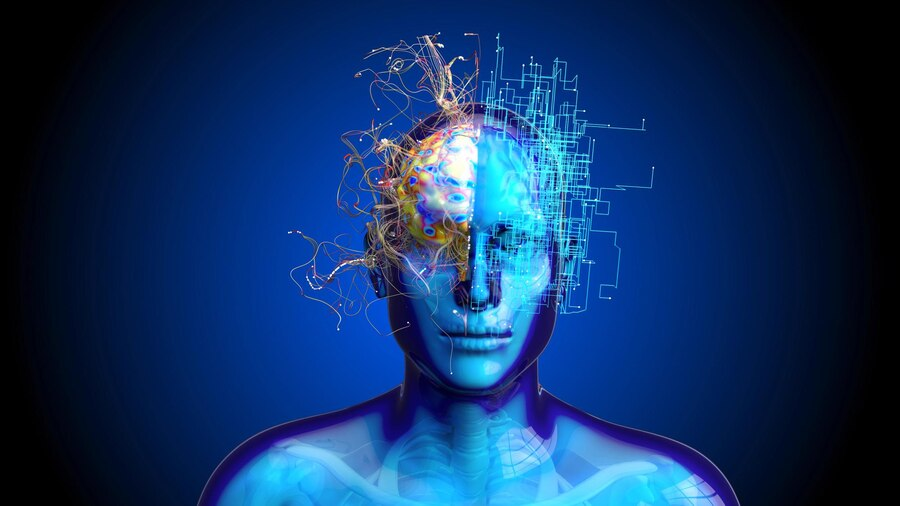
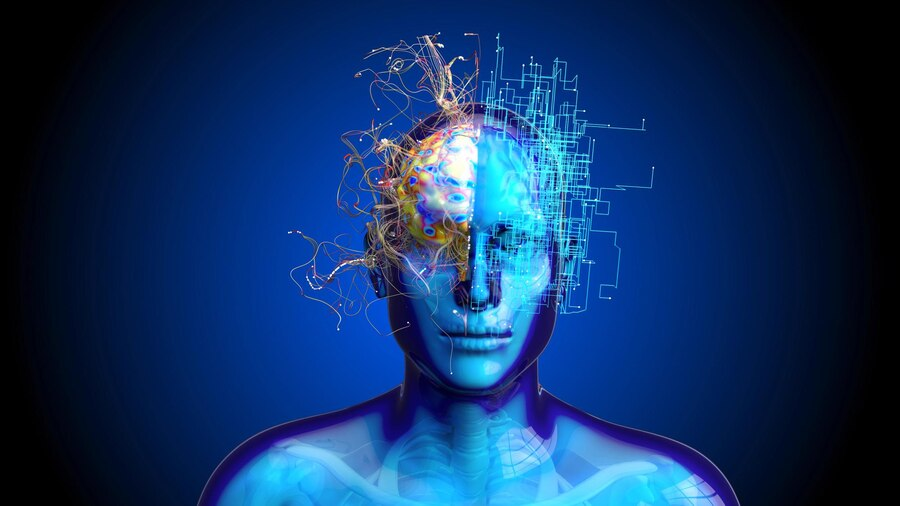
Artificial intelligence startup Anthropic PBC says it has come up with a way to get a better understanding of the behavior of the neural networks that power its AI algorithms.
The research could have big implications for the safety and reliability of next-generation AI, giving researchers and developers greater control over the actions their models take. Outlined in a blog post last week, it looks at the unpredictability of neural networks, which are inspired by the human brain, mimicking the way that biological neurons signal to one another.
Because neural networks are trained on data, and not programmed to follow any rules, they produce AI models that can display a dizzying array of behaviors. Although the math behind these neural networks is well-understood, researchers have little idea why the mathematical operations they perform result in certain behaviors. This means it’s very difficult to control AI models and prevent so-called “hallucinations,” where AI models sometimes generate fake answers.
Anthropic explains that neuroscientists face a similar challenge in trying to understand the biological basis for human behavior. They know that the neurons firing in a person’s brain must somehow implement their thoughts, feelings and decision-making, but they can’t identify how it all works.
“Individual neurons do not have consistent relationships to network behavior,” Anthropic explained. “For example, a single neuron in a small language model is active in many unrelated contexts, including: academic citations, English dialogue, HTTP requests, and Korean text. In a classic vision model, a single neuron responds to faces of cats and fronts of cars. The activation of one neuron can mean different things in different contexts.”
To get a better grasp of what neural networks are doing, Anthropic’s researchers looked deeper at the individual neurons and identified what it says are small units, known as features, within each neuron that better correspond to patterns of neuron activations. By studying these individual features, the researchers believe, they can finally get a grip on how neural networks behave.
In an experiment, Anthropic studied a small transformer language model, decomposing 512 artificial neurons into more than 4,000 features that represent contexts such as DNA sequences, legal language, HTTP requests, Hebrew text, nutrition statements and more. They found that the behavior of the individual features was significantly more interpretable than that of the neurons.
To validate its research, Anthropic created a blinded human evaluator (below) to compare the interpretability of individual features and neurons. The features (red) have much higher scores than the neurons (teal).
This, Anthropic says, provides strong evidence that features can be used as a basis for neural network understanding. By zooming out and looking at the entire set of 4,000-plus features, Anthropic discovered that each one is largely universal across different AI models. As such, the lessons learned by studying the features in one model can be applied to others.
Holger Mueller of Constellation Research Inc. said neural networks are the key development that has made AI become so powerful in the last few years. Yet no one really fully understands how they work, and so it’s impossible to predict how they will answer or respond to any given prompt, he said.
Mueller added that there are even instances where neural networks have generated different answers when fed the same data, confounding even the brightest AI experts. To date, most efforts to understand AI have focused on “mechanistic interoperability,” which is the study of reverse-engineering neural networks.
“It’s in this light that Anthropic has come up with a very interesting approach to solving this confusion with its higher construct of neuron features, and it has shown their ability to predict the outcomes of neural networks by understanding their inner workings more clearly,” Mueller said. “The approach has not yet been scaled, and it will be interesting to see how well it works when applied to much larger models. But it has shown remarkable promise for smaller models, enabling good progress in mechanistic interoperability.”
With additional research, Anthropic believes that it may be able to manipulate the features it describes to control the behavior of neural networks in a more predictable way. Ultimately, it could prove critical in overcoming the challenge of understanding why language models behave as they do.
“We hope this will eventually enable us to monitor and steer model behavior from the inside, improving the safety and reliability essential for enterprise and societal adoption,” the researchers said.
THANK YOU