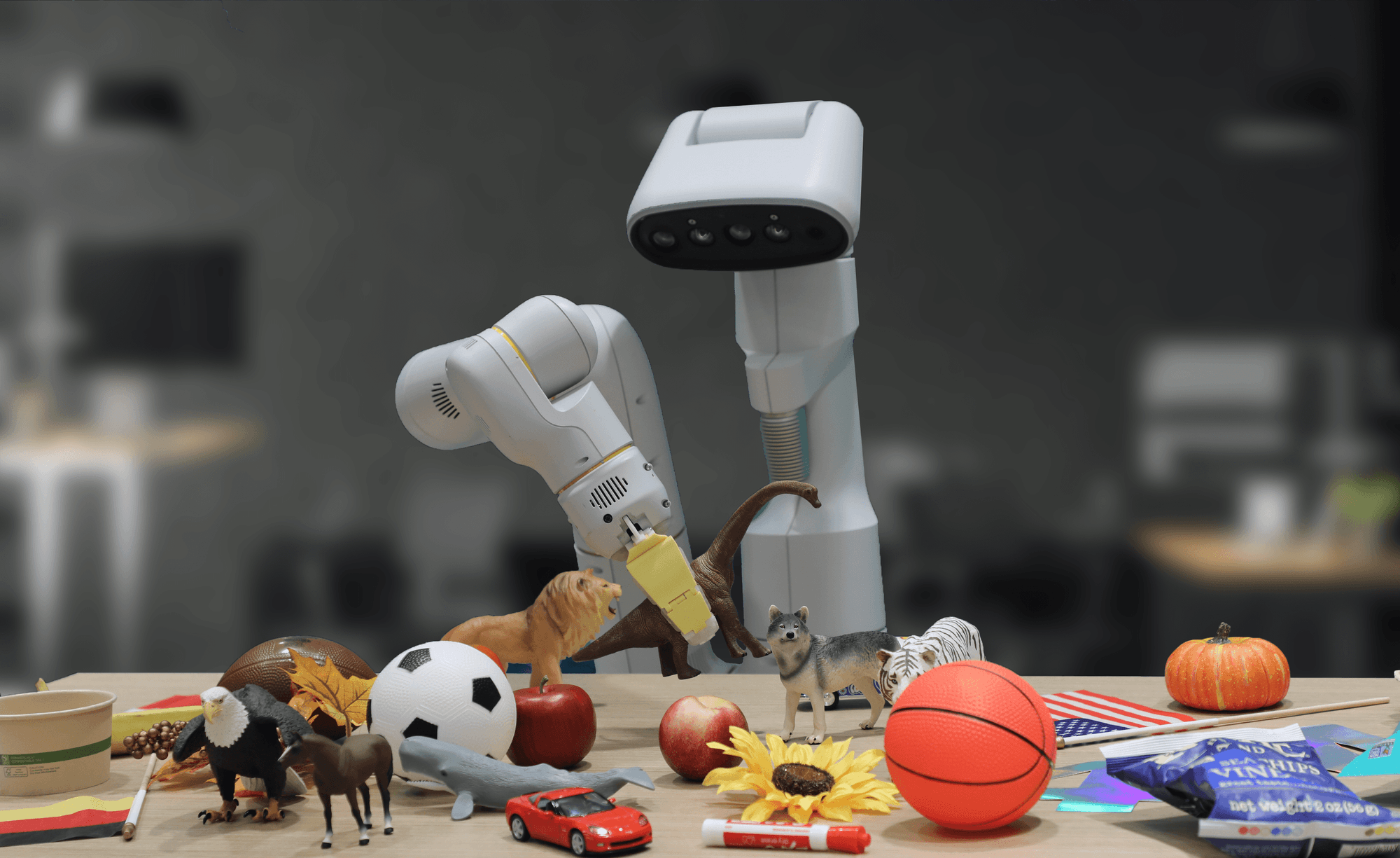
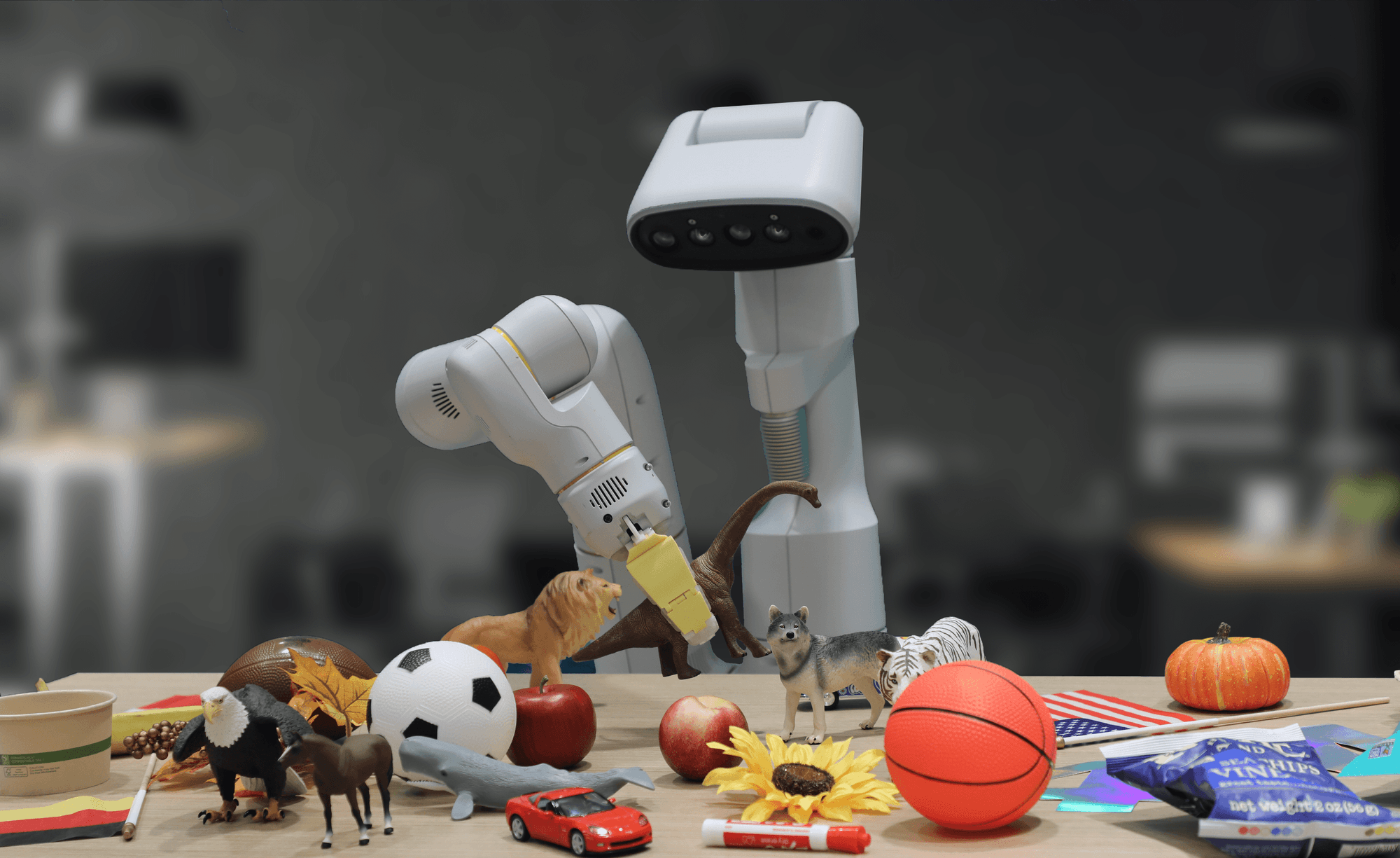
Google LLC today unveiled a new artificial intelligence model that will allow humans to speak to robots and tell them what to do by transforming words into action.
The new model, called Robotics Transformer 2, or RT-2, is capable of learning from both words and visuals in order to understand ideas and concepts in order to translate these into robotic actions such as picking up objects or triggering actions. Google introduced the new model in a blog post today stating that the company is looking to make robots more helpful.
“The pursuit of helpful robots has always been a herculean effort because a robot capable of doing general tasks in the world needs to be able to handle complex, abstract tasks in highly variable environments — especially ones it’s never seen before,” said Vincent Vanhoucke, distinguished scientist and head of robotics at Google DeepMind.
The new system for training robots to be able to listen to words and transform them into action, RT-2, is part of what Vanhoucke calls a novel type of AI system called a vision-language-action model. It’s capable of learning from both visual web and robotics data and can turn this data into instructions for robotics control. It can also provide a chain of reasoning based on the instructions to perform a series of tasks, such as being asked to pick up an object and place it somewhere — say, throw away trash in a bin — or pick select a snack for someone who is tired, such as an energy drink.
Unlike large language models such as OpenAI LP’s ChatGPT or Google’s Bard, VLMs need to be able to combine both the semantic meaning of text and visual data together into a coherent and complex set of concepts in order to complete a task. This creates a whole new set of challenges for robotics engineers combined with the subsequent need to set up objectives for the robot so it can generalize needs based on a request.
In the “Please pick up the trash and throw it away,” example, the robot already has an idea of what trash is and what might be from a large corpus of training data. It will be able to see it in its visual field and it will also be able to identify a trash bin based on its knowledge. From there, translating the action of collecting the trash and throwing it away would be a simple mechanical task of tracking it visually, grabbing and proceeding to drop it into the trash bin.
Previous AI models would need to be trained to understand each of these concepts beforehand in order to proceed with the multi-stage logic of first identifying trash, then the bin and then throwing it away. With RT-2, it doesn’t need to have been explicitly trained on the task of identifying and discarding trash.
It can be even wilder than that, since what is trash? It could be crumpled paper, discarded wrappers or the torn-off tip of a straw wrapper. The AI doesn’t need to be specifically told to pick up these things. It can infer them from its dataset.
“Until now, robots ran on complex stacks of systems, with high-level reasoning and low-level manipulation systems playing an imperfect game of telephone to operate the robot,” said Vanhoucke. “Imagine thinking about what you want to do, and then having to tell those actions to the rest of your body to get it to move. RT-2 removes that complexity and enables a single model to not only perform the complex reasoning seen in foundation models but also output robot actions.”
Google previously introduced another AI visual model called PaLM-E. It helps robots make visual sense of their environments and also allows users to use voice commands for sequential tasks, which researchers used as part of the backbone for the new system. RT-2 also builds on a prior model called RT-1 with the aim of providing it web-scale capabilities for dealing with tasks it has never encountered before.
In its testing, Google said that across 6,000 trials RT-2’s model showed that it retained all the same performance from RT-1 on tasks already in its own training data, or tasks it had “seen” before. As for tests involving novel tasks or questions that it had never been prompted to do, it performed successfully 62% of the time, compared with 32% for RT-1.
“Not only does RT-2 show how advances in AI are cascading rapidly into robotics, it shows enormous promise for more general-purpose robots,” Vanhoucke said. “While there is still a tremendous amount of work to be done to enable helpful robots in human-centered environments, RT-2 shows us an exciting future for robotics just within grasp.”
THANK YOU