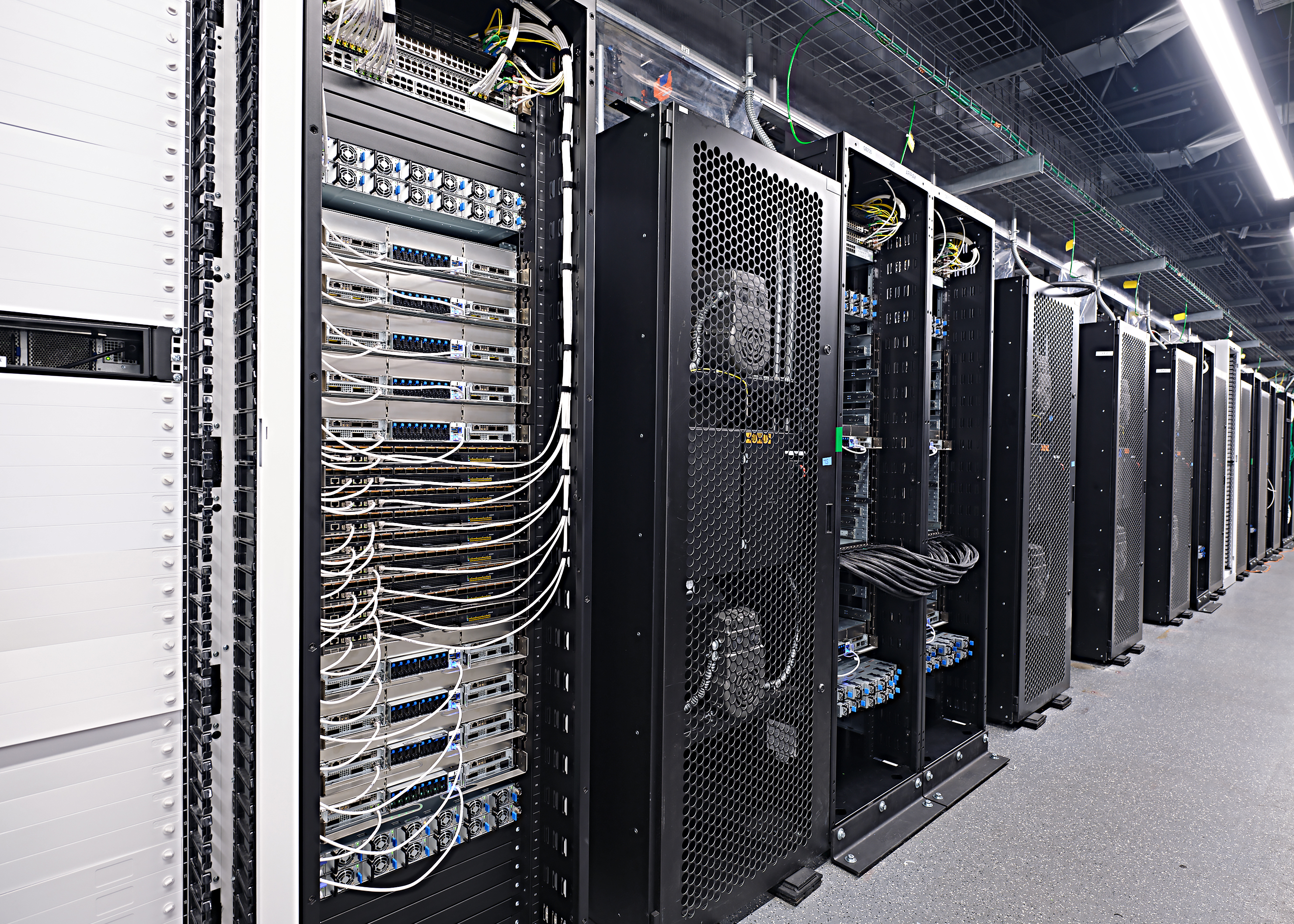
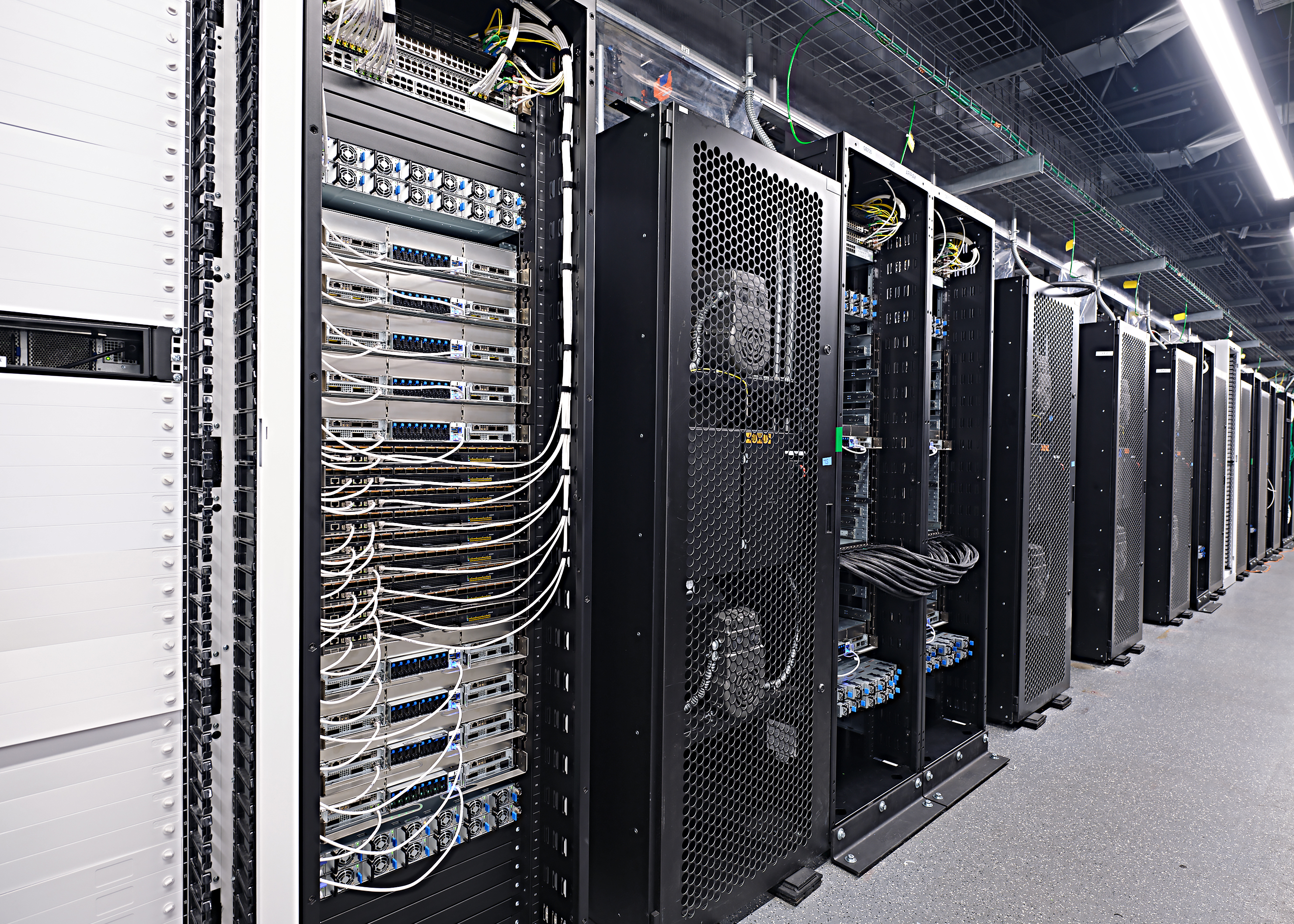
We are witnessing the rise of a completely new computing era. Within the next decade, a trillion-dollar-plus data center business is poised for transformation, powered by what we refer to as extreme parallel computing, or EPC — or as some prefer to call it, accelerated computing. Though artificial intelligence is the primary accelerant, the effects ripple across the entire technology stack.
Nvidia Corp. sits in the vanguard of this shift, forging an end-to-end platform that integrates hardware, software, systems engineering and a massive ecosystem. Our view is that Nvidia has a 10- to 20-year runway to drive this transformation, but the market forces at play are much larger than a single player. This new paradigm is about reimagining compute from the ground up: from the chip level to data center equipment, to distributed computing at scale, data and applications stacks and emerging robotics at the edge.
In this Breaking Analysis, we explore how extreme parallel computing is reshaping the tech landscape, the performance of the major semiconductor players, the competition Nvidia faces, the depth of its moat, and how its software stack cements its leadership. We will also address a recent development from CES — the arrival of so-called “AI PCs” — with data from Enterprise Technology Research. We’ll then look at how the data center market could reach $1.7 trillion by 2035. Finally, we will discuss both the upside potential and the risks that threaten this positive scenario.
Our research indicates that every layer of the technology stack — from compute to storage to networking to the software layers — will be re-architected for AI-driven workloads and extreme parallelism. We believe the transition from general-purpose, x86, central processing units toward distributed clusters of graphics processing units and specialized accelerators is happening even faster than many anticipated. What follows is our brief assessment of several layers of the data center tech stack and the implications of EPC.
For more than three decades, x86 architectures dominated computing. Today, general-purpose processing is giving way to specialized accelerators. GPUs are the heart of this change. AI workloads such as large language models, natural language processing, advanced analytics and real-time inference demand massive concurrency.
While storage is sometimes overlooked in AI conversations, data is the fuel that drives neural networks. We believe AI demands advanced, high-performance storage solutions:
With mobile and cloud last decade we saw a shift in network traffic from a north-south trajectory (user-to-data center) toward an east-west bias (server-to-server). AI-driven workloads cause massive east-west and north-south traffic within the data center and across networks. In the world of HPC, InfiniBand emerged as the go-to for ultra-low-latency interconnects. Now, we see that trend permeate hyperscale data centers, with high-performance Ethernet as a dominant standard which will ultimately in our view prove to be the prevailing open network of choice:
OS and system-level software
Accelerated computing imposes huge demands on operating systems, middleware, libraries, compilers and application frameworks. These must be tuned to exploit GPU resources. As developers create more advanced applications — some bridging real-time analytics and historical data — system-level software must manage concurrency at unprecedented levels. The OS, middleware, tools, libraries and compilers are rapidly evolving to support ultra-parallel workloads with the ability to exploit GPUs (that is, GPU-aware OSes).
Data layer
Data is the fuel for AI and the data stack is rapidly becoming infused with intelligence. We see the data layer shifting from an historical system of analytics to a real-time engine that supports the creation of real time digital representations of an organization, comprising people, places and things as well as processes. To support this vision, data harmonization via knowledge graphs, unified metadata repositories, agent control frameworks, unified governance and connectors to operational and analytic systems will emerge.
The application layer
Intelligent applications are emerging that unify and harmonize data. These apps increasingly have real time access to business logic as well as process knowledge. Single-agent systems are evolving to multi-agent architectures with the ability to learn from the reasoning traces of humans. Applications increasingly can understand human language, are injecting intelligence (in other words, AI everywhere) and supporting automation of workflows and new ways of creating business outcomes. Applications increasingly are becoming extensions to the physical world with opportunities in virtually all industries to create digital twins that represent a business in real time.
Key takeaway: Extreme parallel computing represents a wholesale rethinking of the technology stack — compute, storage, networking and especially the operating system layer. It places GPUs and other accelerators at the center of the architectural design.
The above graphic shows the five-year stock performance of major semiconductor players, with the “AI zone” shaded starting in late 2022 — roughly coinciding with the initial buzz around ChatGPT. Until that timeframe, many were skeptical that large-scale GPU-accelerated AI would become such a powerful business driver.
In our view, the market has recognized that semiconductors are the foundation of future AI capabilities, awarding premium multiples to companies that can capture accelerated compute demand. This year, the “haves” (led by Nvidia, Broadcom and AMD) are outperforming, while the “have-nots” (in particular, Intel) are lagging.
Nvidia’s 65% operating margins have enticed investors and competitors to enter the AI chip market in droves. Both incumbents and new entrants have responded aggressively. Yet the market potential is so large and Nvidia’s lead is so substantial that in our view, near-term competition will not hurt Nvidia. Nonetheless, we see multiple angles regarding Nvidia’s challengers, each with its own market approach.
We align these two leaders because: 1) Broadcom powers custom chips such as Google’s tensor processing units or TPUs; and 2) We believe that TPU v4 is extremely competitive in AI. Broadcom’s IP around SerDes, optics and networking is best-in-class and together with Google represents in our view the most viable technical alternative relative to Nvidia.
Importantly, Broadcom also has had a long-term relationship with Meta and powers its AI chips. Both Google and Meta have proved that AI return on investment in consumer advertising pays off. While many enterprises struggle with AI ROI, these two firms are demonstrating impressive return on invested capital from AI.
Both Google and Meta are leaning into Ethernet as a networking standard. Broadcom is a strong supporter of Ethernet and a leading voice in the Ultra Ethernet Consortium. Moreover, Broadcom is the only company other than Nvidia with proven expertise on networking within and across XPUs and across XPU clusters, making the company an extremely formidable competitor in AI silicon.
AMD’s data center strategy hinges on delivering competitive AI accelerators — building on the company’s track record in x86. Though it has a serious GPU presence for gaming and HPC, the AI software ecosystem (centered on CUDA) remains a key obstacle.
AMD has made aggressive moves in AI. It is aligning with Intel to try to keep x86 viability alive. It has acquired ZT Systems to better understand end-to-end AI systems requirements and will be a viable alternative of merchant silicon, especially for inference workloads. Ultimately we believe AMD will capture a relatively small share (single digits) of a massive market. It will manage x86 market declines by gaining share against Intel and make inroads into cost sensitive AI chip markets against Nvidia.
Once the undisputed leader in processors, Intel’s fortunes have turned amid the shift toward accelerated compute. We continue to see Intel hampered by the massive capital requirements of retaining its own foundry.
Amazon’s custom silicon approach has succeeded with Graviton in CPU instances. Its acquisition of Annapurna Labs is one of the best investments in the history of enterprise tech. And certainly it’s one that is often overlooked. Today, AWS works with Marvell and is applying a Graviton-like strategy to GPUs with Trainium (for training) and Inferentia (for inference).
Dylan Patel’s take on Amazon’s GPU sums it up in our view. Here’s what he said on a recent episode of the BG2 pod:
Amazon, their whole thing at re:Invent, if you really talk to them when they announce Trainium 2 and our whole post about it and our analysis of it is supply chain-wise… you squint your eyes, This looks like a Amazon Basics TPU, right? It’s decent, right? But it’s really cheap, A; and B, it gives you the most HBM capacity per dollar and most HBM memory bandwidth per dollar of any chip on the market. And therefore it actually makes sense for certain applications to use. And so this is like a real shift. Like, hey, we maybe can’t design as well as Nvidia, but we can put more memory on the package.
[Dylan Patel’s take on Amazon Trainium]
Our view is that AWS’ offering will be cost-optimized — and offer an alternative GPU approach within the AWS ecosystem for both training an inference. Though developers ultimately may prefer the familiarity and performance of Nvidia’s platform, AWS will offer as many viable choices to customers as it can and will get a fair share of its captive market. Probably not the penetration it sees with Graviton relative to merchant x86 silicon but a decent amount of adoption to justify the investment. We don’t have a current forecast for Trainium at this time, but it’s something we’re watching to get better data.
Microsoft has historically lagged AWS and Google in custom silicon, though it does have ongoing projects, such as Maia. Microsoft can offset any silicon gap with its software dominance and willingness to pay Nvidia’s margins for high-end GPUs. Qualcomm is a key supplier for Microsoft client devices. Qualcomm, as indicated, competes in mobile and edge, but as robotics and distributed AI applications expand, we see potential for more direct clashes with Nvidia.
Firms such as Cerebras Systems Inc., SambaNova Systems Inc., Tenstorrent Inc. and Graphcore Ltd. have introduced specialized AI architectures. China is also developing homegrown GPU or GPU-like accelerators. However, the unifying challenge remains software compatibility, developer momentum and the steep climb to dislodge a de facto standard.
Key takeaway: Though competition is strong, none of these players alone threatens Nvidia’s long-term dominance — unless Nvidia makes significant missteps. The market’s size is vast enough that multiple winners can thrive.
We see Nvidia’s competitive advantage as a multifaceted moat spanning hardware and software. It has taken nearly two decades of systematic innovation to produce an integrated ecosystem that is both broad and deep.
Nvidia’s GPUs employ advanced process nodes, including HBM memory integration and specialized tensor cores that deliver huge leaps in AI performance. Notably, Nvidia can push a new GPU iteration every 12 to 18 months. Meanwhile, it uses “whole cow” methods — ensuring that every salvageable die has a place in its portfolio (data center, PC GPUs or automotive). This keeps yields high and margins healthy.
The acquisition of Mellanox Technologies Ltd. put Nvidia in control of InfiniBand, enabling it to sell comprehensive end-to-end systems for AI clusters and get to market quickly. The integration of ConnectX and BlueField DPUs extends Nvidia’s leadership in ultra-fast networking, a critical component for multi-GPU scaling. As the industry moves toward the Ultra Ethernet standard, many see this as a threat to Nvidia’s moat. We do not. Though networking is a critical component of Nvidia’s time to market advantage, we see it as a supporting member of its portfolio. In our view, the company can and will successfully optimize its stack for Ethernet as the market demands; and it will maintain its core advantage, which comes from tight integration across its stack.
Nvidia’s software ecosystem has grown far beyond CUDA to include frameworks for nearly every stage of AI application development. The net result is that developers have more reason to stay within Nvidia’s ecosystem rather than seeking alternatives.
Jensen Huang, Nvidia’s CEO, has frequently underscored the company’s emphasis on building a partner network. Virtually every major tech supplier and cloud provider offers Nvidia-based instances or solutions. That broad footprint generates significant network effects, reinforcing the moat.
Key takeaway: Nvidia’s advantage does not hinge on chips alone. Its integration of hardware and software — underpinned by a vast ecosystem — forms a fortress-like moat that is difficult to replicate.
CUDA rightly dominates the software discussion, but Nvidia’s stack is broad. Below we highlight six important layers: CUDA, NVMI/NVSM (here denoted as “NIMS”), Nemo, Omniverse, Cosmos and Nvidia’s developer libraries/toolkits.
Compute Unified Device Architecture (CUDA) is Nvidia’s foundational parallel computing platform. It abstracts away the complexities of GPU hardware and allows developers to write applications in languages like C/C++, Fortran, Python, and others. CUDA orchestrates GPU cores and optimizes workload scheduling for accelerated AI, HPC, graphics, and more.
Nvidia NIMS are a set of inference microservices designed to simplify and accelerate the deployment of foundation models on any cloud or data center.
NeMo is an end-to-end framework for developing and fine-tuning large language models and natural language applications. It provides pre-built modules, pretrained models, and the tooling to export those models into other Nvidia products, helping speed time-to-insight for businesses that want to leverage NLP and large language models.
Omniverse is a platform for 3D design collaboration, simulation, and real-time visualization. While originally showcased for design engineering and media, Omniverse now extends into robotics, digital twins and advanced physics-based simulations. It leverages CUDA for graphical rendering, combining real-time graphics with AI-driven simulation capabilities.
Cosmos is a platform that helps developers create world models for physical AI systems. It’s used to accelerate the development of robotics and autonomous vehicles.
Beyond the core frameworks, Nvidia has developed hundreds of specialized libraries for neural network operations, linear algebra, device drivers, HPC applications, image processing and more. These libraries are meticulously tuned for GPU acceleration — further locking in the developer community that invests time to master them.
Key takeaway: The software stack is arguably the most important factor in Nvidia’s sustained leadership. CUDA is only part of the story. The depth and maturity of Nvidia’s broader AI software suite forms a formidable barrier to entry for new challengers.
Although this Breaking Analysis focuses on data center transformation, we would be remiss not to discuss AI PCs briefly. At CES this year, multiple vendors announced laptops and desktops branded as “AI PCs,” often featuring NPUs (neural processing units) or specialized GPUs for on-device inference.
Survey data shown above is from ETR in a survey of approximately 1,835 information technology decision makers. The vertical axis is Net Score or spending momentum and the horizontal axis is Overlap or penetration within those 1,835 accounts. The table insert shows how the dots are plotted (Net Score and N). It shows Dell laptops at the top of the share curve with 543 N, with strong spending momentum across Apple, HP, and Lenovo. The plot reveals healthy spending momentum for leading PC suppliers.
Currently, the NPU often sits idle in many AI PCs because software stacks are still not fully optimized. Over time, we expect more specialized AI applications on client devices — potentially enabling real-time language translation, image/video processing, advanced security and local LLM inference at smaller scales.
We believe Nvidia, with its track record in GPUs, can offer AI PC technology that is more performant than typical NPUs on mobile or notebooks. However, the power consumption, thermals, and cost constraints remain significant challenges. We do see Nvidia using salvaged “whole cow” dies and building them into laptop GPUs with reduced power envelopes.
Although this section deviates from the data center focus, AI PCs could drive developer adoption. On-device AI makes sense for productivity, specialized workloads, and specific vertical use cases. This, in turn, may reinforce the broader ecosystem transition to parallel computing architectures.
We have modeled the entire data center market — servers, storage, networking, power, cooling and related infrastructure — from 2019 through 2035. Our research points to a rapid transition away from traditional general-purpose computing toward extreme parallel computing.
We categorize “extreme parallel computing” as the specialized hardware and software for AI training, inference, HPC clusters and advanced analytics.
Currently, we estimate that Nvidia accounts for roughly 25% of the entire data center segment. Our view is that Nvidia will retain that leading share throughout the forecast period — assuming it avoids unforced errors — despite intense competition from hyperscalers, AMD and others.
Key takeaway: The anticipated shift toward accelerated compute forms the foundation of our bullish stance on data center growth. We believe extreme parallel computing ushers in a multi-year (or even multi-decade) supercycle for data center infrastructure investments.
We assert that a new trillion-dollar-plus marketplace is emerging, fueled by AI. The data center — as we have known it — will transform into a distributed, parallel processing fabric where GPUs and specialized accelerators become the norm. Nvidia’s tightly integrated platform (hardware + software + ecosystem) leads this transition, but it is not alone. Hyperscalers, competing semiconductor firms, and specialized startups all have roles to play in a rapidly expanding market.
Despite our positive assessment, we acknowledge several risks:
Final word: Nvidia’s future looks bright, in our view, but it cannot be complacent. The company’s best defense remains relentless innovation in both hardware and software — a strategy that has carried it to where it is today and will likely drive its continued leadership in this new era of extreme parallel computing.
THANK YOU