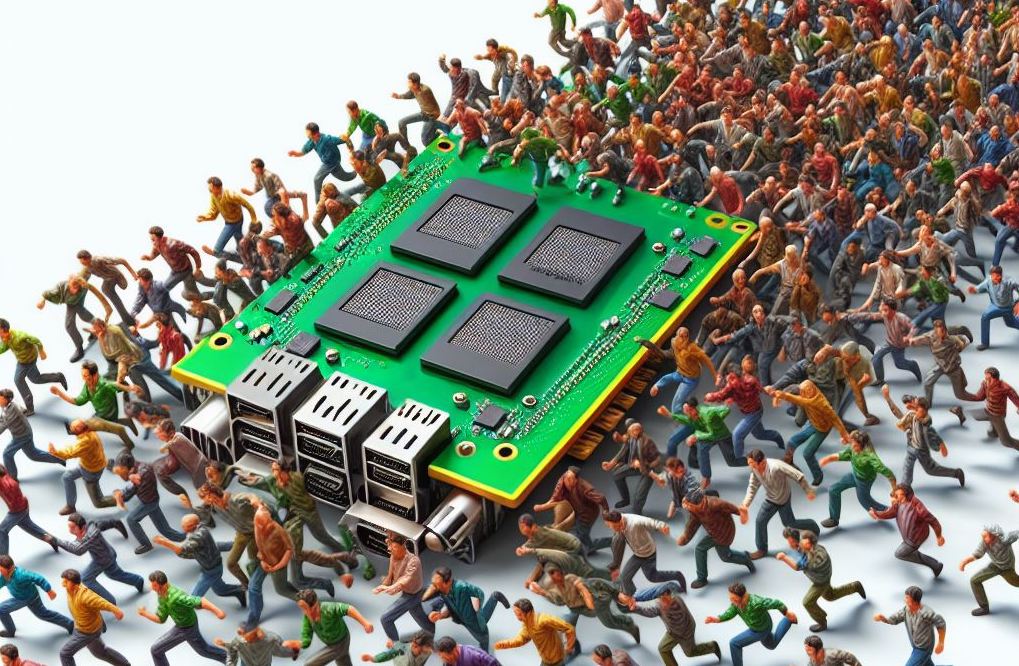
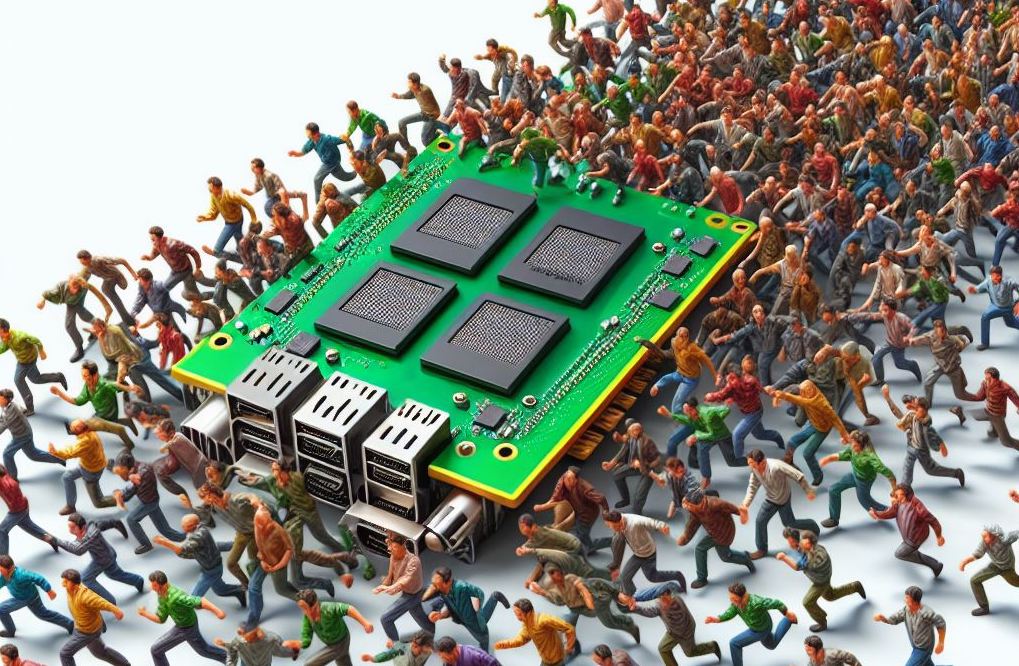
This is the first of two parts. Part two is here.
It’s little mystery that generative artificial intelligence has dominated the spotlight in tech over the past year. A couple of KPMG surveys of top U.S. business executives conducted in March and June of last year document what has become conventional wisdom: Gen AI is being seen as the top emerging technology over the next three to five years. And with it comes steep expectations for economic impact.
A McKinsey forecast estimates that gen AI could conservatively add up to $4.4 trillion annual value to the global economy, which is roughly equivalent to current annual global information technology spending, according to Gartner and Forrester. Goldman Sachs pins the jump to global GDP at 7% over the next decade, which comes out to about $7 trillion.
Forget about the fact that gen AI lets us finally talk to computers the way Captain James T. Kirk did on the Starship Enterprise. That has gotten our attention. Could the sleeper be that gen AI will actually drive real return on investment from technology? And how soon will that ROI materialize?
A Harris poll conducted for consulting firm Insight Enterprises revealed two-thirds (66%) of business leaders reporting already using gen AI within their organizations, with just over half citing productivity as the prime benefits. Where are the main use cases? According to the poll, it’s mostly from visualization for data analysis, copilots for summarizing email and other documents, and, probably least surprising, generation of content (full disclosure: this report was written by a real person). A curious omission, however, is that the survey overlooked one of the most discussed hotspots for gen AI: copilots for code generation.
But let’s get real. Like any technology, the full impacts of gen AI will take time. For instance, enterprise adoption of internet and web technologies came in stages, starting with web browser clients to internal applications and bare-bones informational websites for external customers or business partners. It took time for the web to become transactional, dynamic and intelligent. And it took several years after consumers adopted the iPhone for mobile and edge apps to penetrate the enterprise.
There will be quick hits for gen AI, such as adding natural language query, document entity extraction and summarization, marketing content generation, enhancements to existing enterprise applications and so on. Dig deeper, however, and the transformational potential of gen AI for changing how organizations conduct business is going to take some time.
Spoiler alert: There will eventually be a light at the end of the rainbow. In the short run, however, gen AI is going to be a huge cost drain. It will require deep upfront investments well before the industry starts making real return and enterprises see real transformation.
First, let’s look at how much enterprises are likely to spend. The Economist is quite bullish, predicting 2024 will be the year when enterprise adoption in gen AI will take off, imputing that the experimental phase is already winding down.
We’re a lot less sanguine.
In the short term, most organizations are not going to pay a lot for this muffler. According to a Deloitte study, 70% of companies are testing the waters with gen AI, but fewer than 20% of them are willing to pony up new budget for it. A survey from Enterprise Technology Research is slightly more optimistic, with just over half of companies expecting to add budget for gen AI.
In terms of overall impact on IT spend, in the short run, gen AI should be a drop in the bucket. Deloitte estimates enterprises will spend about $10 billion on gen AI this year, which at first glance sounds impressive. But it’s a drop in the bucket when compared with overall IT spend, which as noted before is estimated between $4 trillion and $5 trillion.
Although in the grand scheme of things, enterprise gen AI investment this year will be quite modest, early adopters lucky enough to grab graphics processing unit capacity will pay dearly for it. That’s because there’s a huge GPU shortage. Nvidia Corp. has been internally discussing how to ration the supply. With H100s reportedly going for up to $30,000 apiece, it’s boom times for Nvidia. With just-reported fourth-quarter earnings triple that of a year ago, CEO Jensen Huang claims that the gen AI has hit a tipping point.
Nvidia’s gain is everybody’s cost. Hyperscalers face huge capital costs for build-out while customers queue up for rare, precious, and expensive capacity. For instance, OpenAI had to commandeer 20,000 Nvidia GPUs on Azure to support ChatGPT when it was first unleashed on the public. It can afford it, given that the company’s valuation has tripled to $80 billion in less than a year.
Here are a few more numbers to make you dizzy. During the fourth quarter of calendar 2023, Alphabet Inc. spent $11 billion in capital expenditures, with Microsoft Corp. not far behind at $9.7 billion. Extrapolate those figures for the whole of 2024 and you’ll get numbers that exceed the gross national products of countries such as Bahrain.
Admittedly, at first glance it appears that AI will only be a fraction of overall capital costs as much of it is toward ongoing opening of more hyperscaler data centers. Nonetheless, AI-related investments are a significant chunk of capital for a technology that will take some time to amortize.
Nonetheless, for anybody serious about developing or implementing gen AI this year, such as vendors and early enterprise adopters, they will likely have to pony up for at least one to two years of GPU capacity commits to get to the head of the line. Although it’s not likely that most customers are likely to hit critical mass 80%-plus capacity utilization on their GPU instances anytime soon, spot markets for unused cycles are still embryonic.
The GPU compute shortage is redolent of the early days of mainframes when corporations had to buy bigger boxes just to ensure adequate just-in-case capacity. And that’s before IBM Corp. introduced capacity-on-demand, where it sold you a bigger box but only charged for processors that were turned on, a solution that didn’t necessarily solve the utilization problem but kicked it down the road.
For born-in-the-cloud tech startups, this flips the script of the past 10 to 15 years. The cloud lowered the barriers to entry for startups in several important ways. It enabled startups to avoid having to buy their own server infrastructure for developing and testing their own products. And if they stayed cloud-native and delivered their software as a service, they avoided the overhead of distributing software and holding customers’ hands for routine version updates.
However, for startups tackling gen AI, the extreme costs of training language models has changed the equation. Published reports show larger startups such as Anthropic PBC spending almost half their monthly revenue on training, with high startup costs prompting some ventures to look for early exits.
Nonetheless, for venture capitalists, we expect irrational exuberance to prevail because of the greater fear of missing out. There’s always money in The Valley. And, as we’ll note in Part II tomorrow, with the learning curve, there will be a longer tail of narrower models that may cut the number of training parameters by at least factors of ten.
The laws of supply and demand have got to bring back sanity at some point. Nature, and the market, abhor a vacuum, so where there’s demand, suppliers are going to rush in. Advanced Micro Devices Inc., Intel Corp., hyperscalers and others are antsy to grab part of the pie, with Amazon Web Services and Google Cloud having the advantage of already having specialized training and inference chips out there. OpenAI CEO Sam Altman is lobbying the United Arab Emirates and others for up to $7 trillion to build a new AI chip supply chain.
Some, such as Databricks Inc. CEO Ali Ghodsi, are more sanguine. He equates the current rush to GPUs with the great bandwidth landgrab of the early 2000s. He confidently predicts that the equation should balance out in as little as a year, implying that enterprises might want to go easy on blocking out so much expensive capacity now.
But there are huge buts to this optimistic scenario. First, there is the dominance of Nvidia’s CUDA libraries that have matured over nearly 20 years. It’s the secret to Nvidia’s home court advantage. These are the toolkits that developers use for exploiting the underlying CUDA programming language and Nvidia parallel computing architecture. Though Nvidia rivals could write emulators for their own chipsets, there will be inevitable performance hits that will be especially noticeable for the types of compute-heavy workloads that characterize gen AI.
Nvidia won’t be the only game in town. On the software side, it will take time for the Intels and AMDs of the world to match the breadth of functions that CUDA set as the de facto standard for the GPU world. Then there’s the question of getting the fabs going to crank out all that silicon. And that’s where Intel’s newly announced foundry business comes in. It’s looking for its leapfrog moment with state-of-the-art lithography machines expected to come online by 2027. TSMC and others won’t stand still either, but again, we’re talking several years for new production to ramp up.
That big sucking sound is the rush of money being poured by technology providers for the promise of returns that are at least 12 to 24 months away. So, where’s the light at the end of the tunnel? You might get some insights from “The Wizard of Oz.” We’ll explain that part of the tale in Part II tomorrow.
Tony Baer is principal at dbInsight LLC, which provides an independent view on the database and analytics technology ecosystem. Baer is an industry expert in extending data management practices, governance and advanced analytics to address the desire of enterprises to generate meaningful value from data-driven transformation. He wrote this article for SiliconANGLE.
THANK YOU