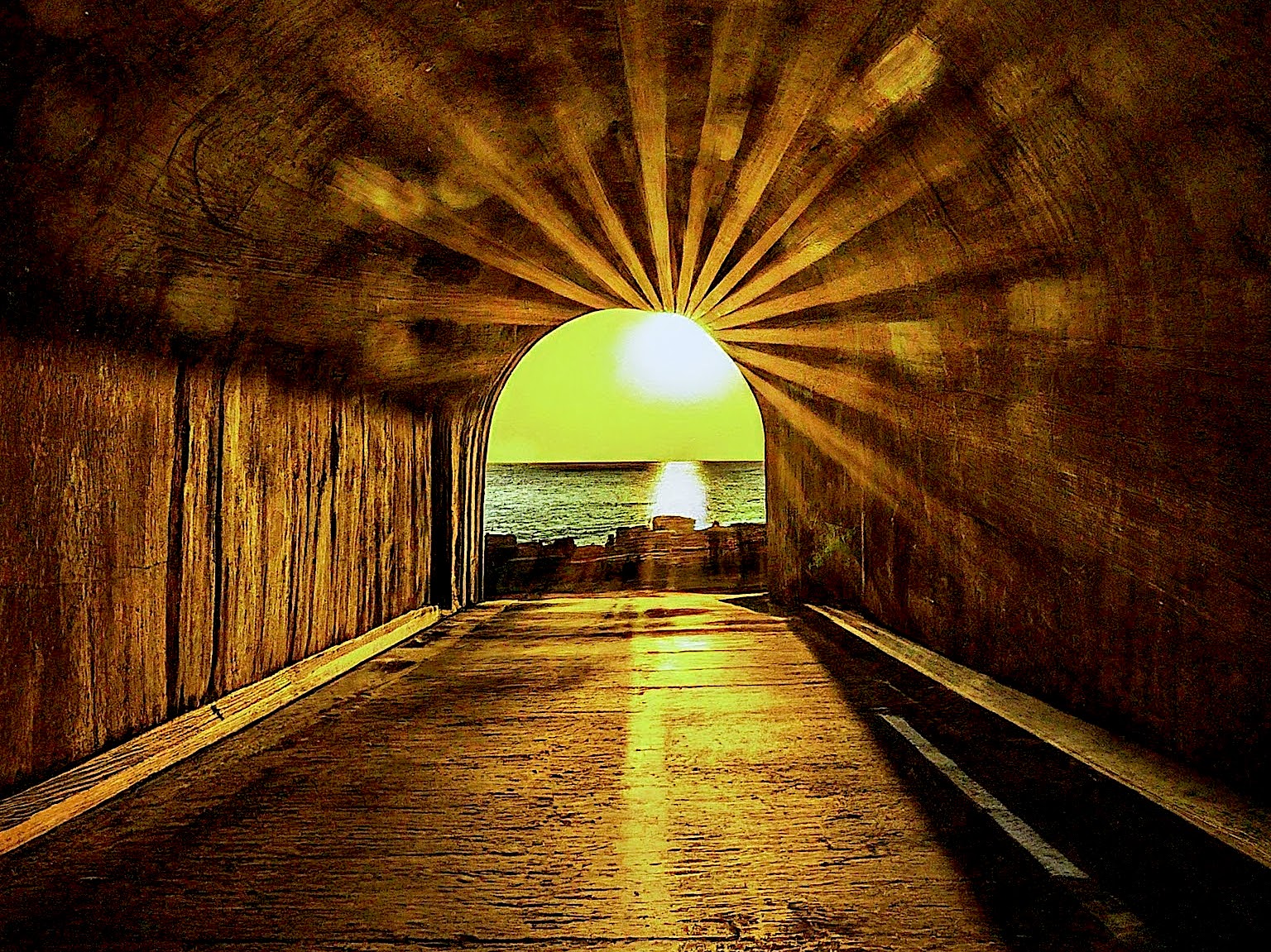
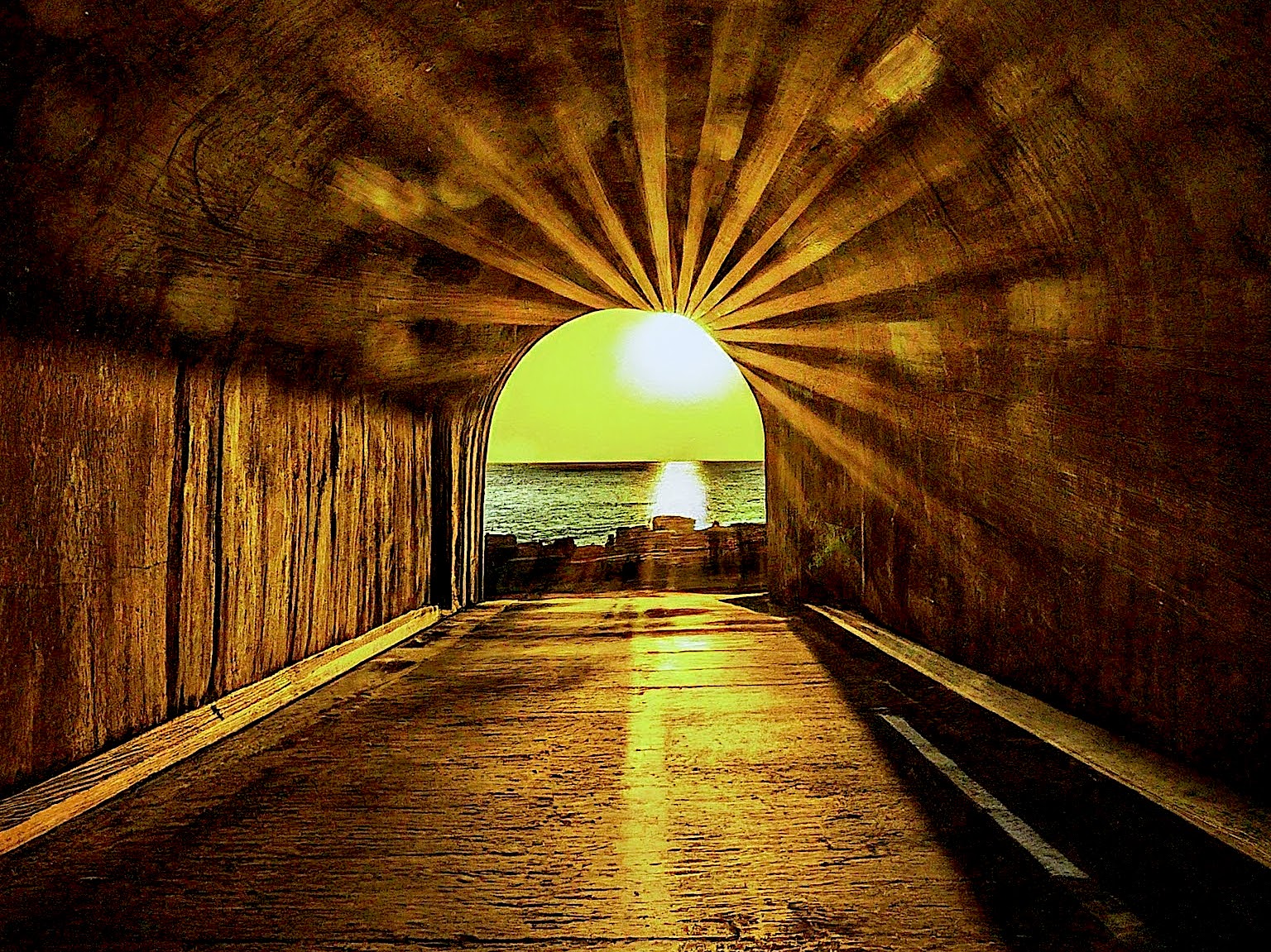
This is the second two parts. You can read part one here.
Yesterday, we rained on the generative artificial intelligence parade. We stated that that today, gen AI is currently a cost sink, with the true promise not coming until at least 12 to 24 months off. Today it’s time to clear the clouds.
We’ll start with “The Wizard of Oz” metaphor that we promised yesterday. It plays out in several ways.
The first metaphor is The Yellow Brick Road. It will be a long road that we’ll have to follow before we finally find the award somewhere over the rainbow. That is, if we can get past the smoke and mirrors first.
The second Wizard of Oz metaphor is the Kansas tornado that swept up Dorothy, which served as the set up for the classic 1939 film. Updating the script to 2024, it’s the sound and fury of that tornado drawing up ridiculous hordes of capital investment. That prompts us to mix metaphors for a moment, back to that sucking sound we spoke of yesterday. The gen AI tornado that has descended on the industry is sucking up much if not most of the available capital, and it’s forcing tech and solution providers to drastically re-budget and reorganize to marshal the resources for what is going to be a very expensive upfront investment.
Now what about those rewards somewhere over the rainbow? Excluding classic bleeding-edge early adopters, for at least the next 12 to 18 months, most enterprises are going to be kicking the tires with proofs of concept. Yes, there will be quick hits with natural language query, smart bots and coding copilots. But beyond that, a number of pieces must come together.
Enterprises need to identify the use cases and figure out how to address governance, bias and intellectual property issues. We won’t dwell heavily on governance here, but the problem is far more opaque and the stakes (especially with deep fakes and copyrights) far broader and deeper than what we’ve been talking about with “classic” machine learning models. Meanwhile, the industry needs to put together the ecosystem and guardrails.
So, if in the short run gen AI is a classic loss leader, where’s the good news in all this?
The industry appears poised to make the investment. Looking at their recent financial statements, the Magnificent Seven are doing just fine, thank you, with their stocks outpacing the rest of the S&P 500 for almost the last 15 months. And they are collectively sitting on a combined pile of cash exceeding $180 billion. The resources are there to dive in.
We focused yesterday on the hardware, where Nvidia Corp. is currently rationing product. The flip side is getting smarter with the silicon we have, or can afford. There’s light at the end of the tunnel here: With time, solution providers will get smarter at cutting down training costs with expertise on how not to go overboard with training. The future, as we’ll note below, is trending away from the 40 billion-parameter models to those that should do just fine with about a 10th of that number.
Nonetheless, the industry is undergoing major disruption. Look closer, and you’ll see furious repositioning: Amazon.com Inc., Alphabet Inc., Meta Platforms Inc., Microsoft Corp., and Tesla Inc. with layoffs in the tens of thousands, leaving only Nvidia unscathed. At first glance, one would think that these firms are preparing for a recession, but excluding Tesla, most are forecasting 10% to 15% growth this year. Admittedly, some of this might be a readjustment from overhiring during the pandemic, but much of this is being driven by the need to mobilize for gen AI, because of the capital and talent needs.
Amazon took cuts in video and streaming; Facebook retrenched on the metaverse; Microsoft cut back on gaming; while Alphabet’s cuts spanned across hardware, ad sales, search, YouTube and other areas. On our end, we’ve seen vendors gutting their marketing budgets.
But what goes down must come up. Since the release of ChatGPT just over a year ago, demand for gen AI-related skills has soared over 18 times according to Lightcast, which tracks employment trends (figure below). That of course refers to demand for data scientists and ML/AI engineers, but it also includes curriculum writers. Surprisingly, prompt engineering was not ranked highly on the list, although there are questions whether that is part of what data scientists or AI developers do.
Although no figures are currently available for the degree to which the tech industry is recruiting, it would be a smart bet that for all the video producers and marketers being made redundant, there’s plenty of effort for re-orging and staffing up for gen AI, such as Google’s merging last year of Google Brain and Deep Mind units. If you want to know who’s hiring, here’s the tip of the iceberg.
Source: Lightcast
We never thought we’d write something like this: The cost and carbon footprint for training big gen AI foundation models could make cryptocurrency mining look almost trivial. Sajjad Moazeni, a University of Washington assistant professor of electrical and computer engineering, estimates that the power for hundreds of millions of queries processed on ChatGPT each day is equivalent to that consumed by 33,000 U.S. households.
As for training the next-generation GPT-4 model, OpenAI CEO Sam Altman was quoted at an MIT conference that the cost exceeded $100 million. At that same MIT event, Altman stated that the era of big models is over, but that’s not stopping OpenAI from developing GPT-5, which is supposed to be, literally, bigger and better.
As we hinted, there’s good news when it comes to sustainability, sanity and, most importantly, the learning curve.
Mainstream enterprises are not OpenAI, Anthropic or Cohere. They are not in the business of writing foundation models, and they’re not burning venture capital cash to train them. They shouldn’t need access to tens of thousands of graphics processing units.
And the work cut out for enterprises should be much more contained than that for the gen AI usual suspects. When training models specific to their own business problems, enterprises could conceivably leverage the work done by the OpenAIs, the Anthropics and all. But to prevent hallucinations and stay focused on their own problems and their ontologies and terminologies, it will be smarter not to train the models they implement on the whole of the internet.
Instead, they will likely restrict training data to their own sets and, optionally, relevant third-party real or synthetic sets from data marketplaces, and keep them updated and relevant with retrieval-augmented generation, or RAG. When it comes to language models trained specifically for the business, the meek (smaller domain-specific models) are more likely to inherit the earth. We’re talking about training models on millions rather than billions of parameters.
And most enterprises won’t be writing their own models from scratch, but instead they’ll take advantage of a growing ecosystem of open source and commercial third-party foundation models that are tuned for their own domains.
Here’s how it could play out. A company finds candidate models for their vertical industry or problem type or both from a marketplace and runs some A/B tests. Once the model is selected, it would be pointed a finite corpus of data. For instance, a language model utilized for summarizing transactions, texts, emails and contracts for compliance violations could target data that is wholly contained within the boundaries of the enterprise and in some cases might be supplemented with selected sets from data marketplaces.
That data would likely be stored as vector embeddings and made accessible through specialized vector indexes that reside in specialized vector databases, or the vector data stores of the operational databases that they are already using. In turn, language patterns and terminology would be trained on much more finite vocabularies and ontologies.
Even if the supply side of GPUs loosens up over the next few years, our take is that best practices with smaller language models, or SLMs, will be the first development that starts bringing sanity to language model compute costs. But that’s not going to happen overnight. It will likely be at least a couple years before we see a critical mass of domain-specific models and best practices for sizing them for us to see significant impact on compute costs. But it will happen.
In some ways, the commercialization of gen AI may follow a similar path with machine learning before it. We’ll see the budding of marketplaces for third-party commercial and open source foundation models, many of which will be domain-specific, that will become available from the hyperscalers and enterprise technology usual suspects.
Amazon Bedrock, Google Cloud Model Garden and IBM watsonx.ai, which each already boast selections of dozens of models, are good examples of what we’re likely to see more of. And these models are bound to be far more compact than the GPTs, Claudes or Geminis of the world.
But perhaps the most impactful development will be gen AI models that hide in plain sight. It will be embedded into horizontal services such as document summarization, BI and visualization tools, and the enterprise applications that we already know from household names such as Adobe Inc., Salesforce Inc., Zoom Video Communications Inc. and so on. Microsoft Copilot for Office 365 is arguably the most prominent poster child, while SAP Joule typifies the types of task-specific copilots (this one for staffing optimization) that we’ll be seeing cropping up in enterprise applications. That’s akin to how we saw machine learning emerging behind the scenes powering the predictive analytics in enterprise apps over the years such as Oracle Fusion Analytics.
The common thread is that it won’t require enterprises go down into the weeds to train models as these are delivered as prepackaged software-as-a-service applications that are priced at the costs that enterprises will bear. Like machine learning before it, for most enterprises gen AI will surface as applications, not raw models.
Tony Baer is principal at dbInsight LLC, which provides an independent view on the database and analytics technology ecosystem. Baer is an industry expert in extending data management practices, governance and advanced analytics to address the desire of enterprises to generate meaningful value from data-driven transformation. He wrote this article for SiliconANGLE.
THANK YOU