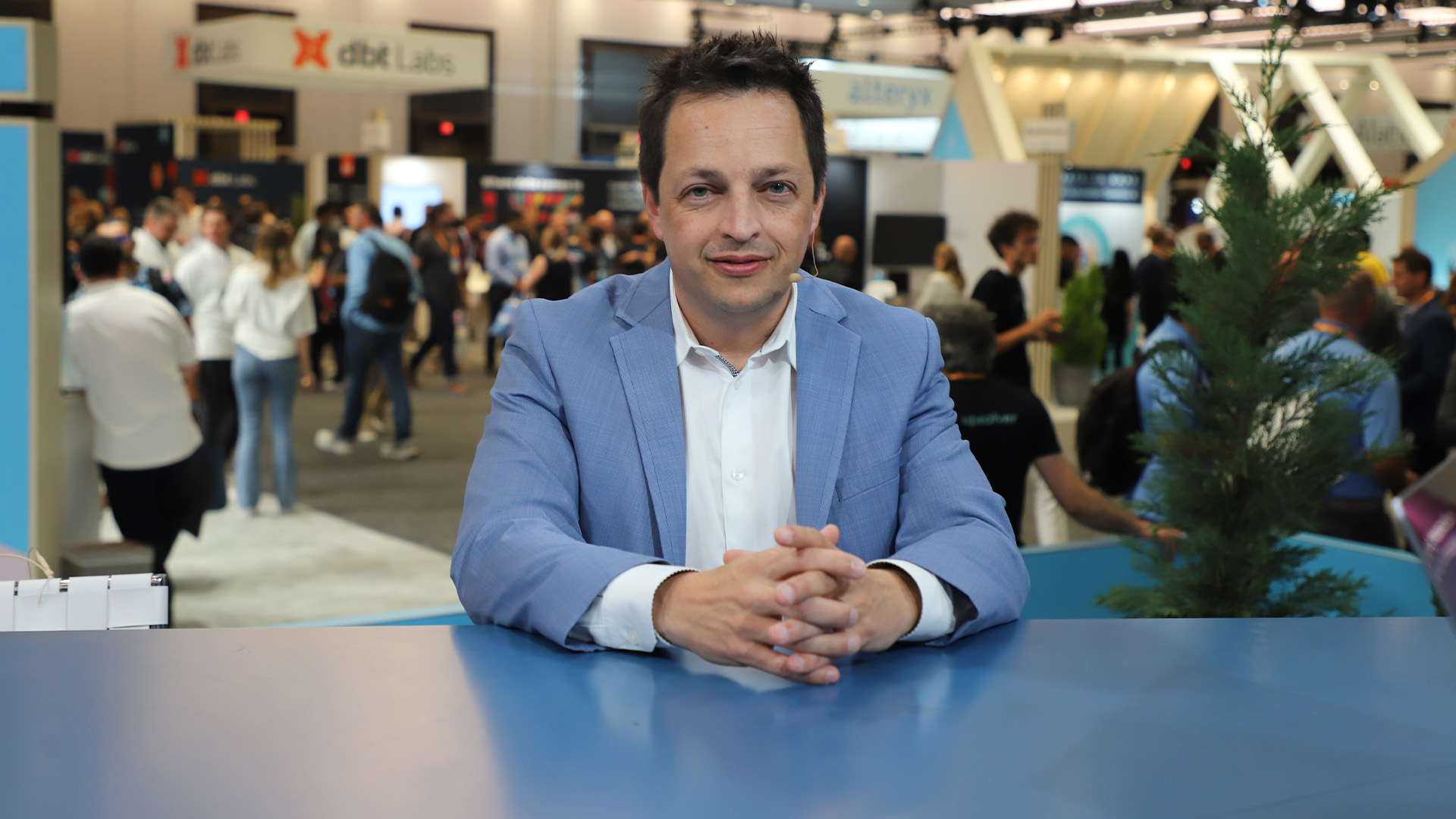
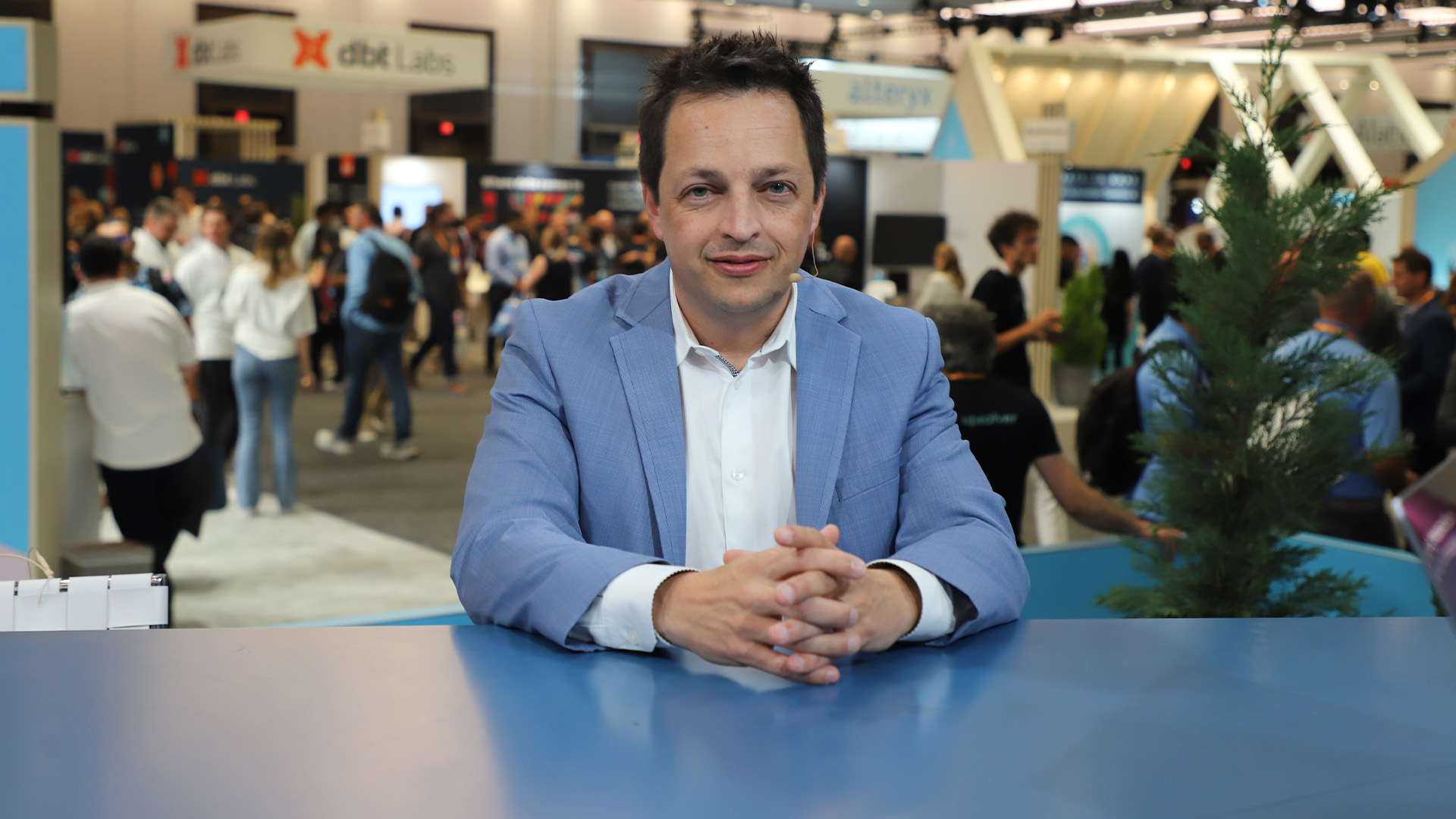
Leveraging its unique perspective on how customers manage data on its platform, Snowflake Inc. today released a report on the changes it’s seen over the past year in data and tools usage as artificial intelligence has grabbed its customers’ attention.
The analysis of more than 9,000 Snowflake accounts found that AI use cases now dominate, with usage of the Python language, tags, unstructured data and purpose-built AI development tools all showing double- and triple-digit growth.
Python is now the overwhelming favorite language for AI and analytics development, with usage growing by 571% over the past 12 months. Close behind is Scala, with 387% year-over-year growth. Java, which dominated a year ago, grew a respectable 131% but has been eclipsed by Python. “Growth in Python is coming at the expense of Java,” said Christian Kleinerman (pictured), executive vice president of product at Snowflake.
Python’s virtues include ease of use, the availability of a large ecosystem of libraries and frameworks, portability and powerful data-handling capabilities.
“We can now see Python is becoming the de facto standard,” Kleinerman said. “Every organization now leads with Python [application program interfaces].”
Further evidence that organizations are ramping up their AI initiatives is the growth in the use of unstructured data like free-form documents and images, which is up 123% year-over-year. One of the most popular uses of AI is extracting insights from large corpora of text or multimedia that aren’t easily handled by conventional business intelligence tools.
“We see customers accessing documents from Python and storing them in structured tables,” Kleinerman said. A capability currently in private preview called Document AI that’s meant to simplify that process, “is completely oversubscribed,” he said. “I have a waiting list of 400-plus customers because you can just point Snowflake to a repository of documents and start asking questions in natural language.”
The use of tags, or metadata that describes and governs the use of data in AI models, has also increased significantly over the past year. The number of tags applied to an average individual object rose 72%, and the number of objects with a directly assigned tag is up almost 80%. The use of tag-based masking to control row-level access surged by 98%, and the cumulative number of queries run against policy-protected objects was up 142%.
Kleinerman said tags are becoming an important element of security policies. They allow users to restrict access at a granular level without locking down entire tables.
“An even more interesting connection between tags and AI governance is that they can improve models’ results by helping them understand questions better,” he said. “Tags provide semantic annotations and semantic understanding of data.”
These tools are used by many people, not just data scientists. Between the introduction of Snowflake Cortex last fall and the end of January, the number of active accounts using machine learning functions grew by two-thirds. Cortex is a managed service that enables non-technical users to analyze data and build AI applications on the Snowflake platform. Over the past six months, monthly usage of Cortex grew 90%.
Cortex enhancements, which will be announced in June, aim to make it easier to customize models through retrieval-automated generation, a natural language processing technique that embellishes pre-trained language models with external knowledge sources to generate more contextually relevant responses.
“Today, you can get the pieces like the vector type and the embedding,” Kleinerman said. “What’s coming in the next three months makes it more turnkey so you can point Snowflake at a set of files or tables and just start asking questions.”
There is unquestionable demand for such a feature, given that a Snowflake survey of 980 Streamlit users found that accuracy was far and away their top worry about LLMs.
Much of the development work customers are doing has shifted from query-like single-text input applications to iterative chatbots. A year ago, 82% of LLM applications built with Streamlit used single-text input; at the end of January, that number had fallen to 54%, while chatbots had grown from 18% of projects to 46%. One of the appeals of chatbots is that they allow models to be refined, whereas query applications are static.
Similar usage growth was evident in Streamlit, an open-source Python library that allows users to create interactive apps from data and machine-learning models. Two years ago, Snowflake made a big bet by acquiring Streamlit Inc., and it appears to be paying off. Streamlit developers are today working on more than 33,000 large language model-based applications, with 65% planned for production use.
However, usage is largely internal today due to concerns about accuracy and hallucinations. “The most common use case is support-related, such as call center,” Kleinerman said. “When I talk to asset managers, they said it will be a long time until they trust a bot to provide answers [directly to customers] because there are lawsuits if they’re wrong.”
THANK YOU