricks has grown from a Python-first data lake product. This has led to Databricks being strong out of the gate with workloads that are code-first data products, such as product recommendation engines.
Databricks will host its Data + AI Summit in San Francisco June 26-29. We expect three focus areas to watch at this key event:
AIOps functionality. AIOps functionality will likely be added to Databricks and the underlying Apache Spark. The importance of this capability is that it will solidify Databricks’ position in the commercial side of data lakes. Open source underpins Databricks’ product strategy and the company has effectively used its position in open-source software to market against competitors such as Snowflake.
Nonetheless, Databricks will have to prove it can be a catalyst for the AI ecosystem. A scan of the agenda at summit shows a major focus on LLMOps and how to deploy on Spark. Databricks has had success building an ecosystem of “horizontally in the data stack” data source companies, particularly customer data platforms or CDPs. But these CDP players are also building on or partnering with Snowflake, Amazon Web Services Inc. and Google LLC, all of which pose formidable alternatives.
It is all the rage for companies out there to vertically integrate, such as in the CDP market. Others focus on warehousing, transformation, preparation and visualization aspects of the modern data stack, such as Databricks with Delta Lake and Lakehouse. This leaves openings for a robust ecosystem.
That said, Databricks seems to be taking a page out of Microsoft Corp.’s and Snowflake’s playbook with “minor” investments in some of these players. For instance, it just announced it’s investing in Snowplow Analytics, an open-source CDP data source provider, as part of the partnership and marketplace integration requirements. How this plays out for a company such as Snowplow, which is also partnered with Snowflake, and whether there’s a tailwind, remain to be seen.
Data quality. Today, data modeling is done with another open-source-turned-commercial product in dbt labs. If you are building tables and views in Databricks (Spark), Snowflake, Google BigQuery, Amazon Redshift, or Postgres, you will most likely use dbt. Dbt Core is the most utilized data transformation and modeling tool. One of the reasons for this is that it is an open source project consisting of python packages that can easily run on a laptop.
As well, dbt Cloud is the commercial offering from dbt Labs Inc., that is built on the dbt Core, with a user interface for those who need to become more familiar with running Python scripts. Dbt is compelling because it’s where data engineering on a warehouse or data lake is done. We have yet to see Databricks bring out a first-party solution for this, and ride with dbt. We would expect Databricks to move into this space for ease of use as data quality becomes harder across multiple data repository silos in the Lakehouse.
How that affects partners such as dbt labs will be an key point of ecosystem discussion if Databricks goes down this path. Importantly, this ties back to the regulations mentioned earlier. As data continues to be utilized in multiple applications, compliance must be maintained. The logical spot is when data is transformed on top of the data repository.
We surmise this as the reasoning behind acquiring Okera Inc., an AI-focused data governance platform — bringing more automation, AI intelligence and ease of use to data quality, which is critical. This becomes especially true given the type of data products built in code on top of Databricks, where the relationships of who has access to what data is critical.
Governance. A third theme to watch is that of data governance. This is heavily tied to the data quality and another reason for acquisitions in the space. One significant requirement for partnering with Databricks is to integrate with the Databricks Unity Catalog, the company’s unified governance solution. This capability will enable Databricks to continue building data governance that works consistently across its ecosystem. The importance of this capability cannot be understated, especially for industries looking to monetize their data via data clean rooms.
In support of this theme, Databricks made several announcements over the past year, including last year’s Delta Sharing and expanded use of Delta Live Tables. Many companies are looking to personalize their interactions with customers or answer similar questions in different industries and are looking for ways to use personally identifiable information-friendly sharing to gain a complete picture of the customer.
We believe there are four keys factors that observers should focus on at the upcoming Databricks summit as indicators of progress:
In the middle of last decade, evidence of a new data stack became more clear with cloud data platforms that separated compute from storage, simplified data warehousing and brought AI and machine learning to the table. It was very common to have departmental deployments comprised of AWS databases, some using Snowflake data warehouses and yet other departments using Databricks. And sometimes, this would occur all in the same department based on the developer or company preferences.
Fast-forward to the end of the decade, and the massive data management market opportunity pointed to a collision course between Snowflake and Databricks to become the dominant platform on which to build modern data apps.
Snowflake is coming from a position of strength in analytics, Databricks from a strong position in data science, and each firm is rapidly innovating to capture the opportunities ahead. Both are vertically and horizontally building and buying features to cover as much of the market as possible.
THANK YOU