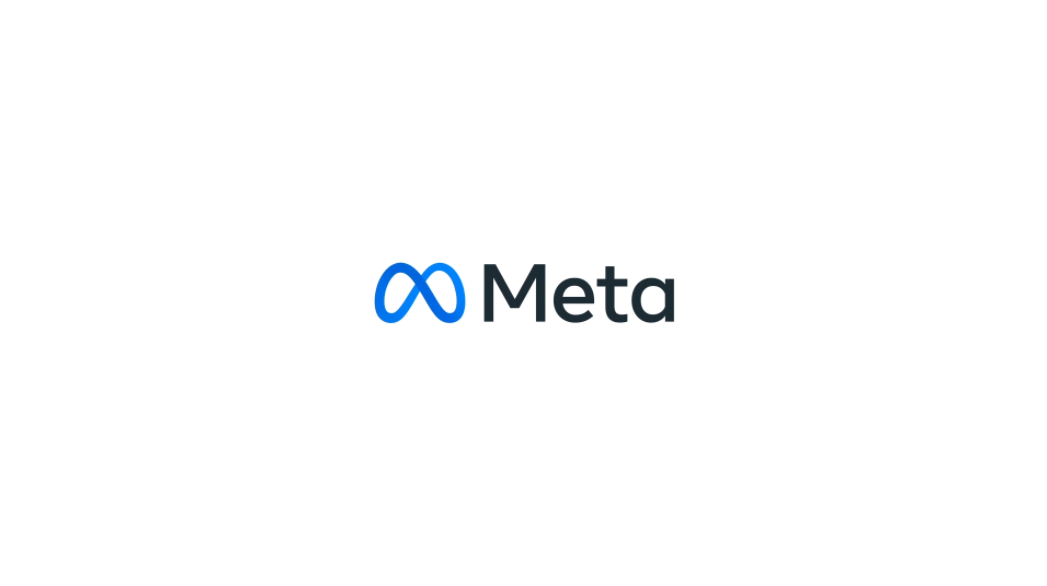
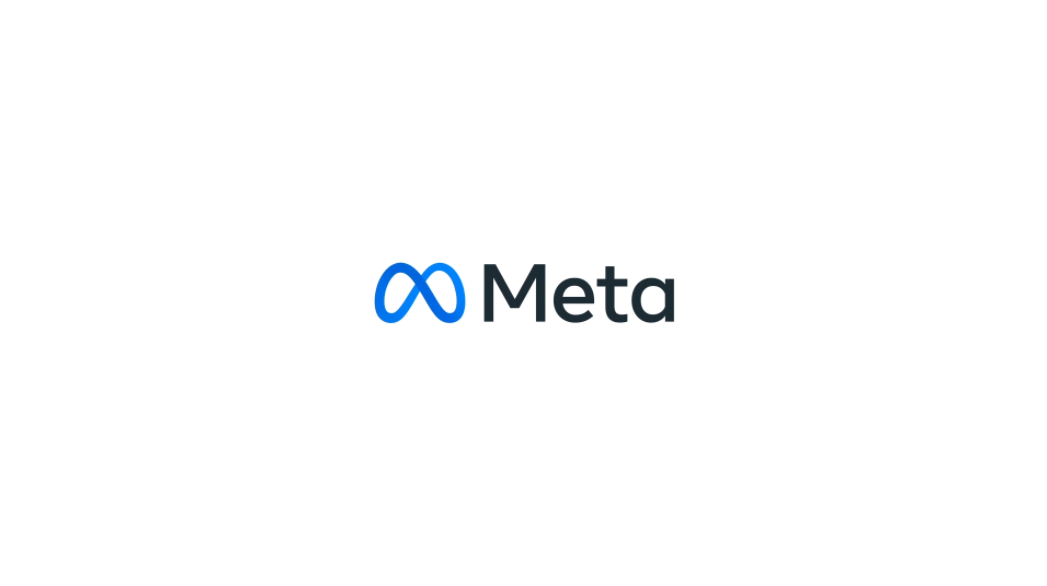
Meta Platforms Inc. today released FACET, a benchmark dataset designed to help researchers audit computer vision models for bias.
The company is rolling out FACET alongside an update to its open-source DINOv2 toolkit. Originally introduced in April, DINOv2 is a collection of artificial intelligence models designed to ease computer vision projects. Thanks to today’s update, DINOv2 is now available under a license that permits commercial use.
Meta’s new FACET dataset is designed to help researchers determine if a computer vision model is generating biased results. In a blog post, the company detailed that evaluating AI fairness is difficult using current benchmarking methods. According to Meta, FACET will ease the task by providing a large evaluation dataset that researchers can use to audit several different types of computer vision models.
“The dataset is made up of 32,000 images containing 50,000 people, labeled by expert human annotators for demographic attributes (e.g., perceived gender presentation, perceived age group), additional physical attributes (e.g., perceived skin tone, hairstyle) and person-related classes (e.g., basketball player, doctor),” Meta researchers detailed in the blog post. “FACET also contains person, hair, and clothing labels for 69,000 masks from SA-1B.”
Researchers can check a computer vision model for fairness issues by having it process the photos in FACET. From there, they can run an analysis to determine if the accuracy of the model’s results varies across photos. Such accuracy variations may be a sign that the AI is biased.
Meta says FACET can be used to find fairness issues across four types of computer vision models.
Researchers can use the dataset to detect bias in neural networks optimized for classification, which is the task of grouping together similar images. Additionally, it makes it easier to evaluate object detection models. Such models are designed to automatically detect items of interest in photos.
FACET also lends itself to auditing AI applications that perform instance segmentation and visual grounding, two specialized object detection tasks. Instance segmentation is the process of visually highlighting items of interest in a photo, such as by drawing a box around them. Visual grounding models, in turn, are neural networks that can scan a photo for an object that a user describes in natural language terms.
“While FACET is for research evaluation purposes only and cannot be used for training, we’re releasing the dataset and a dataset explorer with the intention that FACET can become a standard fairness evaluation benchmark for computer vision models,” Meta’s researchers wrote.
Alongside the release of FACET, Meta today switched its DINOv2 series of open-source computer vision models to an Apache 2.0 license. The license allows developers to use the software for both research and commercial projects.
Meta’s DINOv2 models are designed to extract data points of interest from images. According to Meta, engineers can use the extracted data to train other computer vision models. Meta says that DINOv2 is significantly more accurate than a previous-generation neural network it developed for the same task in 2021.
Besides changing DINOv2’s license, Meta today also added two new models to the toolkit. The first is designed to estimate the distance of the objects in a photo from the camera. The other addition, in turn, is a so-called semantic segmentation model that can break up an image into sections and describe each one.
THANK YOU