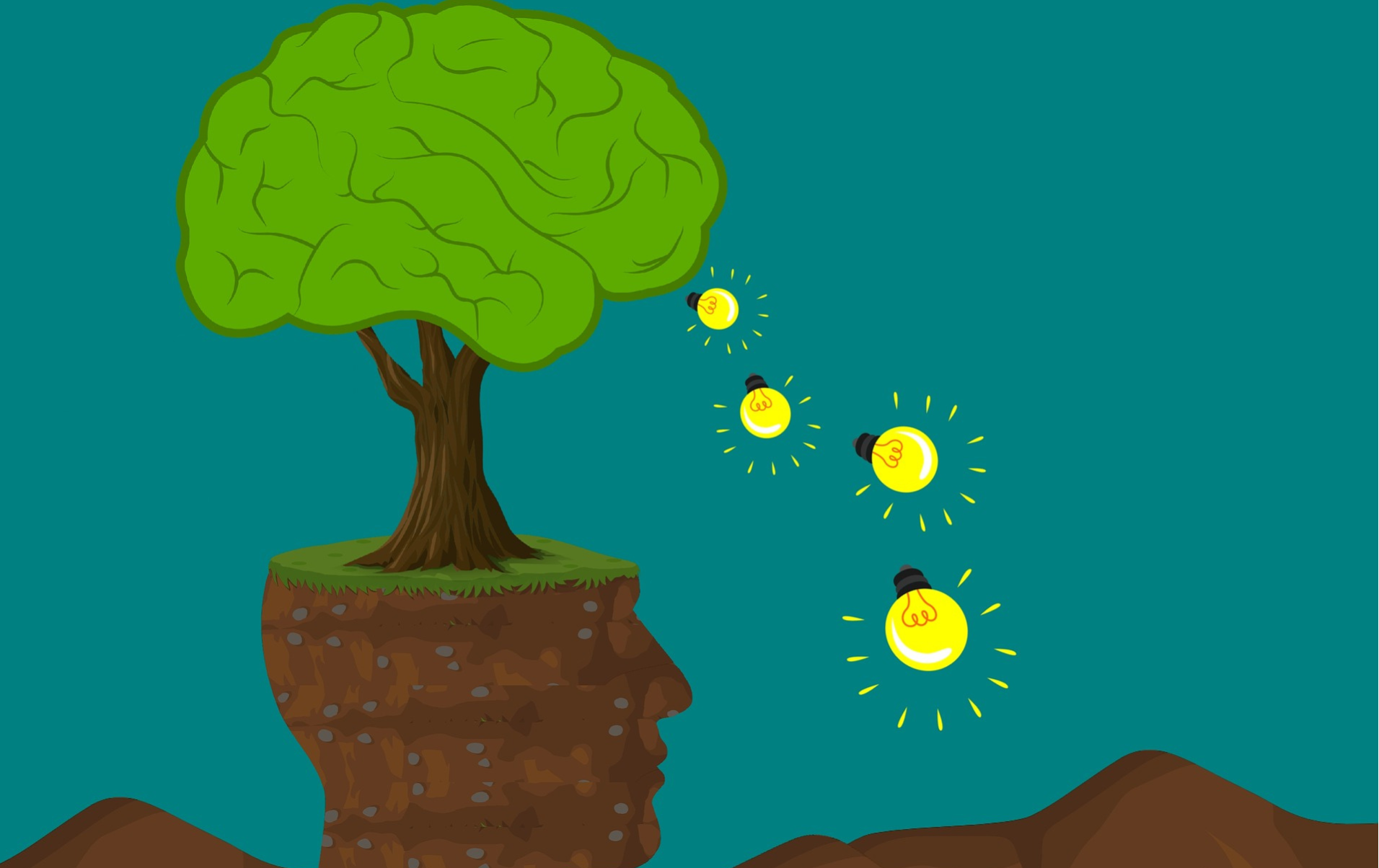
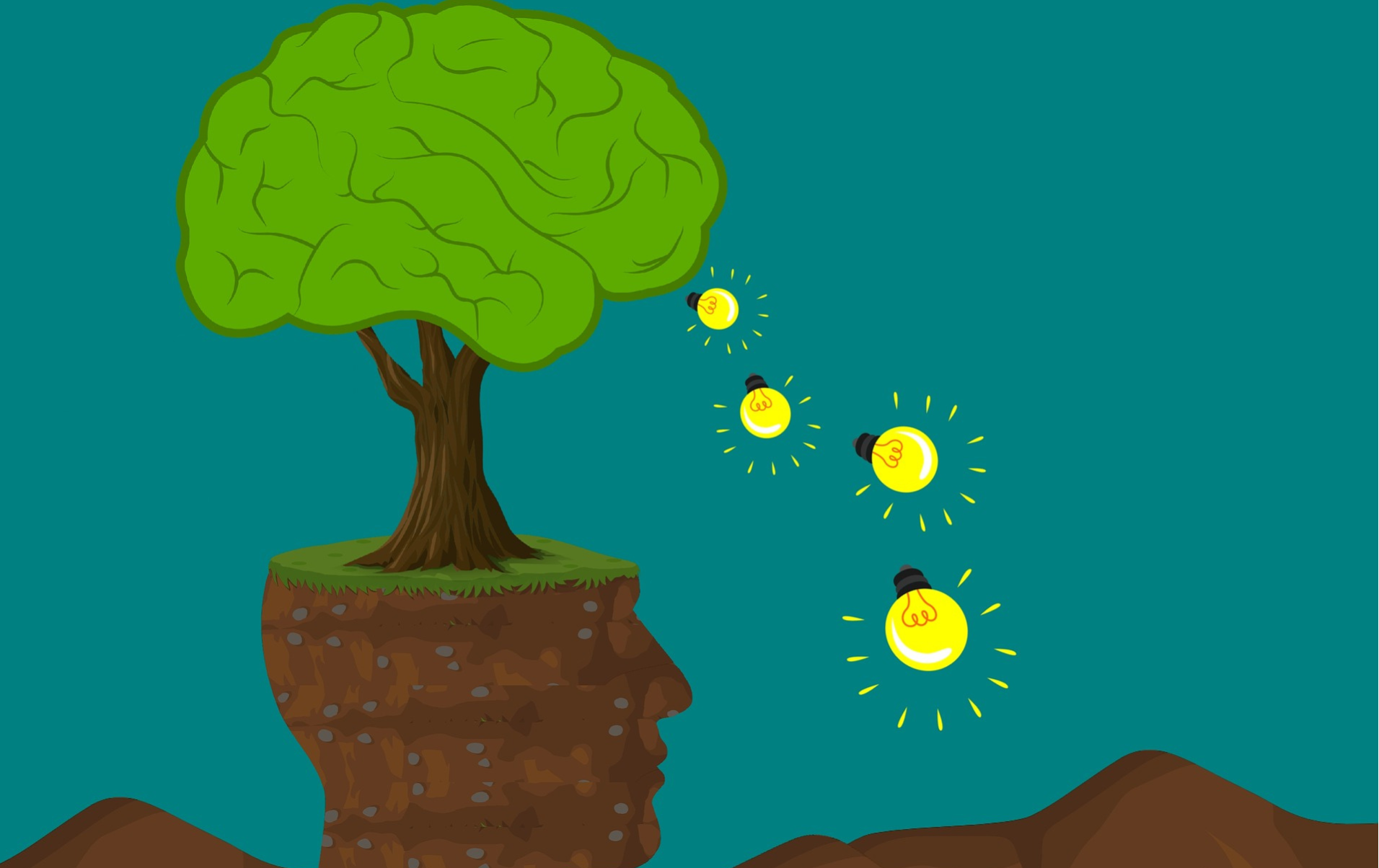
Artificial intelligence has the potential to create substantial business value for organizations, but AI teams often find it challenging to realize and communicate these benefits. In fact, Gartner research has found that difficulty measuring AI value and a lack of understanding around AI benefits and uses are top barriers to its implementation.
AI technologies are unique in that they can learn and adapt in complex ways. These are powerful attributes, but ones that can make it hard to predict the performance of AI models. This challenge will only intensify with the advent of generative AI — an impressive technology, but one riddled with hard-to-predict failure modes.
AI benefits are also difficult to plan for because they require business actions, as well as process and behavioral changes, that go beyond the direct control of AI teams. It can also be challenging to attribute benefits specifically to AI model outputs. This often results in a fundamental gap between AI model outputs and business benefits: Organizations could have the best AI models, yet still fail at delivering value.
Benefits do not happen by themselves. They need to be actively managed and monitored before, during and after AI model deployment. Thus, to derive tangible business benefits from AI projects, data and analytics leaders must implement five benefit realization best practices.
Before they begin, AI projects must obtain funding from the business. To sell the value of AI initiatives, data and analytics leaders must build a value story. Value stories essential to secure financing, drive adoption and create momentum for AI projects to scale.
A value story is a narrative that illustrates progress toward business outcomes. These stories are told from the perspective of stakeholders’ key priorities and communicate both financial and nonfinancial benefits tied to these priorities. They are also helpful in identifying the outcomes and key performance indicators that will define success for the AI project. A value story is not a traditional business case, but rather a compelling articulation of a project’s benefits.
Value stories can be supported by data, but they must be told in a compelling way that evokes emotion from the audience. They should start with the stakeholders’ priorities and conclude with the benefits to the organization.
AI teams must define a value hypothesis: an assumption about the improvement that the AI project will have on a specific business KPI. The hypothesis should flow from the value story, targeting a concrete KPI that is well-aligned to the top priorities of the organization. A hypothesis allows the AI team to remain focused on business value and to iterate toward a specific goal. It does not need to be complicated: A simple format such as “[AI use case] will increase/decrease [business KPI] by [X amount]” is enough.
The business KPI does not necessarily need to be financial. Solely focusing on financial metrics can make organizations miss critical investments in important projects that have longer-term, but strategic, impacts. Indirect metrics that can influence customer success, cost efficiency and business growth can also be impactful.
Capturing AI benefits will not happen automatically. AI teams must have a plan to go from an AI output to a set of actions and changes that will ultimately drive the business KPI. Develop a timeline for when and how AI will be applied to a specific business process and a prediction for how the outcome will be affected by AI.
It is crucial to avoid building this action plan in isolation, as business partners must be ready to execute on actions. The action plan should also include the training and incentive design necessary to ensure that the business adopts the AI models.
It is often challenging to isolate the effect that an AI project has on the target business KPI, because many factors outside the AI project also affect that KPI.
A/B testing is the standard approach to measure a change’s impact on KPIs. In A/B testing of AI, the new AI solution is applied to a randomly chosen subset of cases while a control group is maintained to compare business KPI performance.
However, A/B tests are not always possible or economically feasible. Many organizations that have been successful with AI have used attribution models — such as first-touch/last-touch attribution or latency models — to assign credit to actions taken because of the AI project.
Before fully deploying the AI model, AI teams must choose one or several approaches to test their value hypothesis. Iterating quickly, they must either prove or disprove that the AI use case resulted in the expected business benefit. This discipline is key to keep the focus of the team on benefit realization. Testing should be done as early as possible, with an objective to fail fast and iterate before spending too much time on a misguided effort.
The deployment and release of an AI model are only the start of AI benefit realization. To drive AI business value, it is essential that teams continuously monitor and act on two types of metrics:
AI teams must establish a monitoring system that includes leading and lagging KPIs, detects deviations from expected performance and acts on those deviations. Monitoring is particularly important for AI projects because model input “drift,” system performance and business operations can cause business benefits to dissipate.
Ultimately, this five-step process is iterative in nature. AI teams must build a value story, identify and test their hypothesis, and adjust continuously to improve performance both before and after deploying their AI models.
Leinar Ramos is a senior director analyst at Gartner Inc. covering AI, data and analytics and the creation of data-driven organizations. He wrote this article for SiliconANGLE. Gartner analysts will provide additional insights on driving business value through AI at Gartner IT Symposium/Xpo, taking place Oct. 16-19 in Orlando, Florida.
THANK YOU