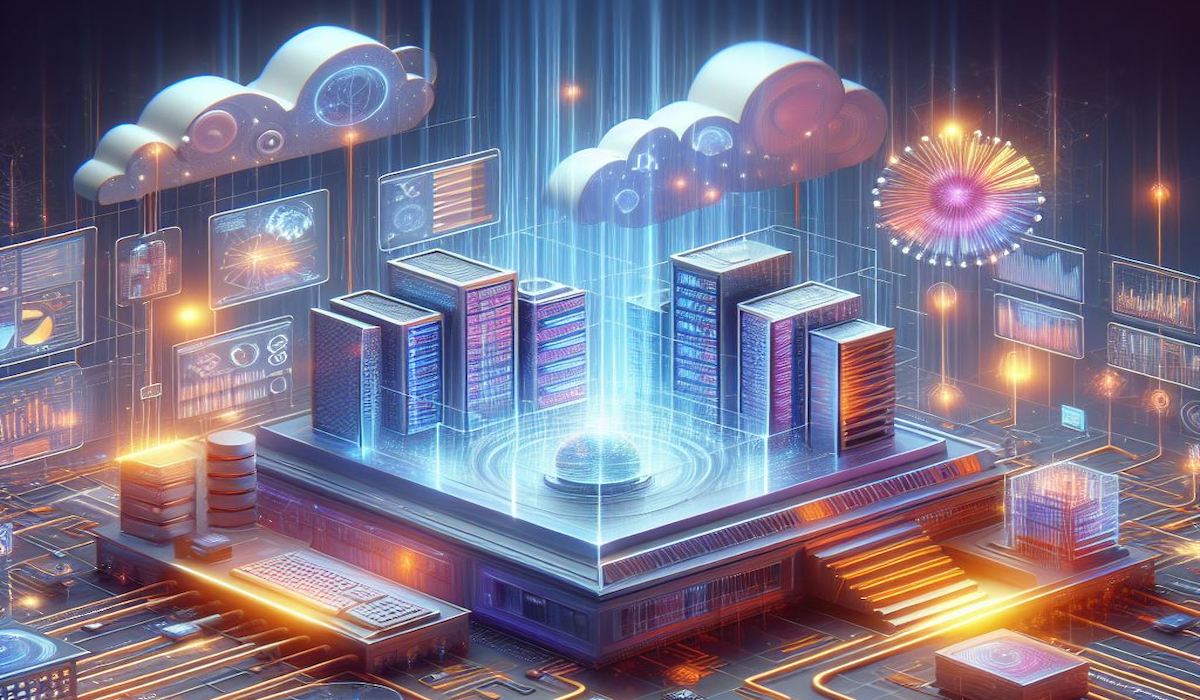
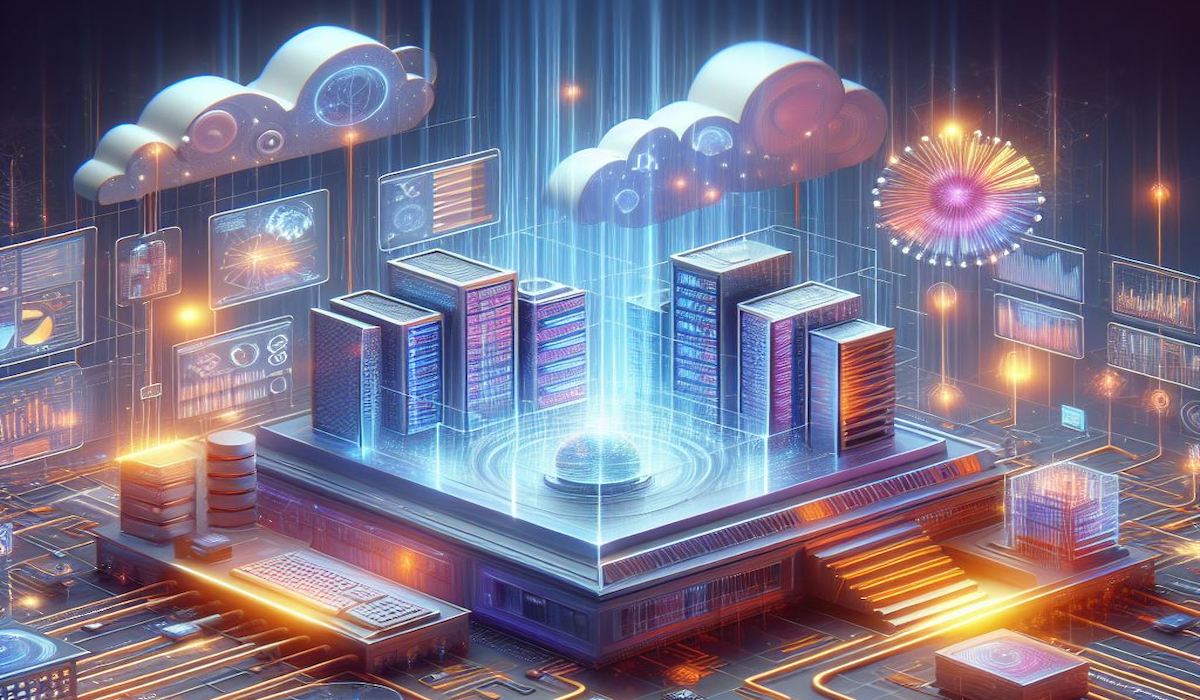
Generative artificial intelligence disrupted the enterprise in 2023 and is now a must-have consideration in 2024 plans.
With this in mind, technical professionals must strive to enhance and modernize their data management platforms to support gen AI to achieve greater business outcomes. Gen AI relies on AI-ready data to function.
However, because gen AI has enterprise-wide implications, modernization often leads to intricate and complex deployment scenarios. Technical professionals are being asked to deliver frictionless data management and to seamlessly integrate with architectures spanning diverse environments, including on-premises, hybrid, edge and cloud systems.
The traditional solutions that technical professionals have previously used might not handle the velocity, variety and volume of data generated by modern application and analytical workloads. Because of gen AI, they must adopt new and recent approaches to data storage and delivery. These approaches can influence a wide variety of data ecosystems, including data lakes, warehouses and hubs.
With the rise of gen AI, technical professionals should feel empowered to bring efficiency and effectiveness to data management practices. Here are four key trends for data management that technical professionals should consider as they look to deliver effective data management solutions and enhance their data architecture with gen AI in mind:
A cohesive data management environment supports all kinds of data workloads and is accessible via unified access with augmented and integrated capabilities. These include advancing gen AI capabilities with a robust framework for common governance and metadata management.
Data and analytics architectures are evolving rapidly due to gen AI, forcing technical professionals to adapt their current strategies to accommodate numerous deployment structures. To succeed, organizations must design adaptable data architecture that seamlessly integrates, enables collaboration and delivers complementary analytics solutions.
Based on this trend, technical professionals should focus their 2024 data architecture efforts on the following activities:
Data storage choices are continuously evolving — even more so because of gen AI — increasing the importance of using multiple data storage and processing engines to maximize cost advantages in both on-premises and cloud environments.
With data usually distributed across multiple systems, both inside and outside the enterprise, it remains difficult to identify and integrate.
Data also needs to evolve as new digital scenarios emerge. The burden of administering infrastructure makes it harder for technical professionals to deliver solutions that keep up with the changing business needs of gen AI. By deploying fully managed platforms, technical professionals can focus on delivering business values instead of mundane database administration tasks, as well as maintenance tasks like backup, patching and scaling.
Technical professionals should focus their 2024 data storage deployment efforts on the following activities:
With gen AI, technical professionals should consider data issues as an engineering problem and strive to empower data engineers to provide accurate and reliable data to consumers and applications within the expected time frames. This should drive data engineers to be involved in various efforts to replatform their data, as data management platforms evolve rapidly, which include architecting, designing and migrating data integration pipelines.
As they architect, design and develop these data integration pipelines, technical professionals need to address a number of challenges. Agility, a data-centric approach for development, and a framework-agnostic solution to define scalability and latency requirement, are just some of the challenges they may face.
Given these challenges, technical professionals should focus their 2024 data integration efforts on the following activities:
Some practices and technologies, including gen AI, are so far-reaching that they influence many aspects of contemporary business and technology. Its influences can be felt at all phases of the data life cycle, and at all layers of a typical application stack. The influences can alter best practices, desirable design patterns, the scope and coordination of various aspects of governance, and the management of fundamental forms of data that are used everywhere.
With the headlong rush to capitalize gen AI techniques, there is an enthusiasm — and the fear of being left behind by competitors — that entices many organizations to pursue gen AI heedlessly.
Sensible organizations will proceed with caution. They should proceed with gen AI only with the official approval of strategic leadership, and only with formalized processes for governing gen AI usage. Remember that some vendor products include features powered by gen AI; use of such features should also be governed accordingly.
To appreciate the far-reaching nature of gen AI, technical professionals should:
Technical professionals should address these four trends to develop their data architecture for use by gen AI in the long term. It is imperative that they take a holistic look at this architecture from an enterprise perspective so that they can address any scenario that may arise.
Ramke Ramakrishnan is a senior director analyst at Gartner Inc., with broad-based experience in driving data management strategies and delivering excellence through research, innovation, advisory and agility for technology professionals. He wrote this article for SiliconANGLE. Gartner analysts will provide additional analysis on emerging technologies, including gen AI, at the Gartner Data & Analytics Summit, taking place March 11-13, in Orlando, Fla.
THANK YOU