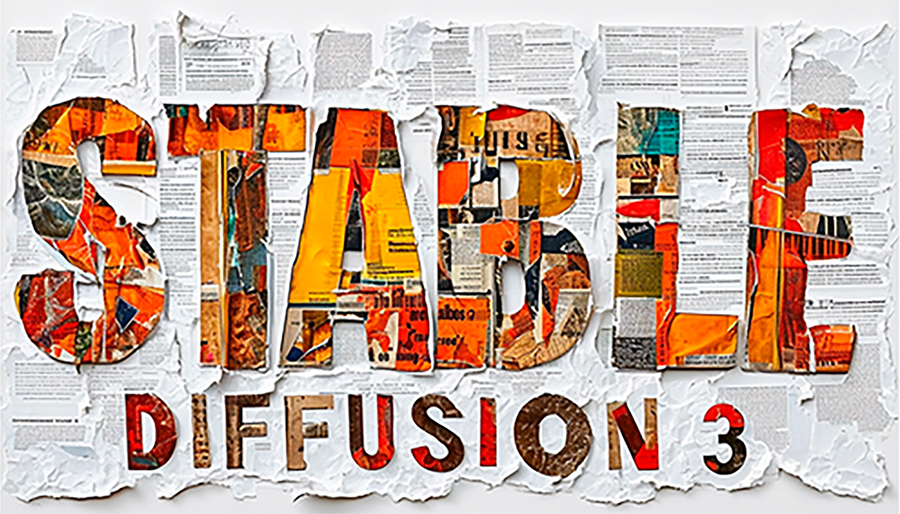
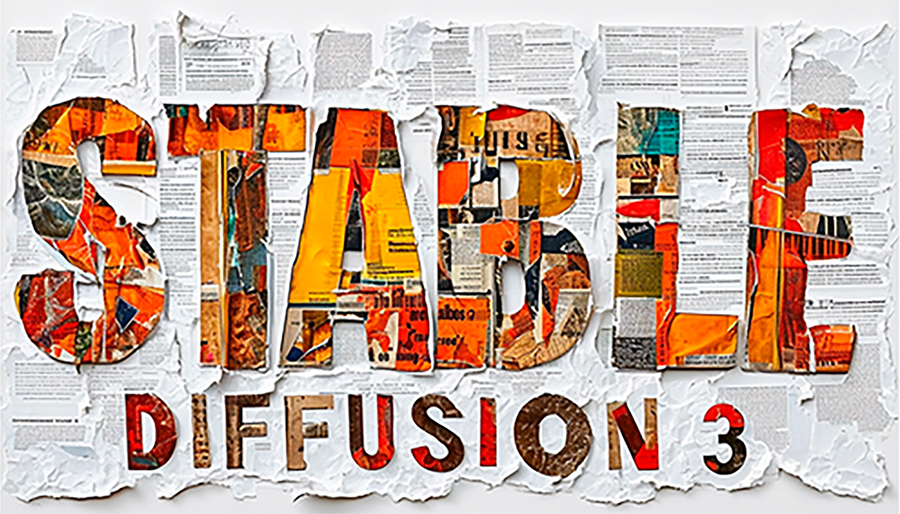
Open generative artificial intelligence startup Stability AI Ltd. today announced the early preview of Stable Diffusion 3, the next generation of its highly advanced text-to-image AI model.
The new model makes a large number of improvements over its previous model, including improved performance, image quality and prompting capability, the company said.
In particular, Stability focused on increasing the model’s ability to accurately generate words and spell better in produced images. One thing that many users of image-generating AI models discover when working with them is that they sometimes create gibberish when asked to make scenes with words in them.
Stable Diffusion 3 comes in a variety of model sizes ranging from 800 million to 8 billion adjustable variables, called parameters, which allows them to be fine-tuned by developers and researchers to produce the images they want. The different sizes, or weights, represent more capable and complex models that can produce more vivid and equally complex scenes, but the larger models also require larger compute infrastructure to fine-tune and deploy.
“This approach aims to align with our core values and democratize access, providing users with a variety of options for scalability and quality to best meet their creative needs,” the company said. As the models are open-source, researchers and developers have direct access to the underlying architecture and code of the models to work with as they see fit.
The new models build on a new backbone that uses diffusion transformer design, which explores a new class of diffusion model architecture. Transformers are the traditional basis for image generators, but they have been developed using a U-Net backbone. They’re so named because the backbone resembles a U-shaped encoder-decoder architecture that segments an image into compressed form and then decodes it to reconstruct it into its original form.
The new model replaces the U-Net with a diffusion transformer that breaks the image up into patches. Researchers discovered that can scale up exceptionally well.
Stability said it will combine this new architecture with “flow matching,” a process described in a research paper as a new method for generative modeling built on “continuous normalizing flows” that allow for training models based on probability paths. Using flow matching, generative models can be trained more quickly. It allows them to generalize more easily by providing the model with the most optimal paths it can take when learning from unstructured data, especially a wide variety of images.
Since the model is still in early preview, Stability said it’s introducing a number of safeguards to prevent its misuse and it will collaborate with researchers, experts and its community to build best practices in AI safety as the release date approaches.
A waitlist is available for developers and researchers interested in testing out the model when it’s released.
THANK YOU