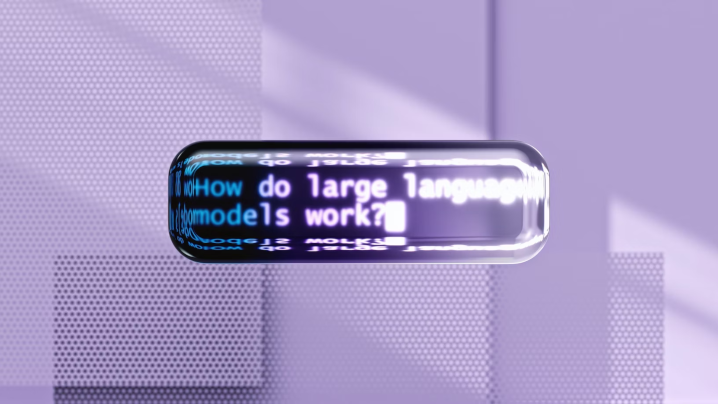
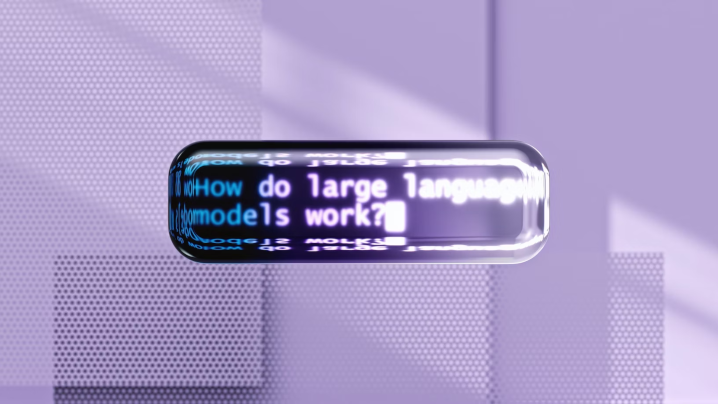
A group of researchers has developed a new benchmark, dubbed LiveBench, to ease the task of evaluating large language models’ question-answering capabilities.
The researchers released the benchmark on Wednesday under an open-source license. The project was sponsored by Abacus.AI Inc., a venture-backed artificial intelligence startup, and included the participation of Turing Award-winning computer scientist Yann LeCun.
LiveBench is designed to address two challenges that the researchers have identified in existing LLM evaluation benchmarks. The first is a phenomenon known as contamination. The other is that software teams often evaluate LLMs’ question-answering prowess using another LLM, which can lead to accuracy issues.
An AI benchmark is a collection of questions used to test neural networks’ knowledge of a given topic. Some benchmarks also contain other types of tasks, such prompts instructing an LLM to debug a code file. By checking how many of the tasks the LLM performs correctly, researchers can gain a better understanding of its capabilities and limitations.
Language models are often trained on large amounts of publicly available web content. In many cases, that content includes answers to questions from popular AI evaluation benchmarks. If an LLM has the answers to a benchmark, it can “cheat” during evaluations, which means the benchmark results won’t accurately reflect its capabilities. This phenomenon is known as contamination in the machine learning ecosystem.
According to LiveBench’s creators, the newly released benchmark can avoid contamination during LLM evaluations. It does so by providing neural networks with tasks to which the answers are unlikely to be included in their training datasets. For added measure, the researchers will regularly refresh LiveBench’s task collection to address the fact that LLMs might eventually obtain answers to the current questions.
“LiveBench is designed to limit potential contamination by releasing new questions monthly, as well as having questions based on recently-released datasets, arXiv papers, news articles, and IMDb movie synopses,” the researches detailed.
During AI accuracy evaluations, language models’ answers to the questions in a benchmark often aren’t scored manually. Instead, researchers use an external LLM such as GPT-4 to check the responses. LiveBench’s creators argue that this approach has limitations because LLMs often make mistakes while evaluating other neural networks’ benchmark responses.
“We show in our paper that for challenging reasoning and math problems, the pass/fail judgments from GPT-4-Turbo have less than a 60% correlation with the true pass/fail judgments,” the researchers detailed. Moreover, they determined that LLMs sometimes erroneously label their own correct benchmark answers as incorrect.
LiveBench addresses those challenges by providing a prepackaged answer to each evaluation question it includes. Using those answers, researchers can determine whether an LLM generated a correct response without having to rely on an external AI system.
The researchers noted that “one weakness is that some types of questions do not have ground-truth answers, such as ‘write a travel guide to Hawaii.’ However, while this limits the type of questions that can be evaluated, it does not affect the validity of evaluation for the questions that can be judged in this manner.”
The current version of LiveBench includes 960 questions across six categories: reasoning, data analysis, math, coding, language comprehension and instruction following. Some of the questions are more challenging versions of test content from existing AI benchmarks. LiveBench’s other tasks change regularly based on information added to frequently-updated public data sources such as arXiv, a popular repository of research papers.
THANK YOU