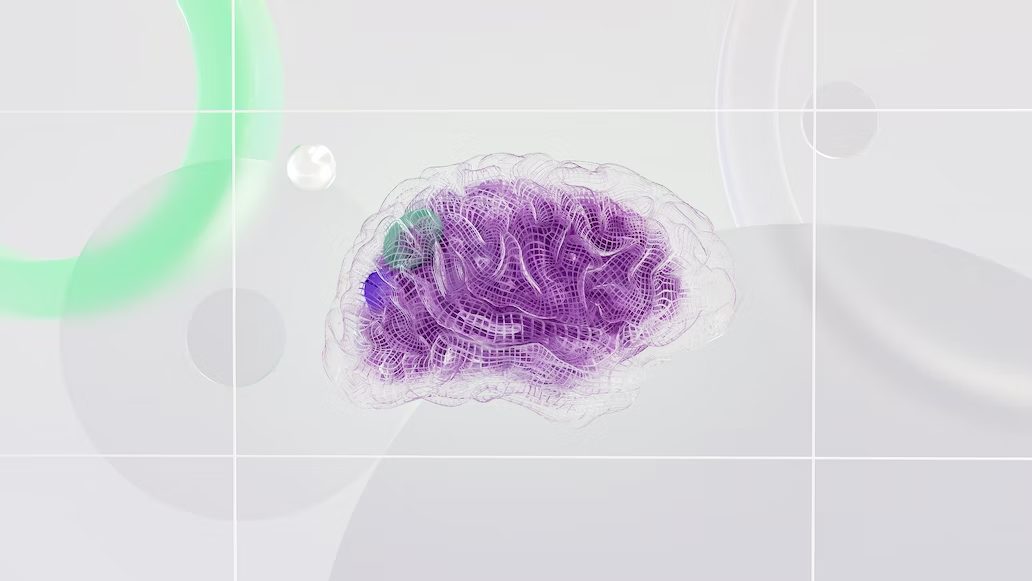
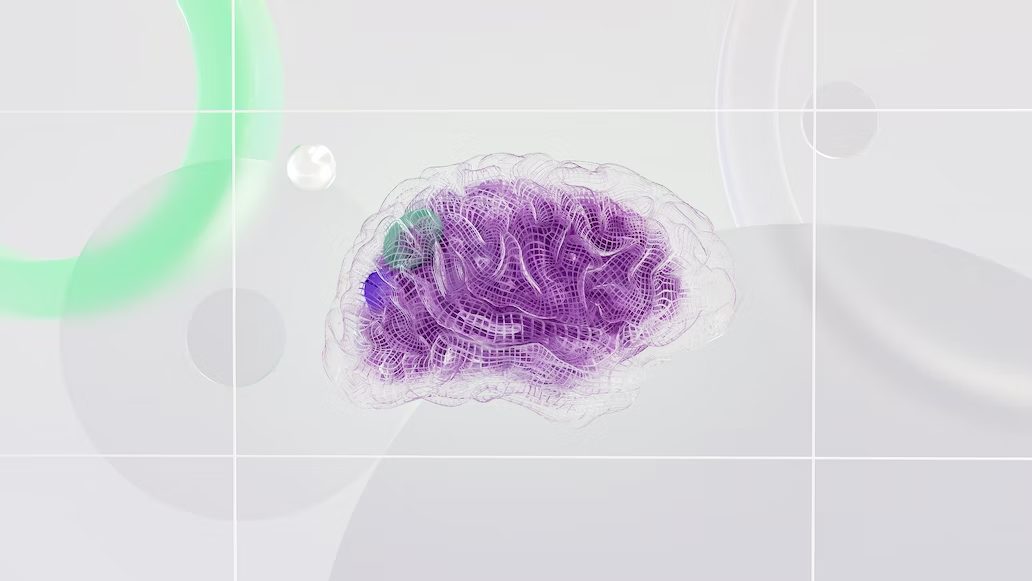
DynamoFL Inc., a startup with software that prevents large language models from leaking sensitive data, has secured $15.1 million in fresh funding.
The startup raised the capital through a Series A round it announced this morning. Canapi Ventures and Nexus Venture Partners were the lead investors. A number of other institutional and angel investors contributed as well.
A language model’s answers to user questions often incorporate information from the dataset on which it was trained. If the training dataset contains sensitive records such as credit card numbers, those records can potentially find their way into the model’s answers. That creates a risk of privacy breaches.
DynamoFL has developed a platform that addresses the challenge. According to the startup, its platform uses a method known as differential privacy to reduce the risk of data leaks.
Differential privacy allows a company to incorporate noise, or accuracy-reducing modifications, into a language model’s training dataset. Those modifications change the individual records that comprise the dataset. As a result, if those records were to be leaked by the language model, they wouldn’t pose a privacy risk because they were modified in a manner that makes them unusable.
Introducing privacy-protecting modifications to a training dataset is fairly simple from a technical standpoint. However, such modifications usually make the training dataset less useful for language model development. The primary innovation in differential privacy, the method employed by DynamoFL, is that it protects privacy without compromising the machine learning development workflow.
DynamoFL’s platform also promises to ease a number of related tasks. In particular, the platform uses an approach known as federated learning to reduce the cost of language model development. The technology also promises to improve security in the process.
Companies often train language models on data from multiple sources. A retailer, for example, may wish to train a neural network on sales logs stored in three different systems. Usually, that would require extracting the sales logs from each system and moving them to a centralized environment for processing.
DynamoFL’s federated learning technology removes that requirement. Instead of moving training data to a centralized environment for processing, the technology carries out training on the systems where the data is stored. As a result, there’s less need to shuffle information between different parts of a company’s infrastructure.
The company says that its technology provides multiple benefits. The fact that training dataset doesn’t have to be moved makes it easier to track and secure, Moreover, reducing data movement lowers the often steep bandwidth costs associated with transporting information between remote infrastructure environments.
“This investment validates our philosophy that AI platforms need to be built with a focus on privacy and security from day one in order to scale in enterprise use cases,” said co-founder and Chief Executive Officer Vaikkunth Mugunthan. “It also reflects the growing interest and demand for in-house Generative AI solutions across industries.”
DynamoFL says its platform has been adopted by multiple Fortune 500 companies in the finance, electronics, insurance and auto sectors. The startup will use its newly announced $15.1 million funding round to further expand its market presence. DynamoFL reportedly plans to hire 18 new employees by the end of the year to support the effort.
THANK YOU