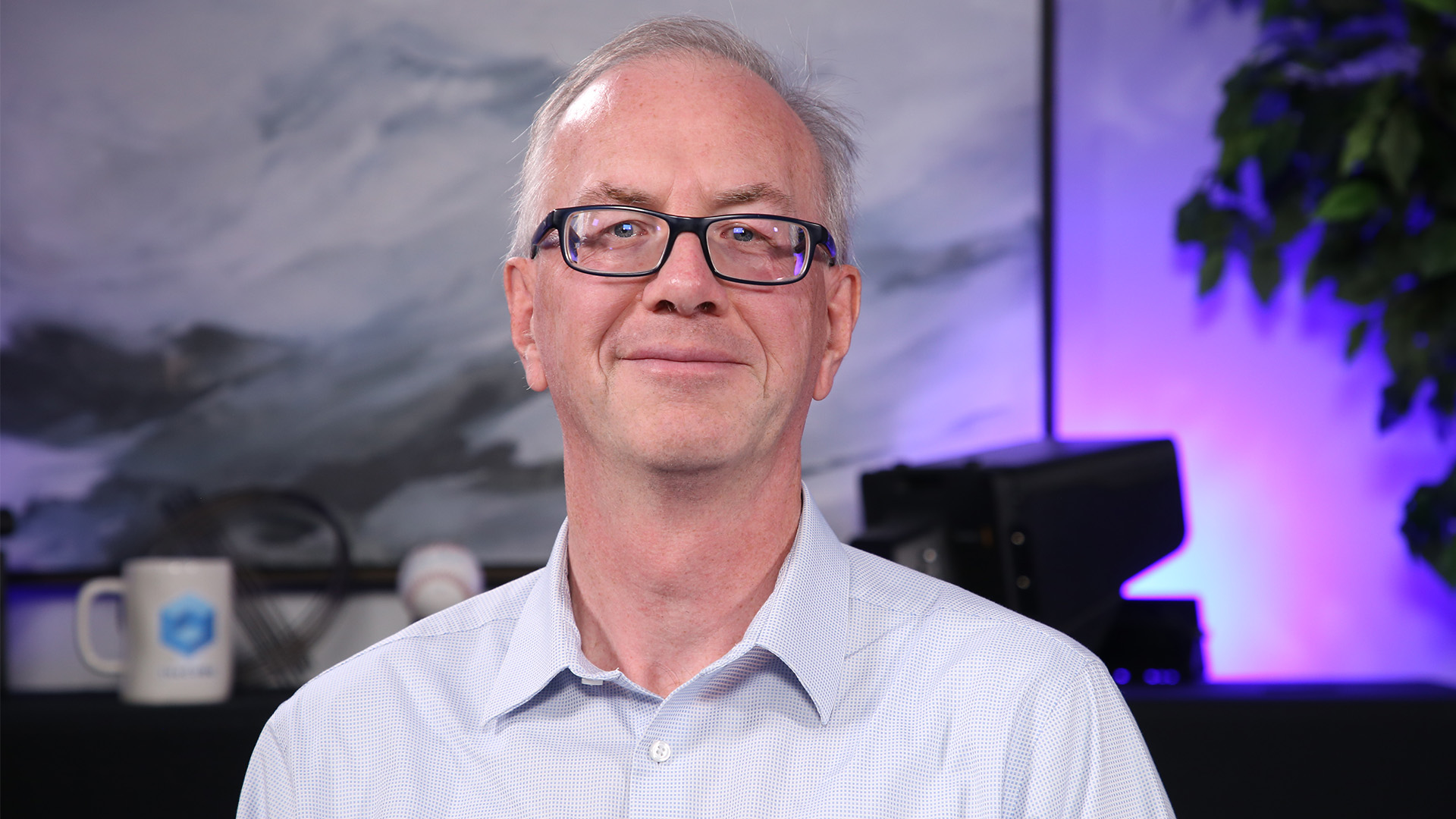
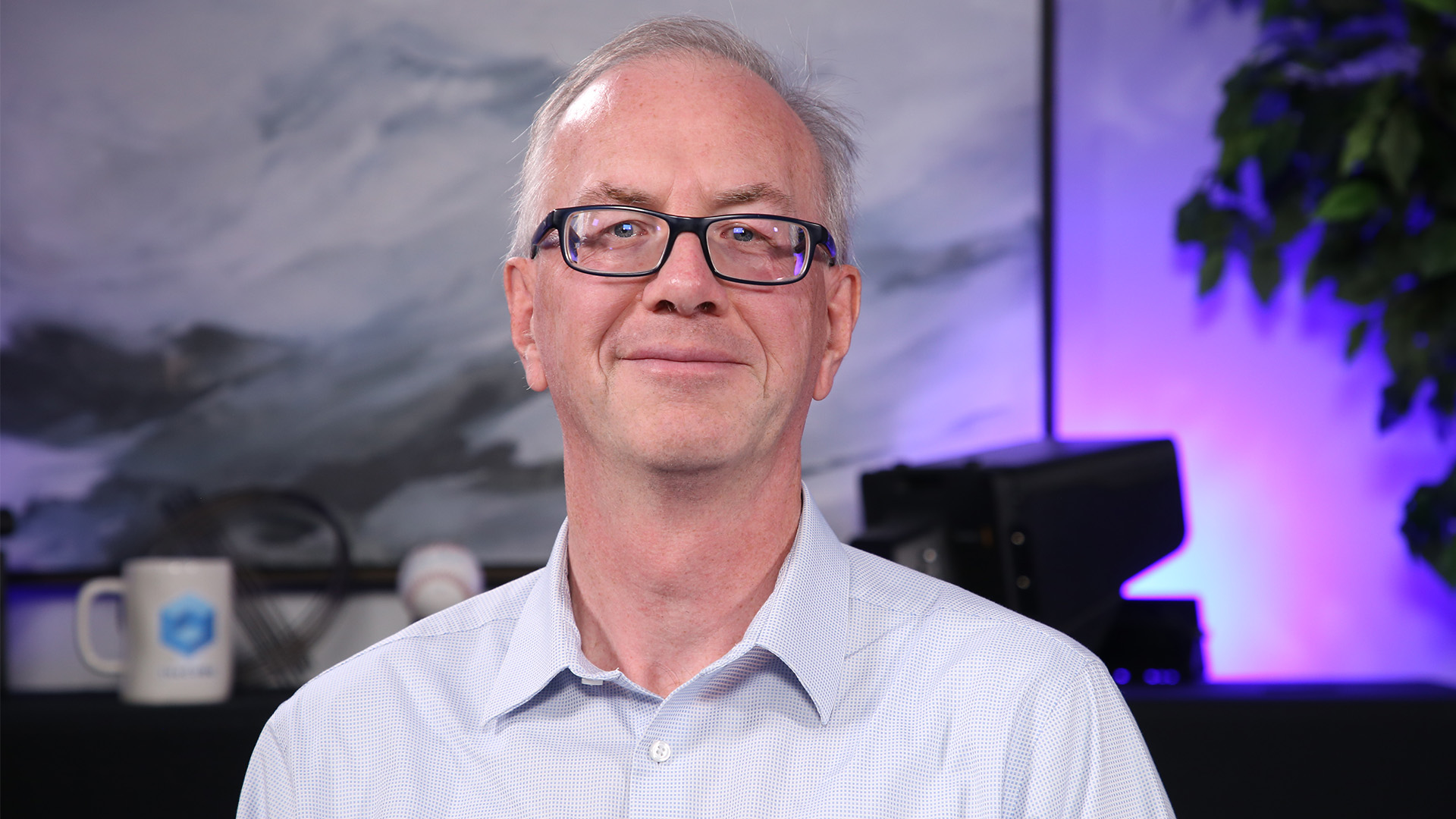
With artificial intelligence becoming a commodity as enterprises realize they have to incorporate this cutting-edge technology, compatibility is of the essence.
And that’s true from the cultural to the hardware approach, as well as the methodologies adopted, according to Frederic Van Haren (pictured), founder and chief technology officer of HighFens Inc.
“One big thing we see is corporate IT people or organizations kind of doing the same thing as they always used to do,” Van Haren explained. “Somebody might say, ‘Well, I understand I need to buy a GPU, but I have an old server lying around. I’m going to put that GPU in my old server and then I’m going to do some AI.’ They quickly realize that it’s not compatible and that there’s a lot more going on. Every organization kind of reinvents the wheel if you wish. Maybe a better way to say it is that a lot of organizations try to reinvent the wheel.”
Van Haren spoke with theCUBE industry analyst Rob Strechay at Supercloud 4, during an exclusive broadcast on theCUBE, SiliconANGLE Media’s livestreaming studio. They discussed why AI is taking the enterprise world by storm and what it takes to be successful in this field.
For maximal ROI, splitting AI into both on-prem and cloud environments is of the essence, according to Van Haren. This approach is needed when dealing with both training and inference parts for cost, flexibility and security reasons.
“We have to split the AI question in two pieces,” he stated. “One is training and inference. Training is really where all your data sits, and typically, depending on the organization, they might decide it on-premises for security reasons or maybe cost reasons. The public cloud is great, but something 24/7 can turn out to be really costly. If you think about the inference of the production side, that’s where I see the public cloud really shining because of the flexibility and only pay for what you use concept is really key there.”
For businesses to run fast, they need to demystify AI, according to Van Haren. This entails organizations understanding what AI can and cannot do for them, as well as comprehending the algorithms, mathematics and data behind the scenes.
“Everybody wants to get up and running as fast as possible,” he said. “The reality is really about basic AI, demystifying AI, the concepts behind it … it’s not voodoo. Explaining there’s data behind it, there is math behind it, there is scale behind it. Why do we need GPUs? What’s happening to the market?”
AI has been around for quite some time. One thing that has changed is the trend has become more data-centric. For instance, data has to be at the epicenter when approaching an AI problem, as well as when deploying the hardware and models, according to Van Haren.
“We always talk about AI as something new,” he pointed out. “The reality is AI has been around for a long time, just like HPC, and I think the big trends in the early 2000s is that we went from a software-centric or code-centric approach to a data-centric approach. Nowadays, it’s data, and that’s a complete shift.”
Given that data continues to be the lifeblood of enterprises, it is stamping its authority in the AI field. As a result, managing data is important because it determines whether it will be a make-or-break affair when it comes to deploying AI models, Van Haren pointed out.
“If you look at the more advanced AI organizations, they don’t have a lack of data,” he said. “The challenge with too much data is you don’t know the value of the data unless you process that data, and processing data comes at a decent cost. I mean buying GPUs, they’re not cheap. In the end, data management becomes your key focus, at least for the organizations that are relatively mature.”
Not only is the quality of the data important, but also the data lineage and the expected business value. This is because AI has emerged as a time-to-market concept, and understanding the data used is vital for maximal value, according to Van Haren.
“Data lineage comes up a lot and it comes up from different angles,” he said. “One of them is bias. If you have a model, you kind of want to understand what data went in there. But at the same time, you want to use data lineage to figure out if the data you used, you were able to extract the value you wanted, and if not, maybe it’s worthwhile to look at different types of data.”
Here’s the complete video interview, part of SiliconANGLE’s and theCUBE’s coverage of Supercloud 4:
THANK YOU