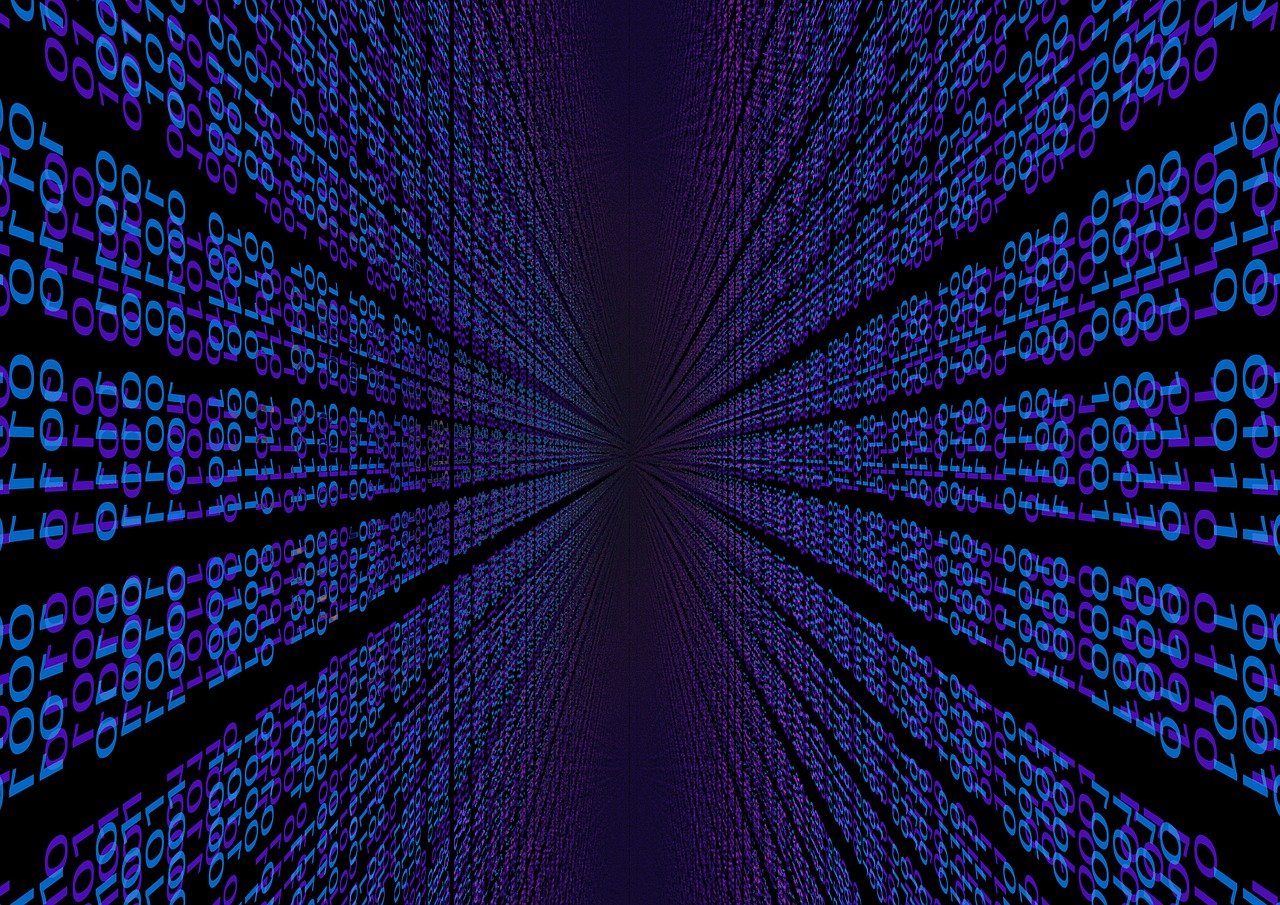
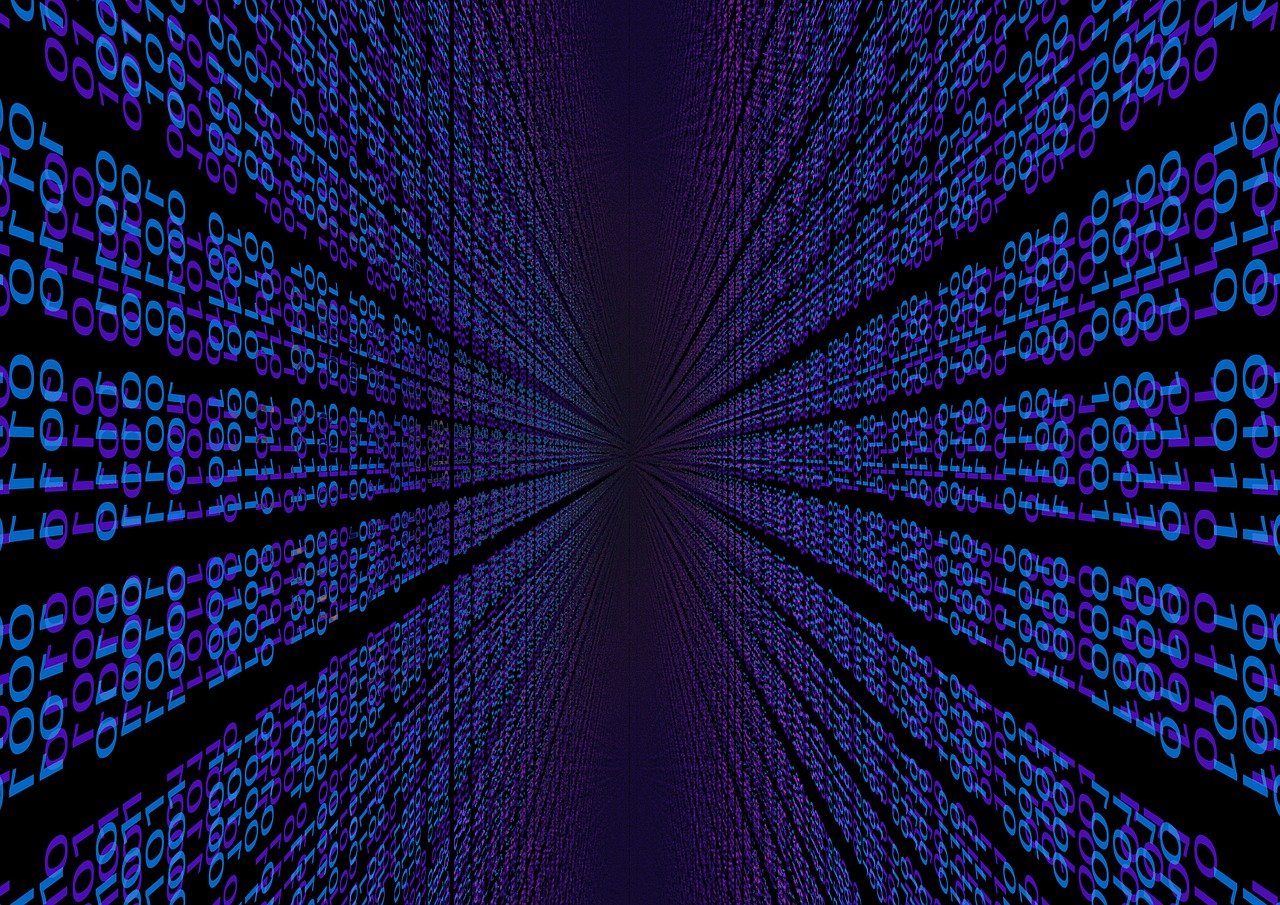
FeatureByte Inc., a startup focused on simplifying the artificial intelligence development task of feature engineering, today launched an automated feature recommendation product that it says can move AI projects along more quickly while cutting computing costs fivefold.
Feature engineering is the process of selecting, transforming or creating relevant input variables or attributes called features from raw data to improve the performance of a machine learning model. The Boston-based company launched last year with the goal of speeding up the preparation of data for machine learning applications and streamlining various ongoing management tasks such as documentation. It said its technology enables users to go from raw data to fully govern pipelines in minutes rather than weeks or months.
FeatureByte’s cloud service automates some of the most manually intensive aspects of feature engineering while improving feature engineering quality by optimizing feature selection for developers, the company said. That’s an improvement over the “brute force” approaches that are commonly used and that run the risk of creating too many possible features while diluting the model’s performance in a particular business context.
The company says data and analytics stacks are built primarily for business intelligence workflows, which makes them difficult for data scientists and data engineers to adapt to AI’s rapid experimentation, time travel, complex computations and historical accuracy needs.
FeatureByte Copilot provides customized feature recommendations for specific use cases, domains and inherent semantics of the data, with feature ideas based on relevance to the use case the company said. It gives data scientists and engineers a full view of the factors it uses to make decisions.
Recommended features are accompanied by a plain English description of why they were selected and how they apply to the use case. Features are automatically categorized and users can view, download and customize the Python scripts that underpin each feature.
All features are automatically categorized whether generated automatically or by users and FeatureByte’s integration with cloud data platforms and a built-in feature store enables automatic deployments of feature pipelines.
“The AI/ML lifecycle is complicated and time-consuming. Automated feature generation is a clear area that can impact model velocity, but adding context from domain-specific generative models provides even greater value, allowing data scientists a warm start, with the human in the loop, ultimately judging value,” said Kathy Lange, research director for AI software at International Data Corp. in a statement.
FeatureByte launched a software development kit in May and a self-service feature platform last month.
THANK YOU