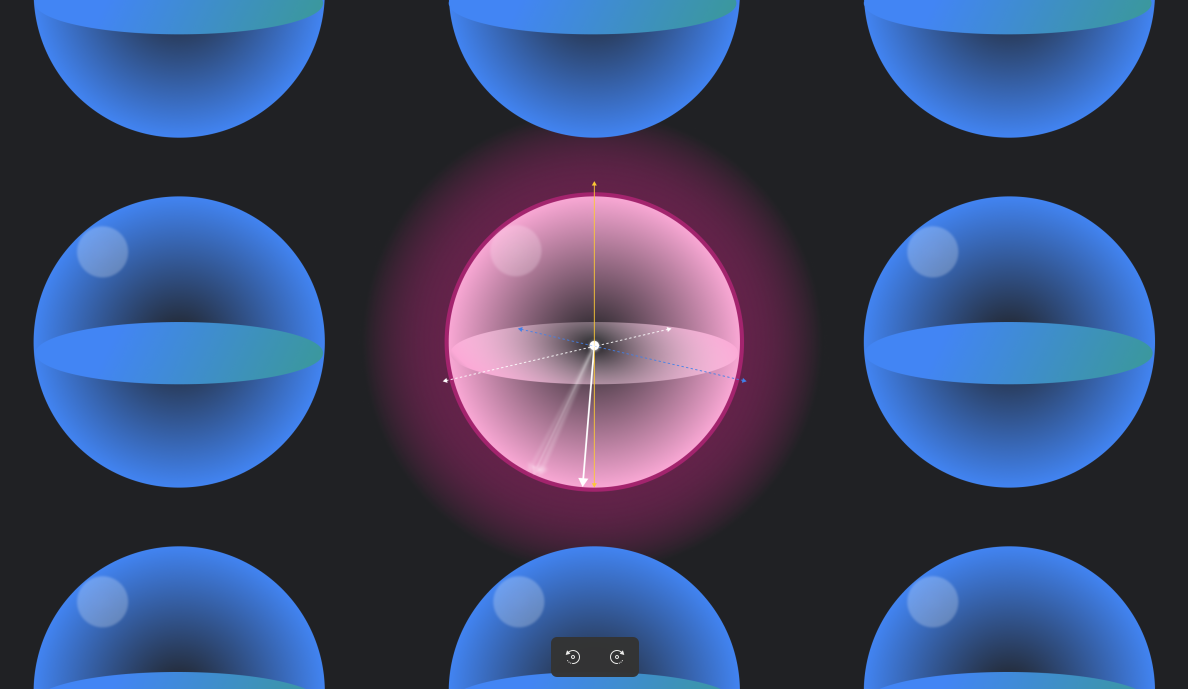
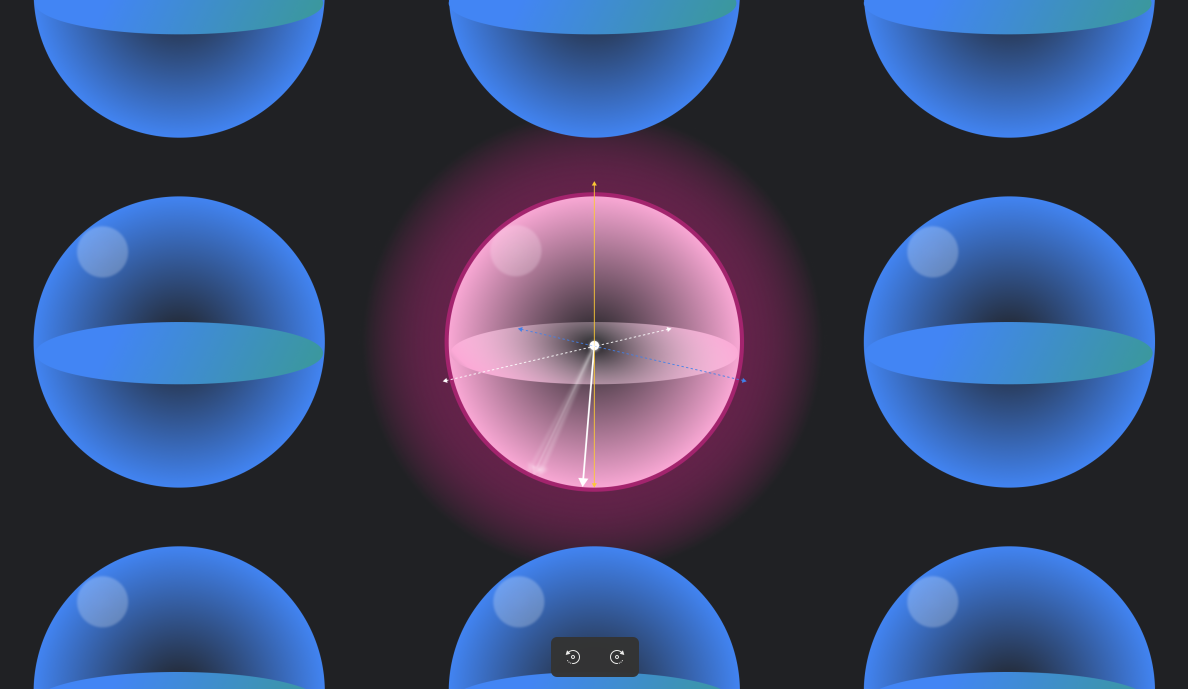
Google DeepMind’s quantum research team says it’s using advanced artificial intelligence algorithms to solve one of the biggest challenges that prevents them from building a reliable quantum computer – error correction.
In a paper published in the journal Nature, the Google DeepMind and Quantum AI teams introduced a new, AI-powered decoder system for quantum computers that can identify computing errors with unparalleled accuracy. Called AlphaQubit, it’s the result of a collaboration that brings together Google DeepMind’s expertise in machine learning with Google Quantum AI’s proficiency in quantum machines.
In the research paper, the authors explained that the ability to accurately detect quantum computing errors is critical for making reliable machines that can scale to tackle the world’s biggest computational challenges. It’s a key step that may one day pave the way to numerous scientific breakthroughs that classical computers will never be able to achieve.
The team explains that quantum computers have the potential to solve problems in a matter of minutes or hours that would take conventional computers years to do. However, one of the major roadblocks in bringing these next-generation systems online is that qubits, the quantum equivalent of traditional “bits,” are extremely unstable and prone to errors. What’s needed is a way to detect these errors, so users can rely on the results generated by quantum machines.
The instability of qubits stems from the way they rely on quantum properties such as superposition and entanglement to solve complex problems in fewer steps. Qubits can sift through vast numbers of possibilities using quantum inference to find solutions, but their natural state is extremely fragile. They can be disrupted all too easily, by microscopic defects in hardware, or even the slightest variation in heat, minuscule vibrations, electromagnetic interference or even cosmic rays.
That explains why existing quantum computers either run at temperatures close to absolute zero, or use novel technologies like lasers to manipulate ion-based qubits held in a vacuum, but while these techniques do help, they don’t completely solve the problem of instability.
By using quantum error correction systems, it’s possible to get around the instability problem by grouping multiple qubits into a single, logical qubit and performing regular consistency checks on them. It then becomes possible to identify logical qubits that deviate from the norm, and correct them when it happens.
The difficulty lies in spotting these errors, and that’s where AlphaQubit can help – Google DeepMind explains that it’s a neural network-based decoder that leverages the same transformer architecture that underpins many of today’s large language models. The team trained AlphaQubit on hundreds of consistency checks, so that it can correctly predict when a logical qubit starts behaving incorrectly.
As the researchers explained:
“We began by training our model to decode the data from a set of 49 qubits inside a Sycamore quantum processor, the central computational unit of the quantum computer. To teach AlphaQubit the general decoding problem, we used a quantum simulator to generate hundreds of millions of examples across a variety of settings and error levels. Then we tuned AlphaQubit for a specific decoding task by giving it thousands of experimental samples from a particular Sycamore processor.”
In the researchers’ tests, AlphaQubit displayed incredible accuracy compared to existing quantum decoders, making 6% fewer errors than tensor network methods in the largest Sycamore experiments. While tensor networks are quite accurate themselves, the problem is that they’re extremely slow. AlphaQubit, on the other hand, can identify errors with more accuracy and at much greater speed, more than fast enough to scale up to handle real-world quantum computing operations.
The researchers said that today’s most powerful quantum computers can achieve only a small fraction of the computing power that the technology will eventually achieve, and so there’s a need to show that AlphaQubit can scale up dramatically. To ensure it can, the researchers trained it on data from simulated quantum systems of up to 241 qubits, which vastly exceeds the number available on Sycamore. Once again, AlphaQubit vastly outperformed existing decoders, indicating it will be able to work with midsized quantum machines in the future.
AlphaQubit has some other useful features too. For instance, it has the ability to report “condence levels” on inputs and outputs, which means there’s lots of potential to improve the performance of quantum processors in future. Moreover, when trained on samples of up to 25 rounds of error correction, the system maintained its high performance for up to 100,000 rounds, meaning it can generalize scenarios far exceeding its training data.
Although AlphaQubit can help improve the reliability of quantum computers, the researchers conceded that there’s a lot of work ahead. For one thing, the system remains too slow to correct errors on a fast superconducting quantum processor in real time.
And as quantum machines grow in size to exceed the millions of qubits necessary to achieve an advantage over classical computers, they’ll need to come up with more efficient ways of training the decoder to handle such a large number.
THANK YOU