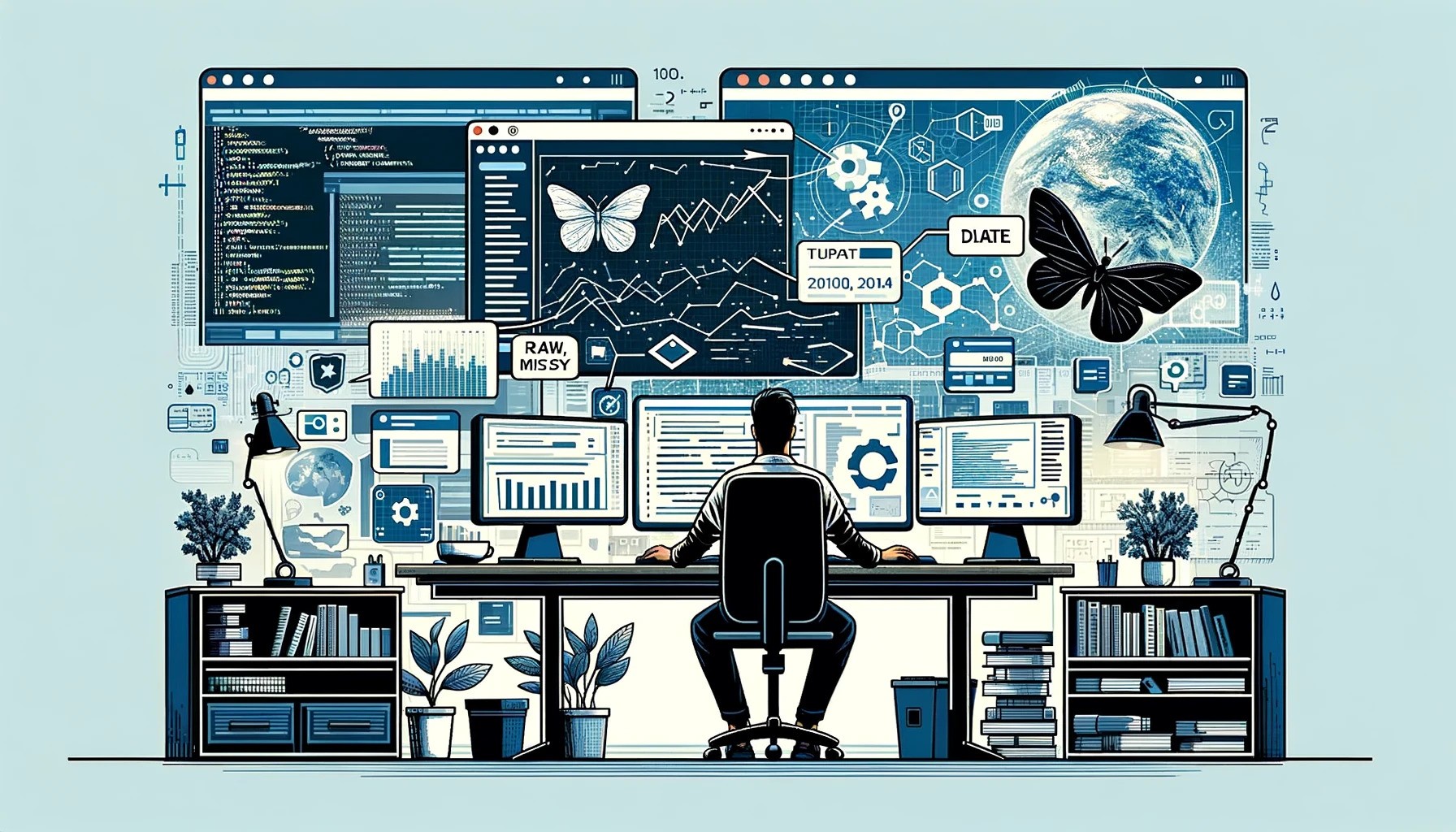
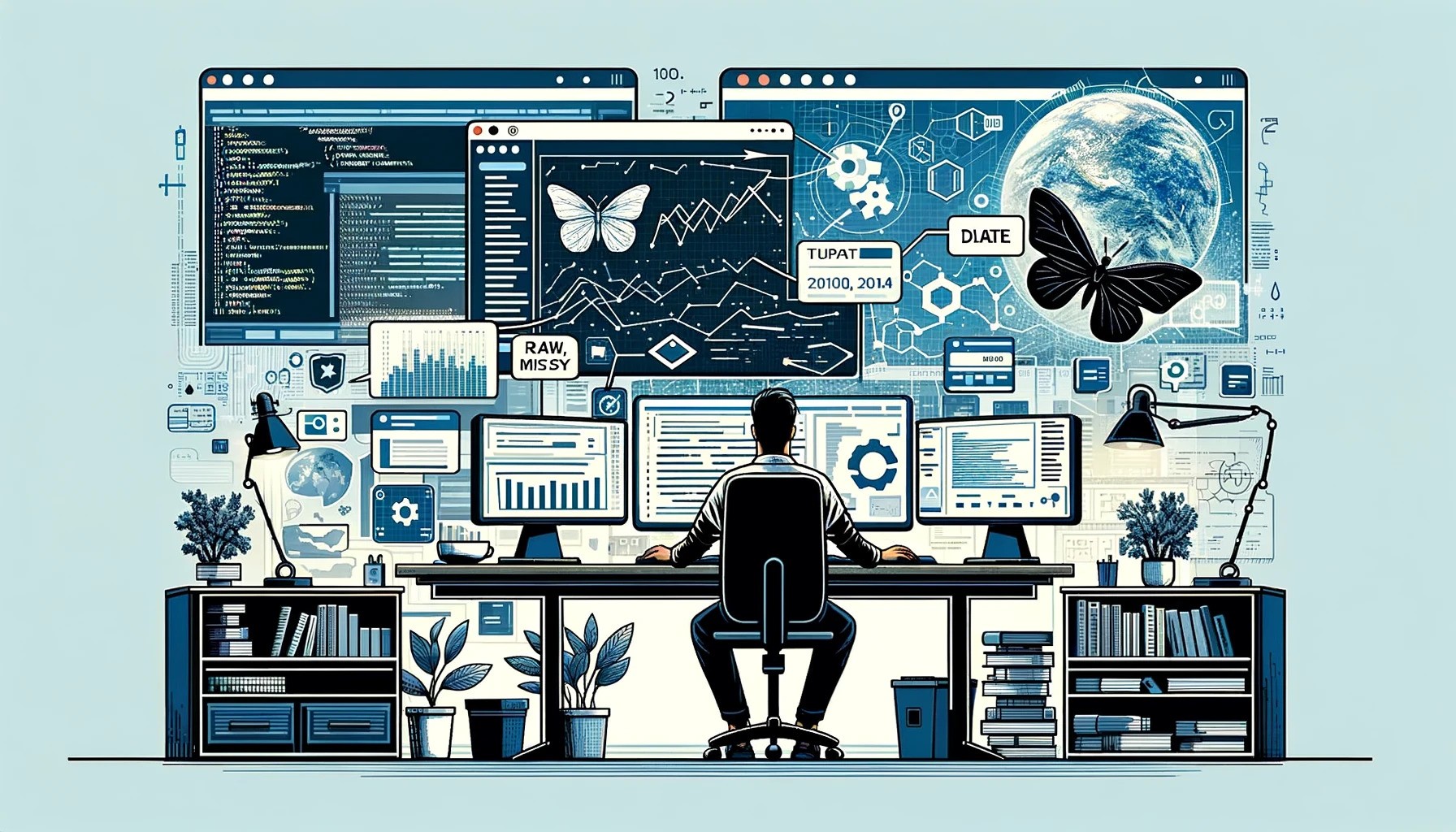
Thomson Reuters Corp. is betting big on generative artificial intelligence, and it has the data foundation in place to do so.
The global provider of professional information for the legal, accounting, government and media industries announced last November that it plans to invest more than $100 million in generative AI and fold the technology into most of its information services. It launched three AI-powered products for the legal industry between November and January, promising to reduce dramatically the time needed to prepare legal briefs, summarize complex documents and ensure policy compliance.
Working with AI is nothing new at the firm, which employs more than 12,000 software developers, data scientists and information technology specialists. It has been experimenting with the technology for over 30 years.
Thomson Reuters’ Cousineau: “Everyone loves and cares about data in this company.” Photo: LinkedIn
“The kind of questions people are asking in the AI world are the questions we’ve been asking for years, and we feel good about that,” said Shawn Malhotra, the company’s head of engineering.
Thomson Reuters can move quickly partly because it has a solid foundation for gathering, validating and curating data. “Our biggest investment is in trust,” said Carter Cousineau, data and model governance vice president. That means setting standards for accuracy and security, ensuring that data has undergone bias detection before being incorporated into a model, testing for false-negative rates and benchmarking performance with human experts.
Most important is that the company has created a data-centric culture. “Everyone loves and cares about data in this company,” Cousineau said. “It’s something we all take pride in.”
Thomson Reuters worked hard to identify data assets across its organizations before generative AI came along. It eliminated outdated and redundant data, defined quality standards, and implemented technology and procedures to ensure up-to-date data.
This basic blocking and tackling is essential if companies are to reap the bounty of large language models, machine learning and other AI applications, experts say. “Poor quality data creates poor quality generation,” said Jitesh Ghai, chief executive officer of Hyland Software Inc and formerly chief product officer at data integration firm Informatica Inc. “While large language models and self-service business intelligence tools are great innovations, the outcomes of those technologies are only as good as the data they rely on.”
Many companies are finding that poor data quality has locked them into the starting blocks on their AI journey. Informatica’s recent survey of 600 data leaders found that while nearly half of organizations have already implemented generative AI, 99% have encountered roadblocks, and 42% of respondents cited data quality as the main obstacle, followed closely by lax data governance.
Organizations have collected a lot of redundant, inaccurate and outdated information as the price of storage declined in recent years, making it cheaper to keep information than to throw it away, said Kevin Cochrane, chief marketing officer at Vultr, the cloud platform owned by The Constant Co. LLC. “It was easy just to store more data and not keep control of it,” he said. “Regaining that control can prove a Herculean task for most companies.”
Organizations that have succeeded in building data-centric cultures are reaping the benefits as AI goes mainstream. Capital One Financial Corp., which started as a credit card provider in 1988 and has become the nation’s ninth-largest bank, has built its reputation on technology-tuned products that serve specific customer segments. The firm employs over 12,000 technologists and devotes a major section of its website to AI-related projects and research.
“Capital One has powered its business model on data-driven decision-making from its very founding,” said Christina Egea, vice president of enterprise data. “From my first days at the company. I couldn’t bring forth an idea not supported by data.”
A central tenet of Capital One’s data-driven culture is to make information as broadly and easily available to its employees as possible. The company has a self-service data platform where users can browse a catalog and discover useful data. Last fall, it published a white paper in the Harvard Business Review about building a data-driven culture.
CapitalOne’s Egea: “From my first days at the company. I couldn’t bring forth an idea not supported by data.” Photo: SiliconANGLE
Sustaining a data-centric culture requires detailed work. Capital One has standardized everything from database schemas to the format used for time stamps. “I’ve lost probably weeks of my life to [International Standards Organization] date formats,” Egea said in a Supercloud 6 video interview with SiliconANGLE (below). “It seems so simple, but we’ve invested in harmonizing that data format across the company because pretty much everybody needs access to timestamps.”
These three organizations are still the exceptions. A November 2022 Gartner Inc. survey of 566 chief data and analytics officers found that only 44% said their data teams effectively provide value to the organization. A January 2023 study by Wavestone S.A. subsidiary NewVantage Partners found that just 26% of data executives at 116 Fortune 1000 companies said they had succeeded in creating a data-driven organization.
“You’d be shocked how many customers don’t have a [data] framework in place,” said Rohit Choudhary, co-founder and CEO of Acceldata Inc., developer of a data observability platform. “In some cases, they have hundreds of petabytes and 35 years of data when the most important assets are just five to seven years old.”
How do you jumpstart generative AI projects from a mess like that? SiliconANGLE contacted more than a dozen experts in AI data management to get their recommendations. The goal is achievable, they agreed, but the key is to start small with well-defined use cases based on the organization’s pockets of good data. Early successes gain buy-in to the value of quality data. Above all, it’s important to enlist human domain experts to oversee model training and validate results.
The good news is that organizations don’t need to boil the ocean to get value from generative AI. Most have islands of structured, tagged and curated data that can be used innovatively.
Acceldata’s Choudhary: “You’d be shocked how many customers don’t have a [data] framework in place.” Photo: Acceldata
For example, Tripadvisor Inc.’s generative AI trip planner “provides a new way for its customers to consume the company’s highly curated data sets of traveler reviews,” said Kjell Carlsson, head of AI strategy at data science platform maker Domino Data Lab Inc. “Similarly, biopharma companies are finding new ways to use their molecular data to accelerate drug discovery by training AI models that in turn suggest real-world proteins to test.” Both examples use a limited corpus of high-quality data.
Know what you’re trying to accomplish and the data you need to achieve those goals, experts advise. “When we have seen generative AI used effectively, it’s always been because the organization had a very clear use case of what they wanted it to do,” said Siân John, chief technology officer at security consulting firm NCC Group plc.
At Thomson Reuters, all new models undergo a proof-of-concept stage that covers “what you’re trying to build and what insights you need,” Cousineau said. “That covers not just governance but privacy, security and intellectual property.” Models are tested for bias, and mitigation procedures are implemented to protect against known risks.
A goals focus can help guide data transformation efforts. At Assurance IQ, “We have a large set of events coming into our data lake, such as interactions with our websites,” Farrell said. “We work with our product team and business leaders to try to understand the key insights we want to take from this information. We map that top-level objective to the low-level data during data transformation.”
IBM’s Calvesbert says early gen AI projects should be internal, “because employees can tell a hallucination.” Photo: LinkedIn
First efforts should be internally focused to avoid the risk of embarrassing and risky hallucinations caused by poorly trained or public LLMs. “A lot of early traction is employee-facing, not customer-facing, because employees can tell a hallucination,” said Edward Calvesbert, director of product management for distributed databases at IBM Corp.
Contact centers are a favorite early use case since most have well-organized and indexed knowledge bases for solving problems. In that context, generative AI becomes “like a coach sitting next to the agents and helping them support users, whether that’s a customer or an internal person,” said Forrester’s Goetz. Customer satisfaction is enhanced, but customers never interact directly with the AI engineer.
Field service is another good early target. “The technician who is busy communicating with the customer, coordinating the schedule and figuring out the tools needed can use a simple voice interface or take a picture of a defective part and have the machine provide a recommendation,” said Vultr’s Cochrane. “You can dramatically improve customer satisfaction, reduce the number of service calls and improve on so many other metrics”
A third good option for LLM neophytes is the IT organization. It deals with highly structured data and IT problems lend themselves well to automated diagnosis. The early success of software development “copilots” makes software engineering a low-hanging fruit.
“In [security operations center] use cases where I have an incident to investigate, and I need to know stuff from a lot of endpoints, machine learning can find all those data points and present them to you,” said NCC’s John.
In those early stages, it’s advisable play it safe. “Start with data that doesn’t have compliance or privacy concerns,” said Starburst Data Inc. CEO Justin Borgman. “Avoid personally identifiable information and sovereign data. The last thing you want is get into deep trouble.”
For organizations that struggle to transform large amounts of data into a form suitable for model training, data lakes are a valuable tool. They allow a large mix of structured, semistructured and unstructured data to be stored, processed and secured so that data cleansing can be an ongoing process rather than a big-bang effort.
Starburst’s Singh: “Start with an open file format and a data lake as the largest center of gravity.” Photo: Starburst Data
“Start with an open file format and a data lake as the largest center of gravity, although not the totality,” said Vishal Singh, head of product for AI, data products, Gravity and Python at Starburst.
Singh recommends using the Apache Iceberg file format to undergird the data lake. Iceberg allows changes to be made to the table schema without breaking it, hides the physical layout of data from users and supports snapshots to maintain a full version history and audit trails.
“With Iceberg, you can train with big models and then see how the data and models have evolved,” he said. “It solves the problems of data warehouses.”
That provenance information is critical to ensuring data quality. “It’s not enough to have a big warehouse and the credentials to query it; you need to understand where the data was sourced from, when it was collected and how reliable it is,” said Jesse Stockall, chief architect at Snow Software Inc. Models invariably degrade over time, so having provenance data allows them to be retrained.
“Make sure there’s the ability to store and trace data from its initial form and not store it in aggregates,” said Vaibhav Vohra, chief product and technology officer at Epicor Software Corp.
At Thomson Reuters, “we look at data across seven different dimensions: accuracy, completeness, conformity, neatness, consistency, coverage and timeliness,” Cousineau said. “We use many different data quality, lineage and validation tools but also human intervention, which is really important.”
NCC’s John: When generative AI is effective, “it’s always because the organization had a very clear use case of what they wanted it to do.” Photo: NCC
Labeling, or affixing metadata tags to operational data, is crucial in model training because it helps training algorithms recognize patterns, make predictions and understand language. “You need good labeling and classification, but most organizations have not spent the time doing that,” said Michele Goetz, a principal analyst at Forrester Research Inc.
Labels shouldn’t be too granular and should map to business concepts, she advised. “Labeling helps build concepts and relationships so the model can identify what works in different scenarios,” Goetz said.
Retrieval-augmented generation can be a godsend for organizations struggling with generative AI. It augments pre-trained language models with external knowledge specific to the business or use case for more reliable and specific results. The technique can also ingest information in real time to deliver up-to-date responses.
“RAG has been a game-changer in that organizations are realizing they don’t need to build models from the ground up,” said Hyland’s Ghai.
IBM’s Calvesbert recommends using RAG with a vector store to train iteratively and test models under development. A vector database is useful for quickly finding data similar to a given example and is widely used in AI model training.
“The lowest risk to deliver real value today is to assemble a knowledge base in a vector store, get it into a RAG pattern and unleash it on your employees,” he said. “They’ll become more productive and give you a feedback loop for the system you’re building.”
Forrester’s Goetz: Most organizations haven’t spent sufficient time on labeling and classifying data. Photo: SiliconANGLE.
There could be a virtue in waiting. Machine learning may ultimately obviate much of the need for manual data cleansing and tagging. Gartner Inc.’s latest Magic Quadrant for data integration tools forecasts that “AI-augmented data management and integration will reduce the need for IT specialists (in particular, data integration developers and data engineers) by up to 40%” by 2026.
“More and more companies are going to use gen AI to help clean and prepare their data, whether for writing dataset descriptions, tagging and categorizing columns or fields or summarizing everything in a data warehouse,” said Ben Schein, senior vice president of product at Domo Inc., a maker of business intelligence software.
IBM uses several models for specialty tasks such as data transformation and governance. “Sophisticated systems have multiple models interacting,” Calvesbert said. “A smaller model might do a good job of understanding sentiment and can trigger a flow based on that. You don’t necessarily want a big model to do that because you can optimize at a lower cost with a smaller one.”
Thomson Reuters’ Malhotra: “The kind of questions people are asking in the AI world are the questions we’ve been asking for years.” Photo: Thomson Reuters
For all the technical challenges AI models present, the cultural issues may be even more formidable. Early adopters said getting AI into end-user hands is crucial to cultivating an appreciation of the importance of quality data. Data silos and ownership issues frustrate the sharing that’s crucial to building a standardized data platform. A company can’t develop meaningful insights about its customers if the marketing department clings tightly to the customer relationship management files.
Thomson Reuters created a sandbox for employees to experiment with LLMs. “Once you engage your whole organization in a safe way to experiment with and try the technology, the questions go from whether we should be using this to how to use it responsibly and safely,” Malhotra said. “Every major technology inflection point happens when things that were only available to deep technologists become available to the entire world.”
Part of gaining buy-in is convincing people that AI is more an opportunity than a threat. Practitioners were unanimous in the opinion that AI will create more jobs than it destroys and can enhance the quality of work for nearly everyone.
They also agreed that the “human-in-the-loop” element is critical.
“I can have the best engineers in the world, but they’re not qualified to say whether a legal summary produced with AI is helpful, harmful, or neutral,” Malhotra said. “Human expertise is essential to creating customer value.”
THANK YOU