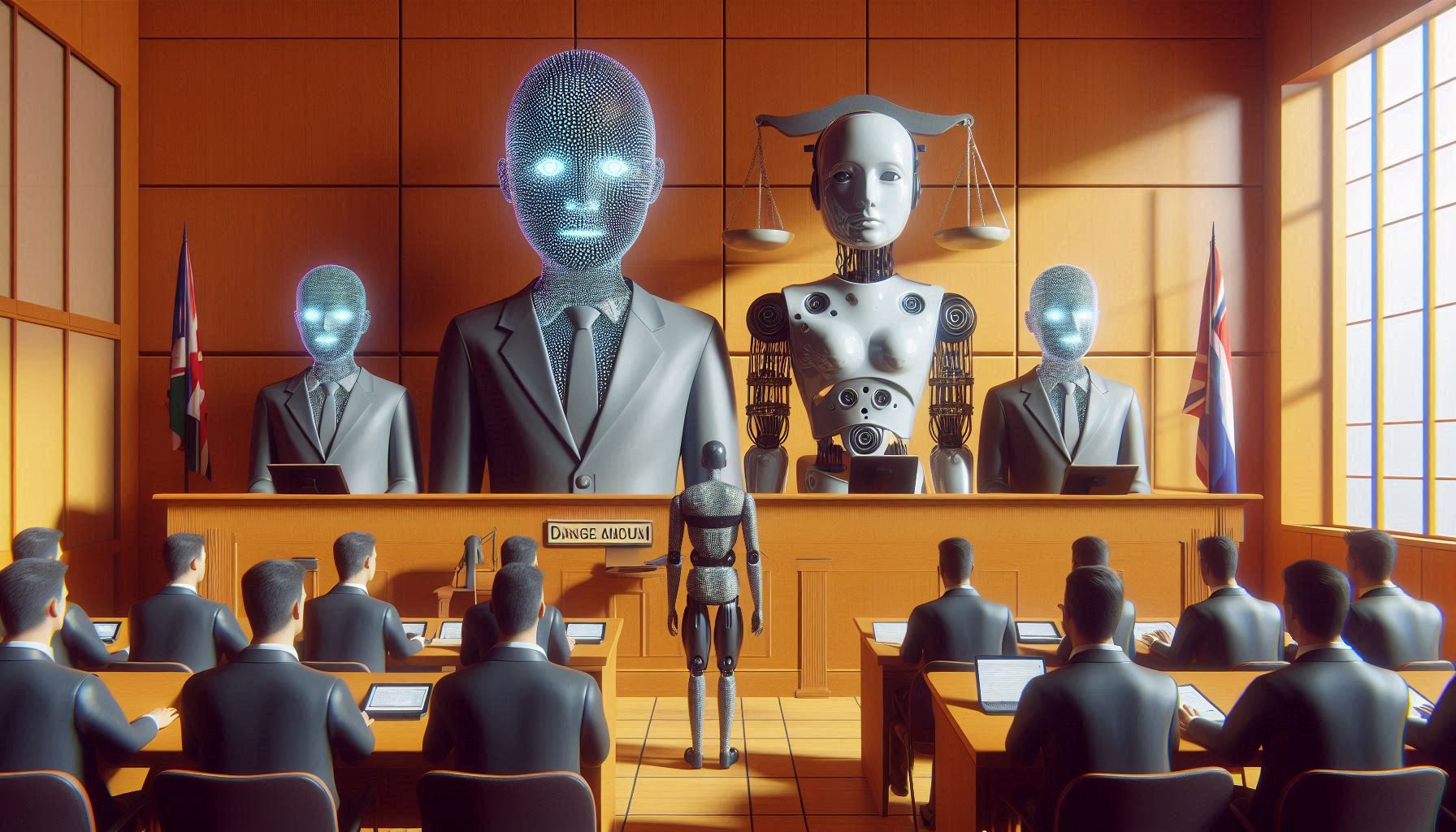
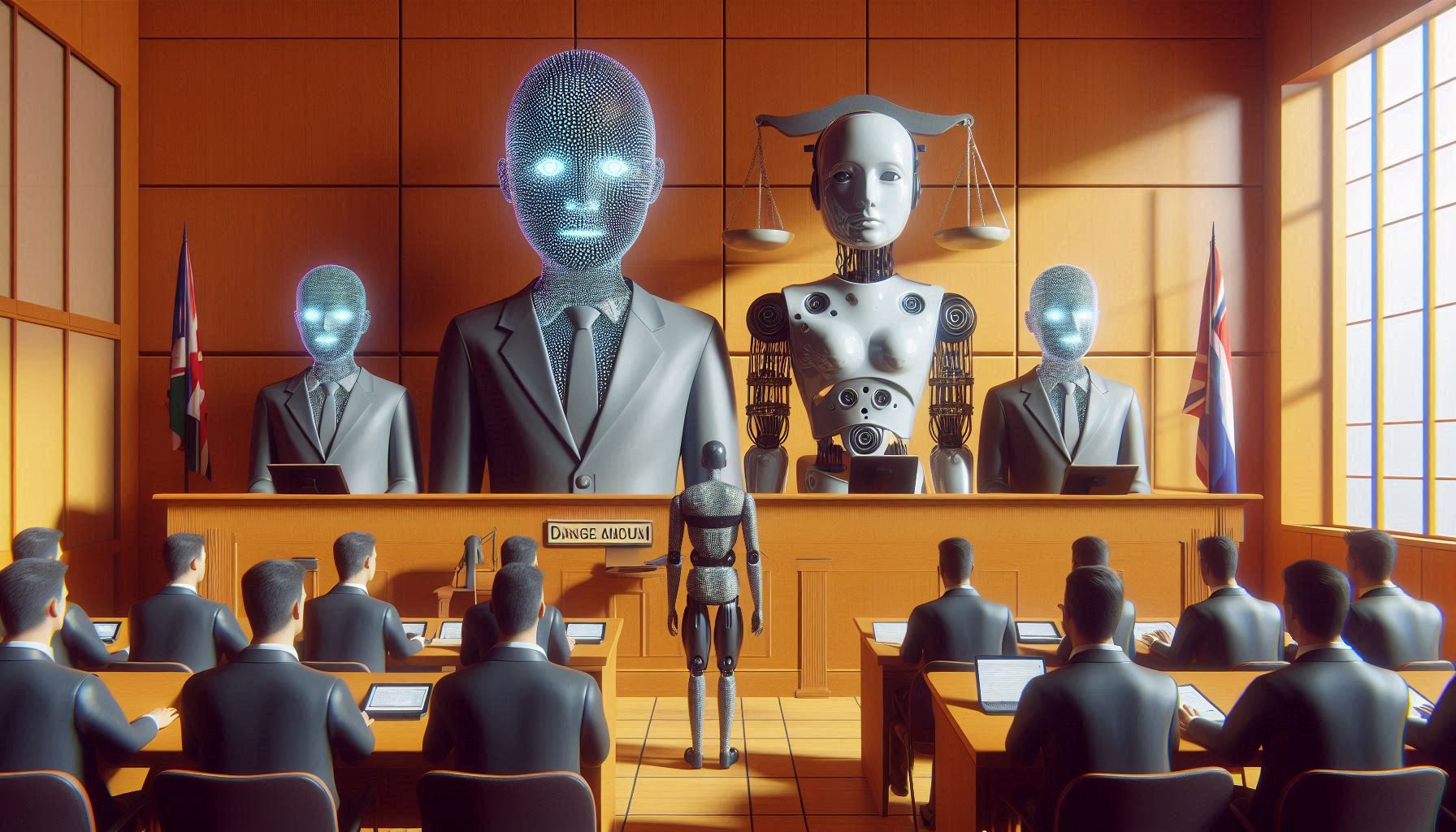
Patronus AI Inc., a startup that provides tools for enterprises to assess the reliability of their artificial intelligence models, today announced the debut of a powerful new “hallucination detection” tool that can help companies to identify when their chatbots are going haywire.
The company says the new model, called Lynx, represents a major breakthrough in the area of AI reliability, enabling enterprises to detect AI hallucinations without the need for manual annotations.
In the AI industry, the phrase “hallucination” is used to describe those moments when large language models generate responses that, while seemingly coherent, do not align with factual reality. LLMs have a preponderance to make things up on the spot when they don’t know how to respond to a user’s prompt or question, and such hallucinations can be dangerous for companies that rely on their AI models to respond accurately to customer’s queries, for example.
AI hallucinations have caused a lot of controversy in the past, with a recent example being Google LLC’s experimental “AI Overviews” feature, which reportedly told one user to “use glue” to stop cheese from falling off their homemade pizza. In another incident, when asked for advice on how best to clean a washing machine, it provided a recipe for what was essentially mustard gas, saying that it’s the best way to get the job done.
Some AI companies have responded to the problem of AI hallucinations by using AI itself to detect them. For example, OpenAI has adapted GPT-4, which powers ChatGPT, to detect inconsistencies in the legendary chatbot’s responses, a concept known as “LLM-as-a-judge.” But there are still concerns over the accuracy of these solutions.
Patronus AI specializes in AI reliability. The company, which raised $17 million in funding two months ago, has created a platform that uses AI to generate what are known as “adversarial prompts” that are designed to test the reliability of LLMs by trying to trick them into generating hallucinations.
According to the startup, Lynx represents the “state-of-the-art” in AI-based hallucination detection, enabling developers to identify inappropriate responses in real-time. Along with Lynx, it has also open-sourced a new benchmark called HaluBench that’s sourced from real world domains to assess faithfulness in LLM responses.
Patronus AI says the performance of Lynx has been thoroughly assessed using HaluBench, and it found that it dramatically outperformed GOT-4 in detecting hallucinations. The largest 70 billion-parameter version of Lynx demonstrated the highest accuracy, beating a number of other LLMs-as-judges that were put through their paces. Patronus AI claims it’s the most powerful hallucination detection model available.
The company explained that HaluBench is designed to test AI models for hallucinations in specific domains, such as healthcare, medicine and finance, making it more applicable to real world use cases.
A sample of Patronus AI’s benchmark results shows that Lynx (70B) was 8.3% more accurate than GPT-4o in terms of detecting medical inaccuracies. Meanwhile, the smaller Lynx (8B) model outperformed the older GPT-3.5 by 24.5% across all HaluBench domains. Similarly, it was 8.6% more effective than Anthropic PBC’s Claude-3-Sonnet, and 18.4% better than Claude-3-Haiku. In addition, Lynx proved to be superior to open-source LLMs, such as Meta Platforms Inc.’s Llama-3-8B-Instruct.
Patronus AI Chief Executive Anand Kannappan said hallucinations are one of the most critical challenges that the AI industry faces. Studies back up such claims, with recent data suggesting that as many as 3% to 10% of all LLM responses are inaccurate.
AI hallucinations can take on many different forms, from leaked training data to exhibiting bias and stating outright lies, such as when ChatGPT falsely accused a prominent law professor of sexual assault. Sometimes, LLMs can go completely off the rails – earlier this year, for example, a beta test version of Microsoft’s Sydney chatbot reportedly professed that it was “in love” with The Verge journalist Nathan Edwards, before confessing to murdering one of its software developers.
Kannappan said these are the kinds of problems Lynx is designed to address. Though he doesn’t claim it can permanently fix AI hallucinations, it can be a valuable tool for developers to measure how likely their LLMs are to start spitting out inaccurate information.
“LLM developers can use [Lynx and HaluBench] to measure the hallucination rate of their fine-tuned LLMs in domain-specific scenarios,” he explained.
The startup said developers can access both Lynx and HaluBench for free via the HuggingFace platform.
THANK YOU