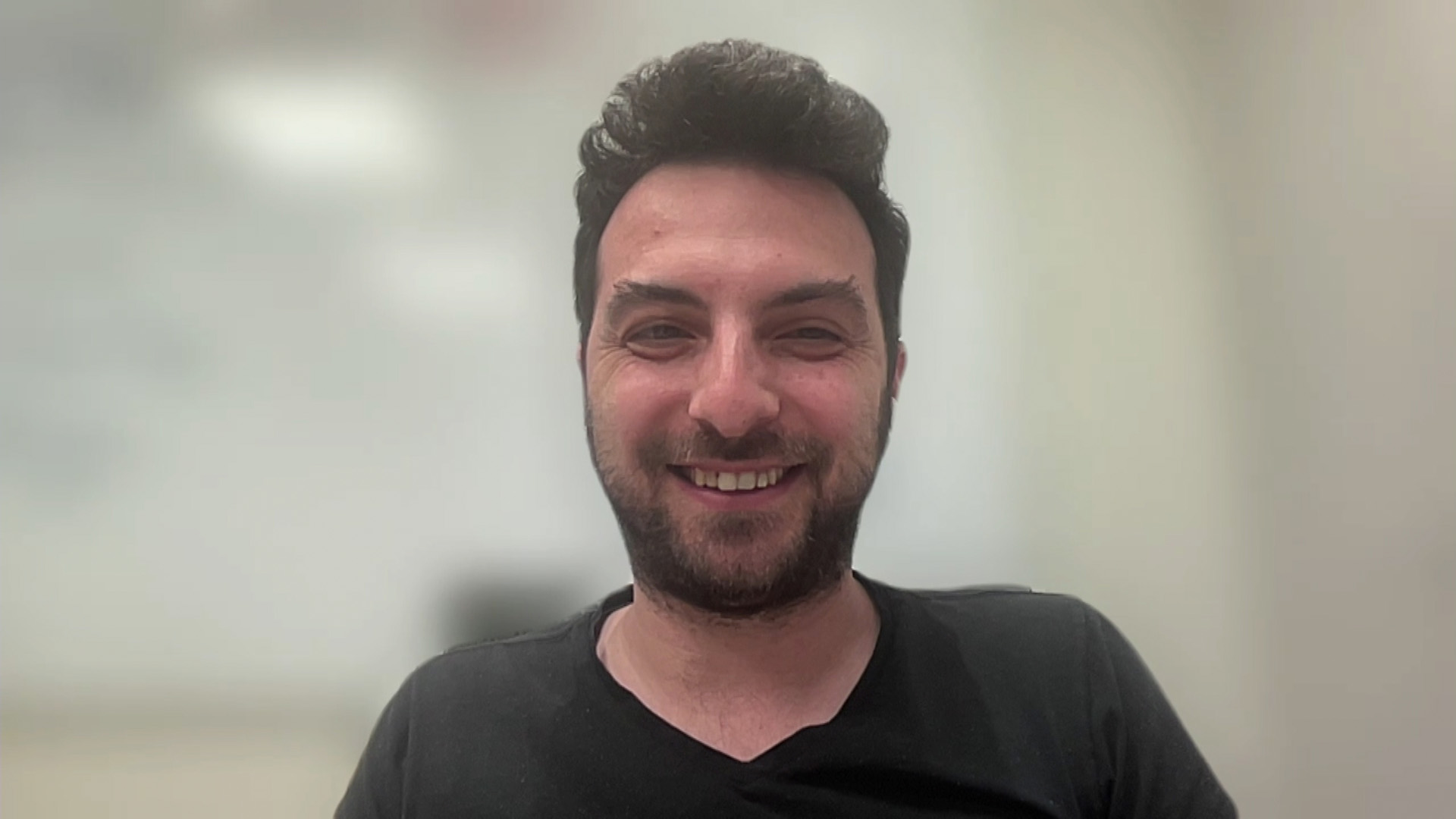
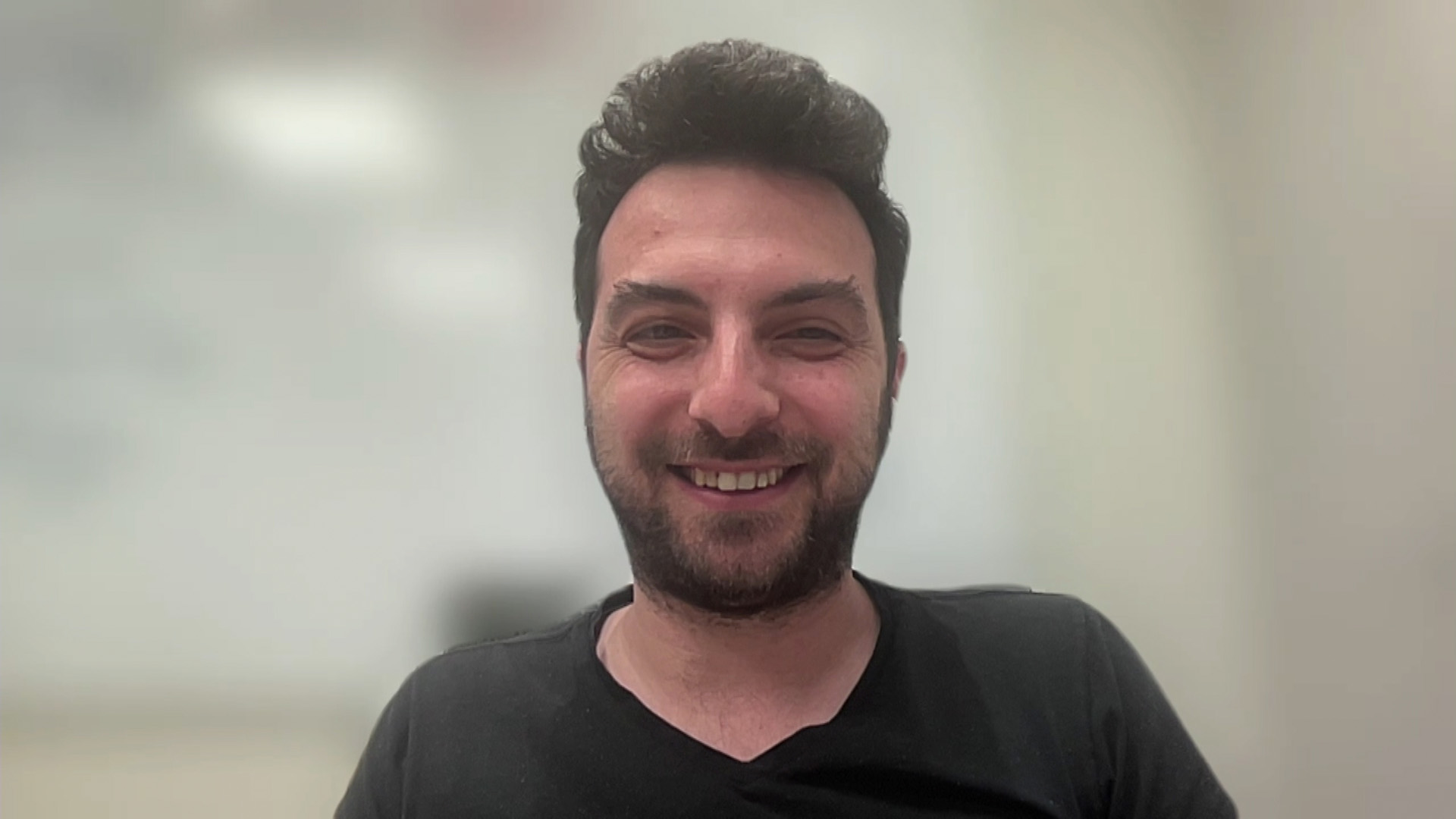
Artificial intelligence is moving so fast that six months feels like eons. In that time, things that seemed like “hype” around generative AI have manifested into reality and are showing promise for enterprise value.
“I think we’re still at the phase where most enterprises are still experimenting with the technology, doing some [proof of concept], testing to kind of understand the capacities and the limitations,” said Ori Goshen (pictured), co-founder and co-chief executive officer at AI21 Labs Ltd. “And [in] the next six months, we’ll probably see more and more production deployments, like gradually going to production and the implementing some of these use cases and testing them in a scaled kind of setup.”
Goshen spoke with theCUBE industry analyst John Furrier at Supercloud 4, during an exclusive broadcast on theCUBE, SiliconANGLE Media’s livestreaming studio. They discussed the emergence of a new wave in the tech industry, where the creative classes gain control and infrastructure/tooling evolves to meet the growing demand.
Six months ago, AI was in a phase of sporadic exploration, with businesses testing the waters to understand the capabilities and limitations of generative models, according to Goshen. Today, there has been a significant shift toward massive experimentation.
Every company and enterprise is now exploring how generative AI can impact their operations, even at the board level. This heightened attention and visibility have sparked immense interest in the field. It’s important to note, however, that many use-case scenarios from these wide-reaching experiments will be “flashy” but lack impact on enterprise bottom lines, according to Goshen.
“I’m speaking here very much intuitively — I think some of the use cases will be maybe flashy and really cool but not necessarily prove themselves in terms of [return on investment]. And others will be just dramatic ROI, more than people have expected or anticipated before,” he noted. “That’s the perspective I’m seeing from the last six months, kind of a year from now where we’re heading.”
Historically, emerging technologies have often dazzled with impressive demonstrations but struggled to scale. Thus, a real challenge in the gen AI space is transitioning from flashy demos to production-ready, scalable applications. Broken down, it shows in the areas of security and data governance, cost and reliability, according to Goshen.
“The aspects are the whole issue of security and how [to] manage data in this regime, where if you’re trying to customize these models, then some of that data is becoming available through these models actually can be extracted through this model,” he explained.
In relation to costs, scalability is the holy grail for enterprises exploring new value areas. What works well in a small demo may not be economically viable at scale. On reliability, gen AI models are inherently probabilistic, making mistakes and occasionally “hallucinating” information. Ensuring transparency, explainability and reliability in mission-critical environments is a complex challenge, Goshen added.
Open-source communities have played a vital role in accelerating research and progress in gen AI, thus promoting the development and democratization of the field. However, there still needs to be a nuanced delineation of the very concept of open source in the gen AI context.
“I think there are some organizations that take that path of full open source,” Goshen explained. “But the most common practice we’re seeing is actually that partial open source, where they just release the weights and the weights. You can think about these as binaries. It’s not like code — you cannot do a lot of things with it, but you can fine-tune it.”
Full open source, where both the model code and training data are released, is less common. In the future, there’s the general anticipation of a shift toward AI systems that encapsulate a portfolio of large language models. Open source will continue to play a significant role, enabling the proliferation of specialized models for various purposes, according to Goshen.
Additionally, there’ll be an architectural shift from relying on a single all-encompassing model to a combination of specialized models and a sophisticated orchestration layer. That layer will be responsible for managing the statistical nature of the models, minimizing errors and ensuring transparency and explainability. This architectural evolution could lead to a new operating model for AI, Goshen concluded.
Here’s the complete video interview, part of SiliconANGLE’s and theCUBE’s coverage of Supercloud 4:
THANK YOU