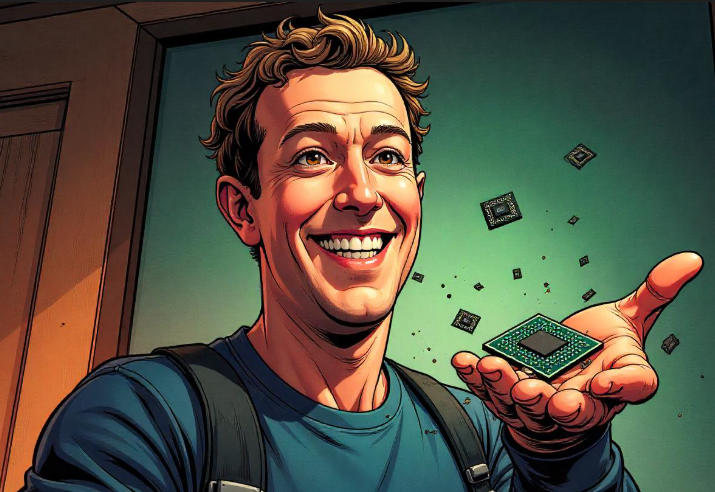
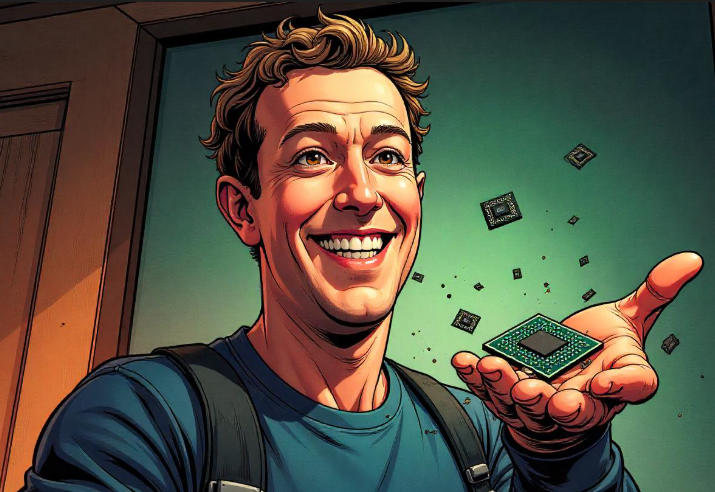
Meta Platforms Inc. is forging ahead with its plans to reduce its reliance on Nvidia Corp.’s graphics processing units, and is currently testing its first in-house artificial intelligence training chip.
According to Reuters, the test process involves manufacturing an initial small batch of chips, and if it proves to be successful, the company will look to gear up production quickly.
The in-house chip test is part of an effort by Meta to try to rein in its spending at a time when it’s investing heavily in the AI infrastructure it believes will be necessary to stay at the forefront of the industry. The company, which owns Instagram and WhatsApp as well as Facebook, has said it will spend about $114 billion to $119 billion in 2025. Up to $65 billion of that amount will go to capital expenditures, which are primarily directed at AI infrastructure.
By making its own chips for AI training, Meta would not need to buy so many expensive GPUs from Nvidia and other suppliers. Several big technology companies, including cloud giants such as Amazon Web Services Inc. and Google Cloud, already mass-produce their own AI processors. OpenAI is trying to do the same, and two months earlier it said it aims to finalize its chip design later this year.
An anonymous source told Reuters that Meta’s new chip is a dedicated AI accelerator that’s purpose-built for training large language models. This should mean it’s more power-efficient than Nvidia’s general-purpose GPUs.
The company is working with Taiwan Semiconductor Manufacturing Co. to manufacture its new chip. The test comes following the successful completion of Meta’s first “tape-out,” which is a significant step that involves sending the initial design of the chip to a manufacturing partner, in order to assess that it is feasible. The tape-out phase is extremely expensive, with costs typically running into tens of millions of dollars, and it often takes between three and six months to complete.
Neither Meta nor TSMC were inclined to comment, but Reuter’s sources say the new chip is part of Meta’s Meta Training and Inference Accelerator series of chips, which have seen mixed success to date. The social media giant was forced to scrap an earlier MTIA chip design during the development process, but last year it managed to deploy its first processors, designed specifically for inference tasks. That chip is now powering Meta’s AI-based recommendation systems, which determine the content that appears in users’ Facebook and Instagram feeds.
When Meta abandoned its first MTIA chip in 2022, it had no option but to double down on Nvidia’s GPUs, and it has ordered billions of dollars’ worth of those chips since then. The GPUs are used for both training and inference, as well as recommendations and ads.
If the latest test is successful, Meta wants to start using its in-house chips to train its next-generation Llama LLMs. That will enable it to scale back on its GPU purchases.
Holger Mueller of Constellation Research Inc. said Meta is following the proven lead of the big three cloud infrastructure vendors in designing its own in-house chip architecture, having come to the conclusion that Nvidia’s chips are too expensive and power-hungry.
“This is why Google is leading the AI race, because it had a three-to-four year headstart, but not all of these in-house chips were immediately successful, which is why Nvidia leads in the data center today,” the analyst said. “As for Meta, it will want to make sure that its chips perform better than Nvidia’s in terms of both price and performance. It’s likely going to have to keep using Nvidia’s GPUs in parallel with its own for a while, as no vendor has been able to create a V1 chip that could compete with Nvidia straight away.”
Meta’s multibillion-dollar investments in AI infrastructure have come under heavy scrutiny recently. Some AI researchers have questioned whether throwing more data and computing power at LLMs will lead to meaningful progress. Such doubts have gained traction with the recent debut of Chinese startup DeepSeek Ltd.’s DeepSeek R-1 reasoning model, which was reportedly built at a much lower cost, using less advanced GPUs.
The arrival of DeepSeek sparked a big drop in the value of Nvidia’s stock, and the market has since become even more volatile amid broader trade concerns.
THANK YOU