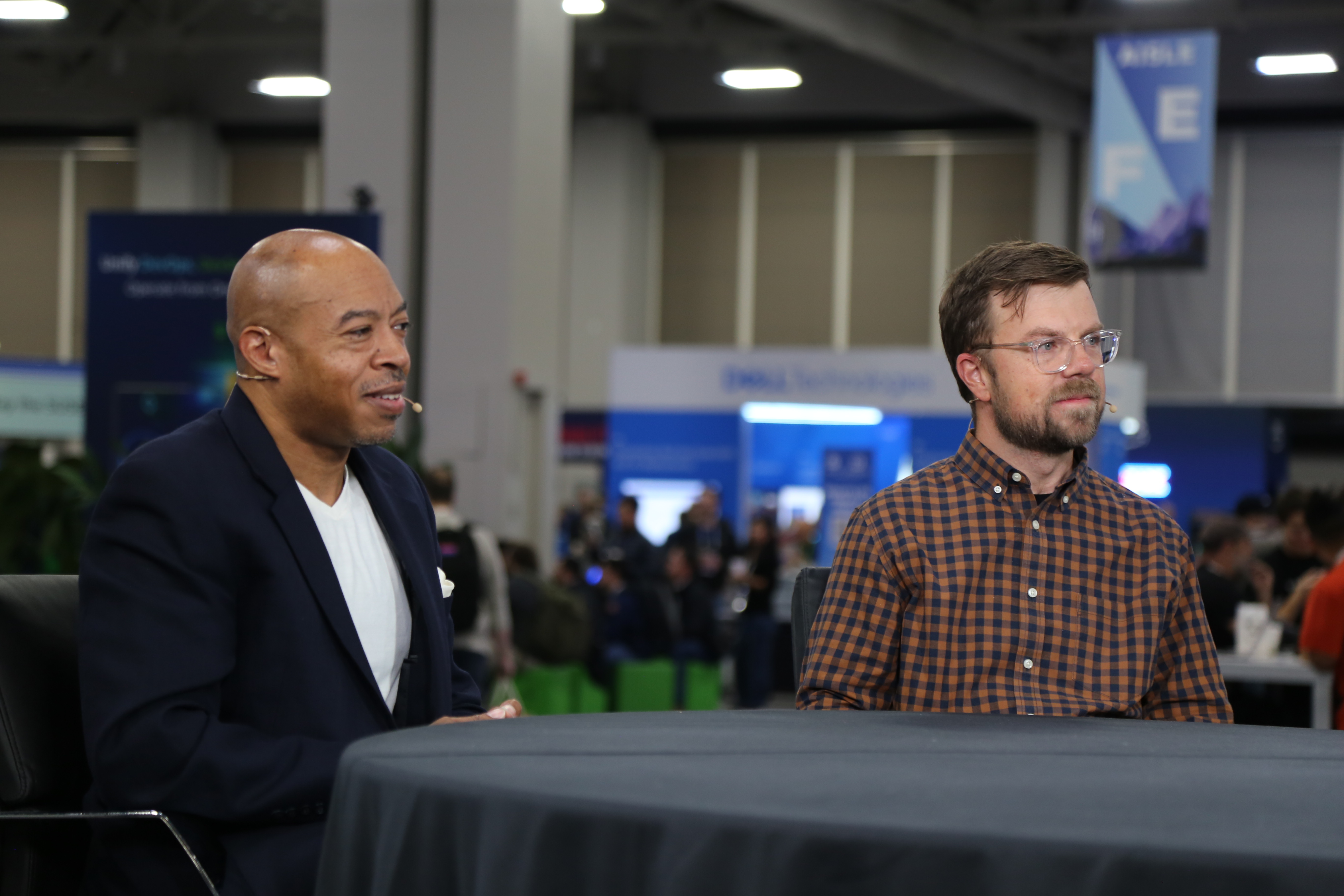
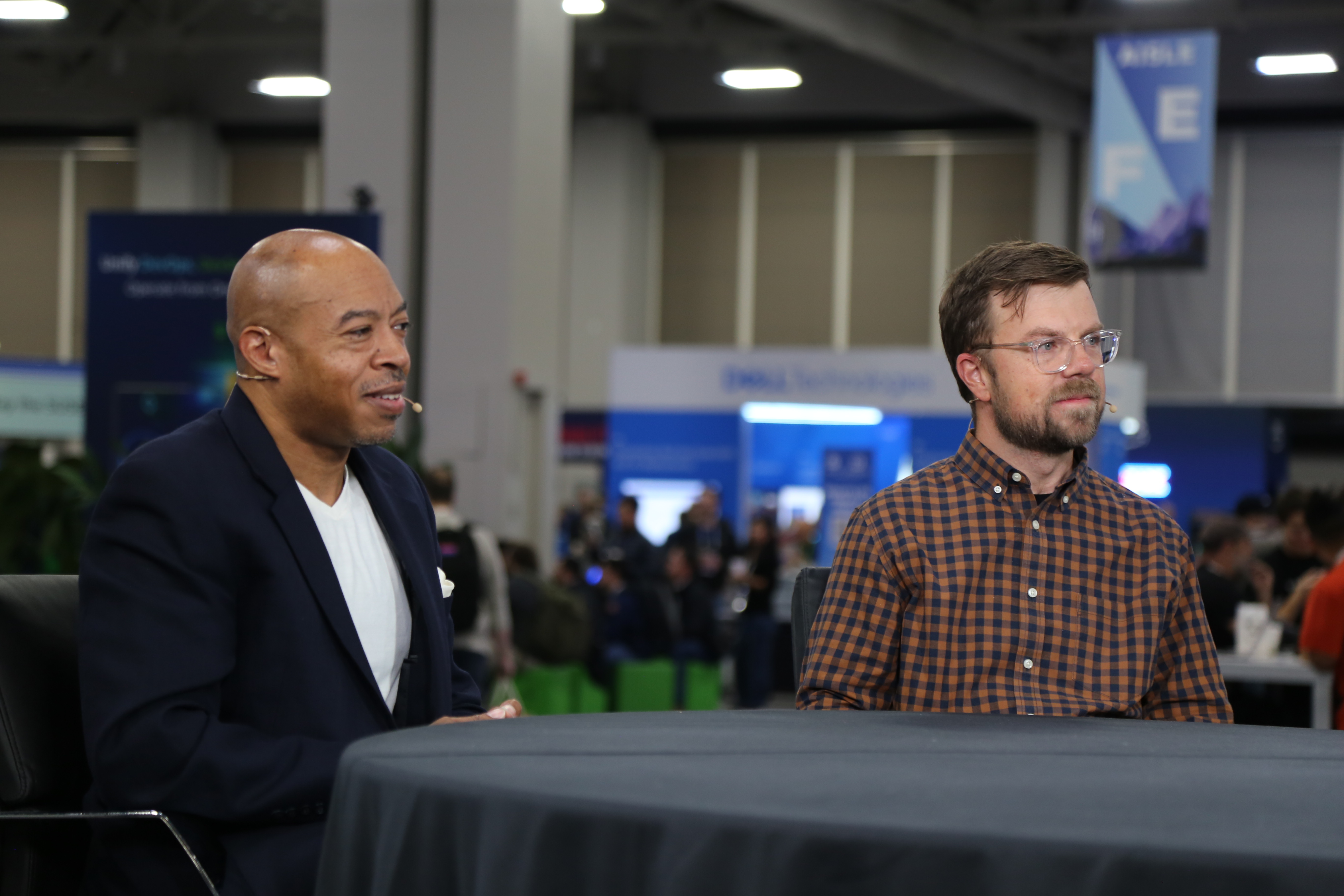
The rapid evolution of artificial intelligence and its integration into cloud-native technologies is reshaping industries. As organizations grapple with the dizzying pace of innovation, thought leaders emphasize the importance of grounding the cloud-native AI conversation in practical applications and strategic frameworks.
Google Cloud’s Bobby Allen and Brandon Royal talk with theCUBE about cloud-native AI technologies.
“You can’t go anywhere without someone sprinkling some AI on a little bit of everything,” said Bobby Allen (pictured, left), cloud therapist at Google LLC. “I think even grandmas and grandpas are messing with AI at this point. I think what we’re also seeing is that it’s becoming … futuristic, but it’s also bleeding into everything. The range of people that want to play with this stuff, that want to touch it, they want to understand it and they want to get their mind wrapped around it. I think people can see the pace or feel the pace speeding up every day.”
Allen and Brandon Royal (right), product manager of AI infrastructure at Google Cloud, spoke with theCUBE Research’s Rob Strechay, on theCUBE, SiliconANGLE Media’s livestreaming studio, for the “Google Cloud: Passport to Containers” interview series. They discussed cloud-native AI technologies as a paradigm shift for data-driven businesses. (* Disclosure below.)
The convergence of cloud-native technologies, Kubernetes and AI is driving transformation across industries. Experts in the field are observing significant trends, including the rising demand for scalable training and inference solutions. Kubernetes plays a considerable role in large-scale AI operations, particularly in training machine learning models and deploying them for inference, according to Royal.
“We’re using deep learning and neural networks essentially under the covers to make models that can do predictions,” he said. “Now if you look at AI as we define it today, sort of modern AI, we use things like large language models, or LLMs, and those are technologies that can be pre-trained with a whole bunch of data. Think of all of the knowledge of the internet and human civilization codified into a single model that can then be delivered to people open source or is available on the open internet.”
Inference, on the other hand, is the application phase. It enables users to access AI models via APIs to perform tasks such as generating insights or automating processes. This critical step brings AI’s value to life, bridging the gap between development and real-world utility. However, organizations often misunderstand the complexity of moving from training to inference. Fine-tuning pre-trained models or leveraging off-the-shelf solutions can simplify this transition, allowing companies to deliver AI-driven applications faster.
“A model is only valuable until we can put it behind an API and make it available to do something interesting,” Royal said. “That’s really what inference is all about. It’s taking a model, whether it’s a large language model, a diffusion model for images or a simple model, and providing an endpoint by which we can expose that to users. That’s really where the fun and interesting stuff happens. You train a model, you evaluate the model, you test the model, and then eventually you take all that work and put your model out there and you deliver it.”
The explosion of pre-trained AI models has created new opportunities for organizations to jumpstart their AI initiatives. Companies no longer need to develop every aspect of a model from scratch. Instead, they can adopt pre-trained models, such as Google’s Gemini, or open-source options from platforms such as Hugging Face.
“Evaluations are now like a sub-domain in and of itself,” Royal said. “How do you evaluate the performance of a model? It used to actually be pretty simple. To evaluate performance, you give it the same sort of input and it gives you a similar kind of output and you can validate. Is that better or worse? But now that we’re getting into large language models, doing that evaluation is quite human. It’s quite subjective.”
Enterprises must weigh performance metrics and benchmarks against their unique needs, Royal added. Tools and frameworks that facilitate these evaluations are evolving, enabling businesses to make informed decisions about deploying AI at scale.
Looking ahead, experts predict significant advancements in distributed computing for cloud-native AI and machine learning. Distributed training and inference across Kubernetes clusters will allow organizations to deploy AI models more efficiently across regions. This approach ensures that businesses can meet global demands without compromising performance, according to Allen.
“Distributing models in a more efficient way is going to become much more of a trend,” he said. “Up to this point … it’s really the more advanced organizations that are doing that kind of work. But now, as frameworks are starting to mature, it’s easier and easier to do distributed training and distributed inference across Kubernetes clusters or nodes or whatever it happens to be.”
In one use case example, the AI-powered advertising solutions company, Moloco Inc., managed 10 times faster model training times using Google’s Kubernetes Engine. The company leverages predictions from several deep neural networks while continuously designing and evaluating new models. In another example, LiveX AI Inc. achieved over 50% lower TCO with custom AI agents trained and served on GKE and Nvidia.
Here’s theCUBE’s complete video interview with Bobby Allen and Brandon Royal:
(* Disclosure: Google Cloud sponsored this segment of theCUBE. Neither Google Cloud nor other sponsors have editorial control over content on theCUBE or SiliconANGLE.)
THANK YOU