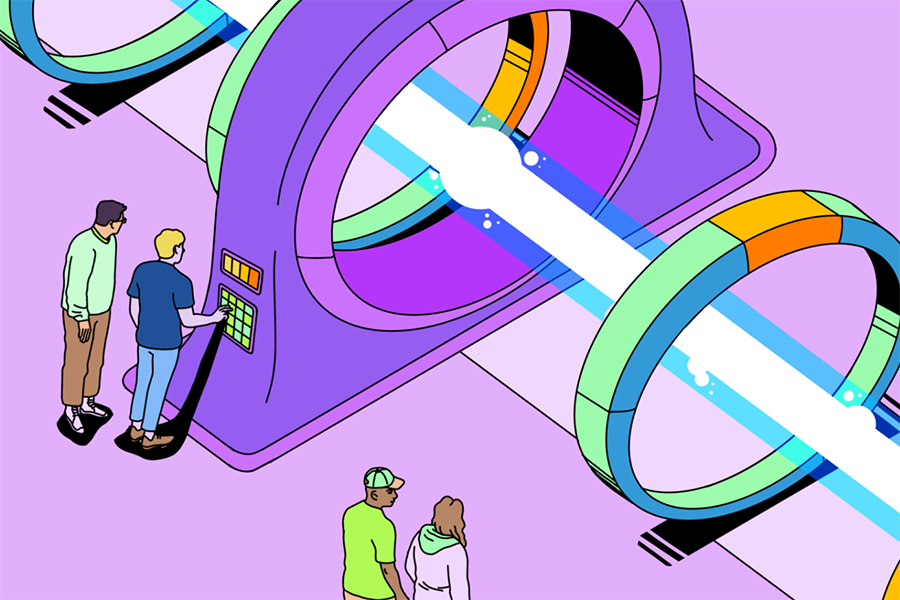
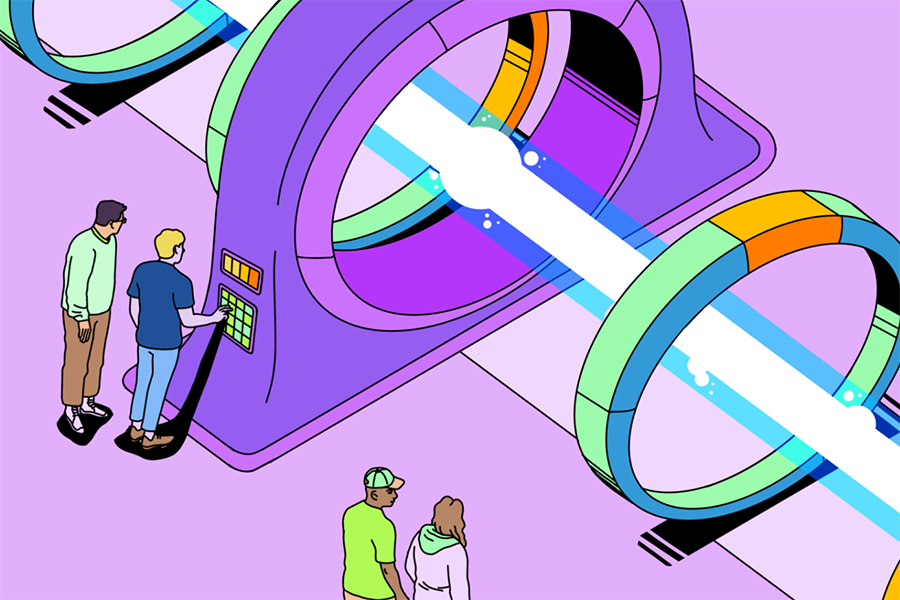
ArthurAI Inc., a startup that monitors and streamlines artificial intelligence and machine learning models, today announced the launch of Arthur Bench, an open-source tool that will help companies pick the right generative AI model based on their data and needs.
Bench is a tool for evaluating large language models, prompts and hyperparameters for generative AI text models, such as OpenAI LP’s ChatGPT chat bot. Generative AI text models can take large amounts of data and transform it by understanding conversational natural language prompts and responding with humanlike language to write research reports, summarize documents and answer questions.
However, not all LLMs are built equal. Some of them have been designed with particular types of logic or modalities in mind, such as being able to come to a particular answer swiftly based on a particular set of parameters or produce lengthy document summarization based on swaths of data. Others are lightweight and designed to be less costly to train and deploy, while yet other models have been developed to preserve the privacy of the users for regulatory compliance.
To assist in this evaluation, Arthur also unveiled the Generative Assessment Project. This research project ranks the strengths and weaknesses of LLM offerings on the market from industry leaders such as OpenAI, Anthropic and Meta Platforms Inc. and shares discoveries with the public about their metrics.
“As our GAP research clearly shows, understanding the differences in performance between LLMs can have an incredible amount of nuance,” said co-founder and Chief Executive Adam Wenchel. “With Bench, we’ve created an open-source tool to help teams deeply understand the differences between LLM providers, different prompting and augmentation strategies, and custom training regimes.”
In the GAP evaluation, Arthur looked at various available models to examine how well they handled challenging questions to which they wouldn’t immediately know the answers to see if they would “hallucinate.” That’s when an AI model produces false information and states it confidently.
The researchers also tested how well the AI models “hedged” their answers when asked for opinions — which is to say, they didn’t provide an opinion, which is what the model generally should do — or produced poor quality answers and other metrics. The results in GAP are continuously being updated as more research is being produced for the community to evaluate.
In addition to GAP, Arthur Bench helps companies evaluate models based on their needs. For example, if a company is integrating an LLM into an application that will answer simple text questions based on a knowledge base or responds to common customer queries, the user may not need an advanced LLM. There are also numerous models that have been developed for case-specific needs such as summarization of large documents or research that companies can benefit from by understanding their underlying data use and application needs.
Another company may need an in-house model to assist its coding and development team with software production, which would require evaluating different models capable of understanding different programming languages. In some of these cases, they might even benefit from bringing the AI model in-house, where they can manage the infrastructure and environment themselves to control costs and avoid proprietary knowledge from leaking to third parties. At the same time, the business would want to know which LLM would be most efficient and capable.
Most current LLM evaluation is done through academic benchmarks that don’t translate well to real-world scenarios. The power behind Bench is that it makes it possible for companies to test current existing models using their own data, so they can get a consistent benchmark to see how it holds up.
The release of Bench follows the launch in May of Arthur Shield, which acts as a “firewall” between the LLM and customers that helps identify potential problems such as data leakage, toxic or offensive activity and hallucinations before they become an issue. Shield can also help detect cybersecurity issues such as malicious prompts from users. That’s where users attempt to get the AI to say things that would make the company look bad, or prompt injection attacks that could be used to steal or expose sensitive information.
THANK YOU