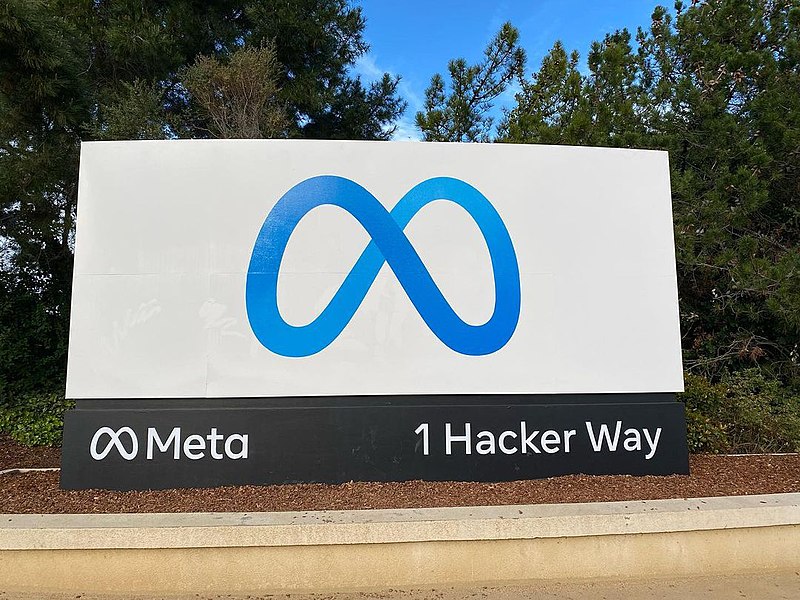
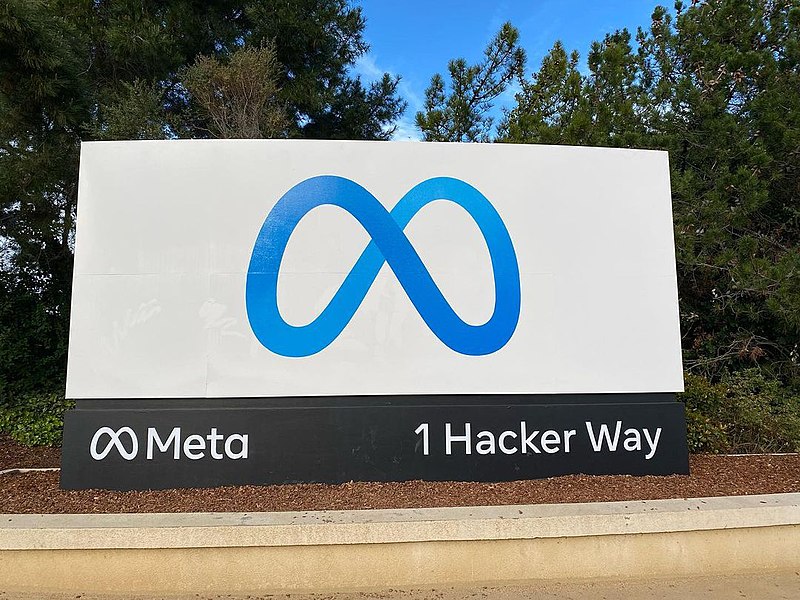
Meta Platforms Inc. is moving to fix an issue that caused its Meta AI chatbot to claim the assassination attempt on Donald Trump didn’t happen.
The Facebook parent announced the development on Tuesday. The disclosure came against the backdrop of recent efforts by other tech giants, notably Google LLC and Baidu Inc., to address safety issues in artificial intelligence models. The two companies this week detailed technical advances designed to mitigate the risks associated with large language models.
Meta AI is a chatbot powered by Llama 3 that rolled out for Facebook, Instagram, WhatsApp and Messenger last year. Following the assassination attempt on Trump, the chatbot told some users that the event didn’t happen. Meta says that the issue emerged in a “small number of cases.”
Joel Kaplan, Meta’s vice president of global policy, detailed in a Tuesday blog post that the company is “quickly working to address” the chatbot’s incorrect answers. He also provided information about the cause of the issue.
Kaplan attributed the problem to hallucinations, a term for situations where an AI model generates inaccurate and nonsensical responses. He detailed that Meta initially configured its chatbot to give “generic response about how it couldn’t provide any information” about the shooting. Following user complaints, the company updated Meta AI to answer questions about the event, which is when the hallucinations started emerging.
“Like all generative AI systems, models can return inaccurate or inappropriate outputs, and we’ll continue to address these issues and improve these features as they evolve and more people share their feedback,” Kaplan wrote.
The executive also addressed a second recent issue in Meta’s AI systems. A few days ago, the systems incorrectly applied a fact-checking label to a photo of Trump that was taken immediately after the assassination attempt. According to Meta, the error emerged because its algorithms had earlier added a fact-checking label to a doctored version of the same photo.
“Given the similarities between the doctored photo and the original image – which are only subtly (although importantly) different – our systems incorrectly applied that fact check to the real photo, too,” Kaplan explained. “Our teams worked to quickly correct this mistake.”
AI safety also came into sharper focus for Google this week. In a blog post published this morning, the company detailed several new steps it’s taking to address the spread of non-consensual sexually explicit deepfakes.
Google provides a mechanism that allows people to request the removal of deepfakes from search results. Going forward, the Alphabet Inc. unit will remove not only the specific file that a user flags through the mechanism but also any copies of the file that it finds on the web. Furthermore, Google will “aim to filter all results on similar searches about” the affected users, product manager Emma Higham wrote in the blog post.
As part of the same effort, Google is taking steps to prevent its search results from incorporating deepfakes in the first place. “For queries that are specifically seeking this content and include people’s names, we’ll aim to surface high-quality, non-explicit content — like relevant news articles — when it’s available,” Higham wrote.
Baidu, the operator of China’s most popular search engine, is also investing in AI safety. On Tuesday, VentureBeat reported that a group of researchers from the company has developed a new “self-reasoning” mechanism for LLMs with RAG, or retrieval-augmented generation, features. The mechanism promises to make such models significantly less likely to generate inaccurate output.
When a RAG-enabled LLM receives a user question, it searches its data repositories for documents that contain relevant information. The Baidu researchers’ self-reasoning mechanism can check that the document a model uses to answer a question is indeed indeed relevant to the user’s inquiry. From there, the mechanism evaluates the specific snippets of text with those documents that the LLM draws upon to generate its response.
The researchers evaluated the technology’s effectiveness using several different AI accuracy benchmarks. During the test, a model equipped with a self-reasoning mechanism achieved performance similar to GPT-4 even though it was trained using significantly less data.
THANK YOU