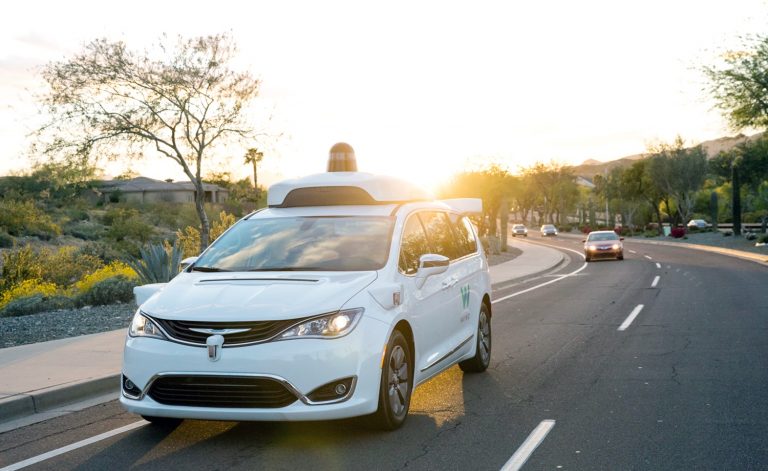
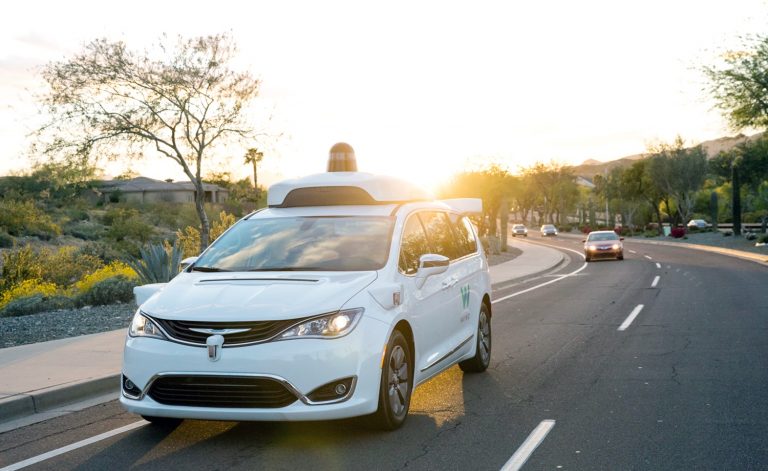
DeepMind, Alphabet Inc.’s artificial intelligence research unit, is assisting sister company Waymo with its efforts in the autonomous vehicle market.
Researchers from DeepMind detailed the collaboration in a post on the group’s blog today. The division has been working with Waymo to harness a technique called population-based training to speed up the development of autonomous driving software.
Originally invented by DeepMind as part of a previous AI project, the method takes cues from evolution to enhance AI models. The process of building a neural network is inherently evolutionary in certain respects. During development, AI models go through training sessions in which they crunch sample data and modify their behavior to produce more accurate results.
But many other essential development tasks still have to be performed with manual methods. Among the most important of these tasks, and the one DeepMind helped Waymo streamline, is hyperparameter exploration. This is the process of fine-tuning the essential behavioral settings that are hard-coded into an AI model. Engineers often set a model’s settings manually or, in complex projects, use a computer-assisted search wherein a script tries out a large number of randomly generated options.
Population-based training makes the workflow much more efficient. The basic concept is to use an automated algorithm to test different setting combinations across a group of AI models, identify underperforming members and replace them with versions of their top-performing peers. The group becomes better at processing data with each such cycle.
“Networks are evaluated periodically and compete with each other for “survival” in an evolutionary fashion,” DeepMind’s researchers detailed. “If a member of the population is underperforming, it’s replaced with the “progeny” of a better performing member. The progeny is a copy of the better performing member, with slightly mutated hyperparameters.”
DeepMind collaborated with Waymo to apply this approach to optimizing the group’s autonomous driving models. In their first joint project, Waymo used the technique to train an AI for detecting pedestrians, cars and other objects near a self-driving vehicle. Population-based training helped reduce the number of false positives that the model produced by 24%.
Waymo went on to apply the technique to other projects as well. Using the method, the group has halved both the time it takes to find an optimal set of settings for an AI and the amount of processing power required to do so. Plus, it saved time for researchers by reducing the need for manual input during development.
“By incorporating PBT [population-based training] directly into Waymo’s technical infrastructure, researchers from across the company can apply this method with the click of a button, and spend less time tuning their learning rates,” the researchers wrote. “Since the completion of these experiments, PBT has been applied to many different Waymo models, and holds a lot of promise for helping to create more capable vehicles for the road.”
Support our open free content by sharing and engaging with our content and community.
Where Technology Leaders Connect, Share Intelligence & Create Opportunities
SiliconANGLE Media is a recognized leader in digital media innovation serving innovative audiences and brands, bringing together cutting-edge technology, influential content, strategic insights and real-time audience engagement. As the parent company of SiliconANGLE, theCUBE Network, theCUBE Research, CUBE365, theCUBE AI and theCUBE SuperStudios — such as those established in Silicon Valley and the New York Stock Exchange (NYSE) — SiliconANGLE Media operates at the intersection of media, technology, and AI. .
Founded by tech visionaries John Furrier and Dave Vellante, SiliconANGLE Media has built a powerful ecosystem of industry-leading digital media brands, with a reach of 15+ million elite tech professionals. The company’s new, proprietary theCUBE AI Video cloud is breaking ground in audience interaction, leveraging theCUBEai.com neural network to help technology companies make data-driven decisions and stay at the forefront of industry conversations.