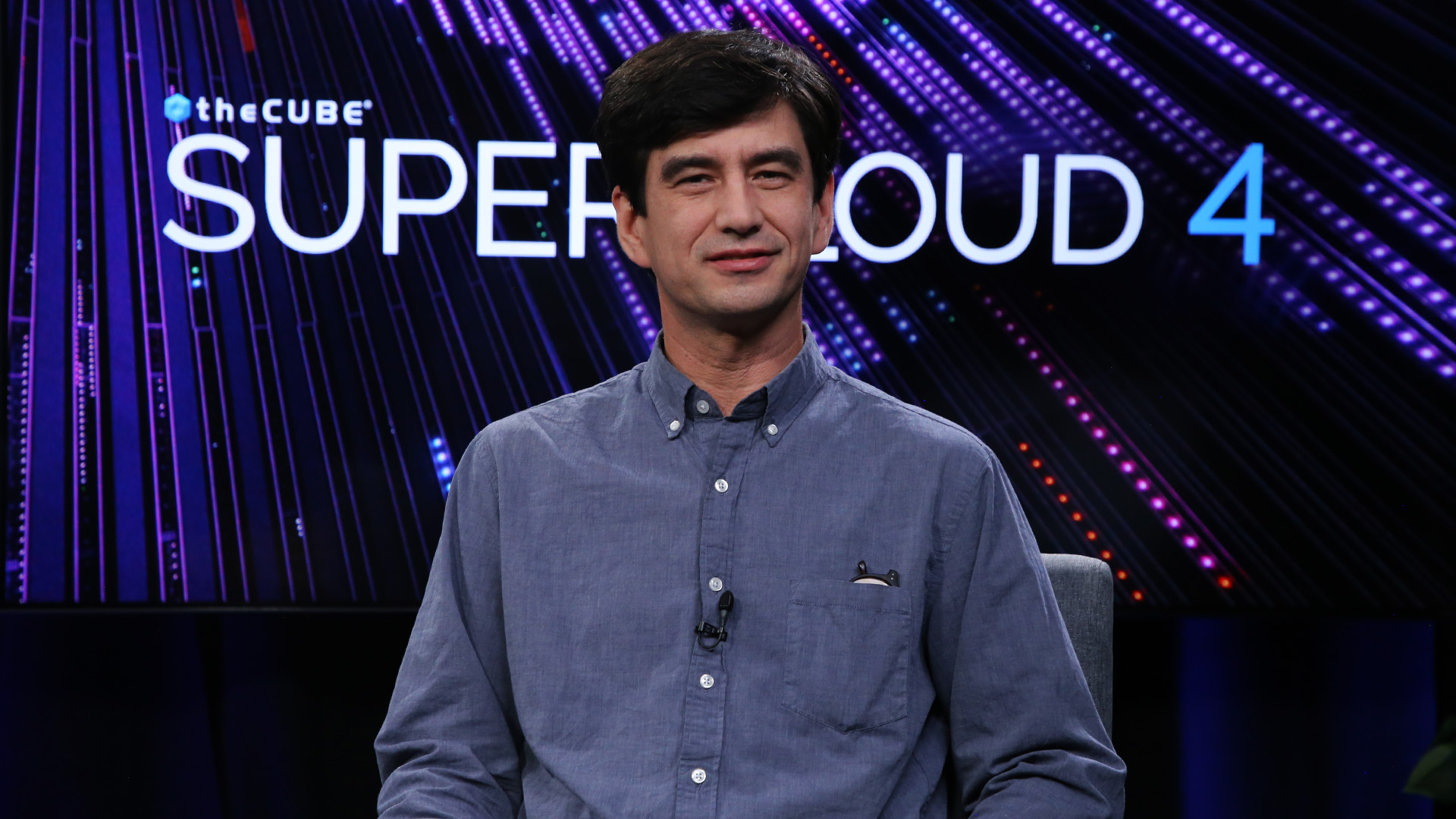
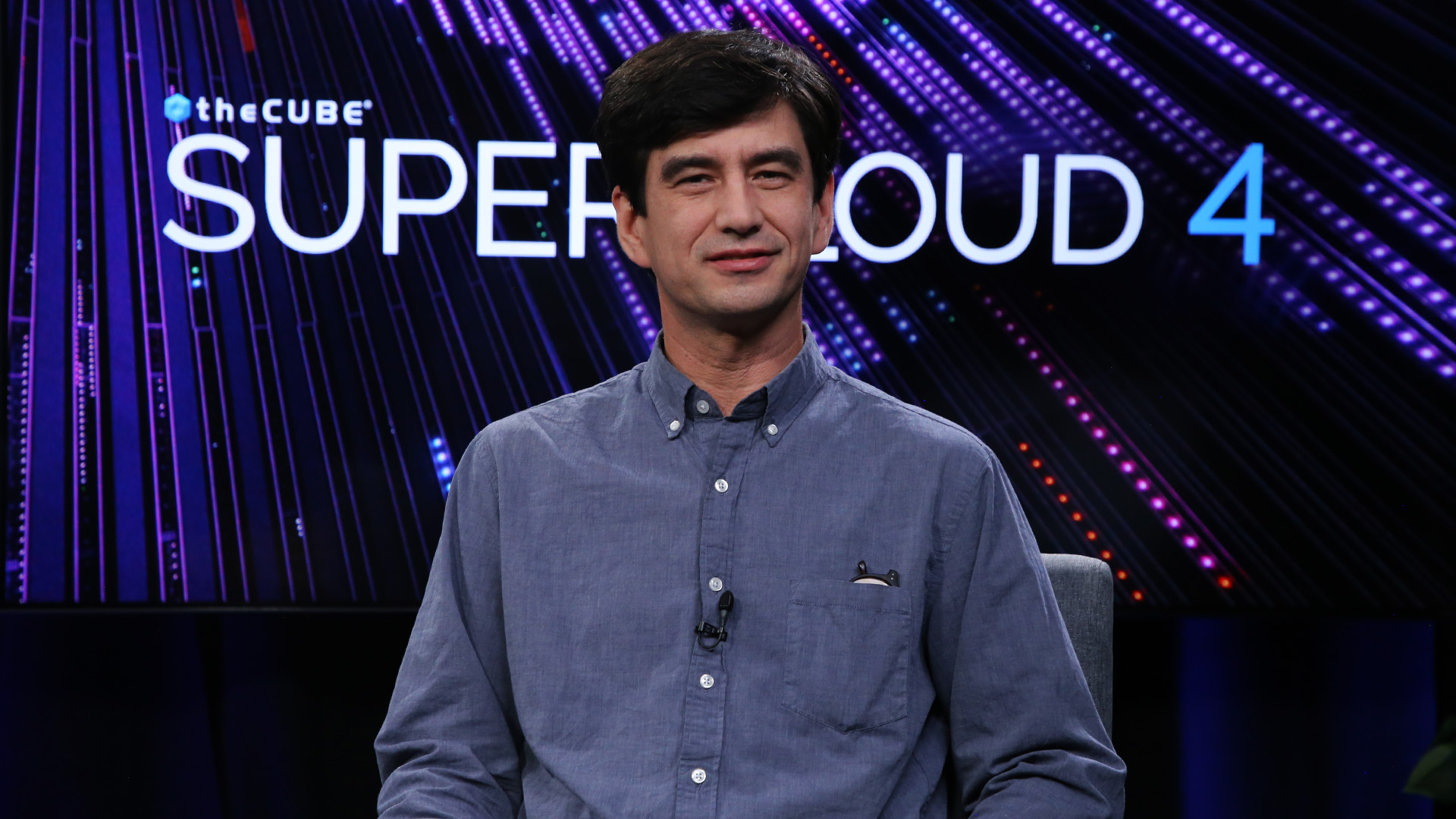
The data arms race in the artificial intelligence world is shaping the future of technology and innovation — and ensuring data quality, addressing model collapse and choosing the right infrastructure are all critical components of success in this race.
The democratization of AI and the availability of everyday, real-world data have brought AI into the mainstream. The challenges lie in bridging the gap between data scientists building models and the infrastructure required to support them.
“Their main goal right now is obviously to capture as much of the data as they’re generating as possible and keep it as long as they can,” said Andy Pernsteiner (pictured), chief technology officer of VAST Data Inc. “But also to curate and cleanse it so that it’s useful for training against.”
Pernsteiner spoke with theCUBE industry analyst Dave Vellante at Supercloud 4, during an exclusive broadcast on theCUBE, SiliconANGLE Media’s livestreaming studio. They discussed the critical issues surrounding data quality, specialized cloud service providers and the ever-expanding role of AI in our everyday lives.
In the fast-paced world of AI and deep learning, the demand for quality data has become paramount. The race for data isn’t just about quantity; it’s about ensuring that the data is reliable and free from biases, according to Pernsteiner.
“There’s ways that the people developing the models can work around it or fine tune against it, but it’s extra effort as opposed to training against real data,” he said. “If everything you’re getting is synthetic, then it’s challenging to know if that’s a real signal or a fake signal.”
One of the key challenges in this data arms race is the concept of “model collapse.” When synthetic data dominates, models tend to over-rotate on the highly probable, potentially ignoring the improbable. This imbalance can lead to biased and polluted models. To combat this issue, organizations are increasingly looking to acquire real, high-quality data to train AI models.
“To get data to a state where you can actually use it for training is very difficult,” Persteiner said. “There are technologies that can leverage GPUs to do data prep and ETL. When you’re in the state where it’s hard to get something and it costs a lot of money, you better be ready for it when you have it.”
The emergence of specialized cloud service providers is changing the landscape of AI infrastructure. Traditional cloud giants, focused on virtualization, may not provide the level of specialization required for AI and deep learning projects, according to Pernsteiner.
“Once you find that you’re having to scale the amount of data that you’re training and the number of GPUs and the orchestration required to keep all of that running in concert, that’s where you need somebody who’s more specialized in it,” he added.
These specialized CSPs offer turnkey solutions for enterprises and commercial entities, eliminating the need to build complex infrastructure from scratch. They understand the unique requirements of AI workloads, including the high-performance and IO-intensive nature of deep learning. This specialization allows an organization to make the most of its GPU resources without the hassle of data preparation and orchestration, Pernsteiner continued.
“I was recently speaking in London at a talk, and it’s all data scientists, data engineers and people who are in theory involved in AI deep learning and machine learning,” he said. “There’s this big disconnect between people who are practitioning and using the infrastructure.”
While some technologies such as Delta AI, Iceberg and Hudi AI focus on analytics and structured data, many AI and deep learning projects rely on unstructured data. It is important that a platform that can handle both structured and unstructured data efficiently, eliminating the need for additional data transformations, according to Pernsteiner.
“Those are very heavily focused on analytics, which means the data has to be structured to get into it in the first place,” he said. “Whereas a lot of the LLM-based sort of deep learning projects and, of course, all the multimodal projects are focused on unstructured data to start with. As customers start to learn more and more about how to actually do unstructured deep learning, they’re going to need a platform that can handle both.”
As the AI landscape continues to evolve, organizations will need to navigate these challenges and leverage specialized cloud service providers to stay competitive in the data arms race. The future of AI depends on the ability to harness vast amounts of data effectively and efficiently while maintaining data quality and integrity.
Here’s the complete video interview, part of SiliconANGLE’s and theCUBE’s coverage of Supercloud 4:
THANK YOU