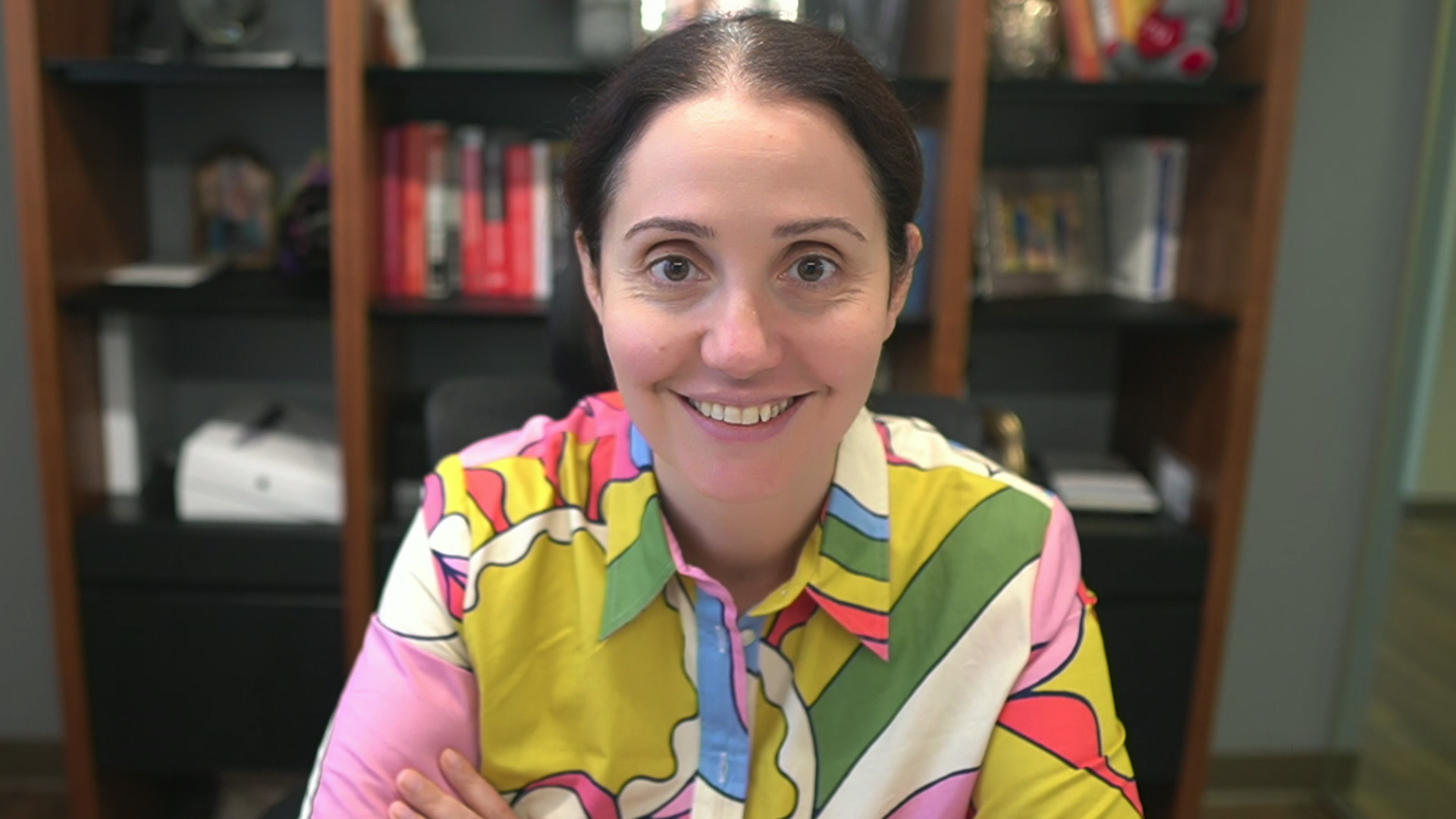
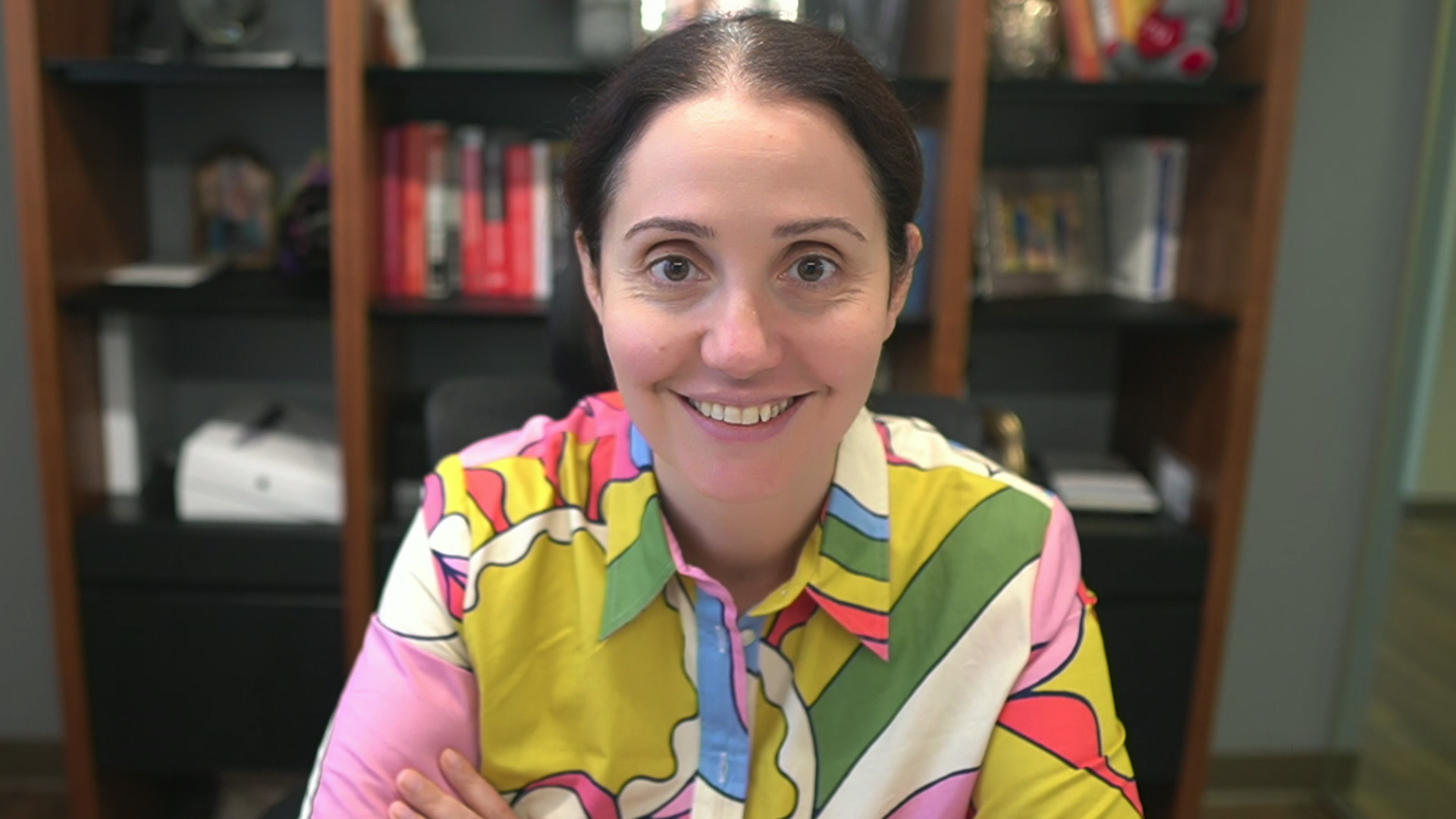
In recent months, there’s been a surge of interest in data platforms, new artificial intelligence models and the platforms they run on. But for those who have been invested in AI for long periods, some lessons often go missed, including when it comes to AI data foundations.
There’s a fascination at play with large language models. But AI is often viewed in the wrong way, according to Rudina Seseri (pictured), founder and managing partner at Glasswing Ventures.
“I think AI is being viewed, largely driven by a lot of mindshare and newsshare, as a monolithic notion, ‘I have an AI company, I have an AI fund,’ and I think it gets lost in that noise,” Seseri said. “The notion that AI is an output, meaning that it’s delivering human-like intelligence by software or software and algorithms wrapped in a can, as I like to say, which is typically a device of sorts. That is not the how of it, that’s the what and the output.”
Seseri spoke with theCUBE Research’s Paul Gillin at the Supercloud 7: Get Ready for the Next Data Platform event, during an exclusive broadcast on theCUBE, SiliconANGLE Media’s livestreaming studio. They discussed the complexities of AI development and the importance of AI data foundations.
When understanding how AI is viewed incorrectly, there’s much to consider. That includes understanding the “how” of the situation, according to Seseri.
“If we use really neural nets and deep learning, and more broadly, machine learning as the proxy for the how, it’s only about 80% correct to do so,” she said. “But for the purposes of this discussion, I think it’s good enough, an understanding that there are literally hundreds of architectures and subarchitectures, thousands of techniques that need to be leveraged or can be leveraged to deliver the intelligence or the output of AI. And they’re extremely varied in nature.”
Often, one can combine architectures and techniques in ensemble models. But saying AI is like saying technology, and if one really wants to understand, they must go deeper, according to Seseri.
“In fact, at Glasswing, we have created a framework. We refer to it as the AI palette, where literally we have mapped just some of the major architectures and subarchitectures and techniques and training approaches, and then match them with the data sets and the use cases,” she said. “Developing AI is quite complex, and what goes into it is largely varied.”
When considering generative AI in the context of the enablers, it involves transformer and attention technologies and methods, according to Seseri. Aside from that, there’s also architecture called recurrent neural nets.
“It is a type of deep learning architecture, and basically what you can do is train it so you can move the data bidirectionally,” she said. “It has memory. It’s very, very powerful. Within it you have subarchitectures and techniques like PINNs, one of my favorites, which stands for physics-informed neural networks, where you can fundamentally bring the boundaries of the physical world to the algorithms to mirror and simulate the physical world, with incredible ramifications.”
As enterprise CIOs increasingly view AI as a priority item for them moving forward, a chief area of concern involves data quality, data duplication and data proliferation throughout the enterprise. It’s a problem, but a solvable one, and organizations must consider AI data foundations, according to Seseri.
“There are multitudes of possibilities around algorithmic and machine learning-type approaches that one can take. The real key is the data,” she said. “At Glasswing, we’re thesis-driven. One of our theses is the data foundations. We’re not focusing on the foundational models, we’re focusing on the data foundations.”
What that means is how to make data cheaper, better and faster. For a CIO, data quality is one big component of managing data and ensuring that it’s high-quality data and high-quality output, according to Seseri.
Large language models, of course, are not the end-all, be-all of generative AI. Small language models also have a role to play.
“Having your own small language model is quite powerful, because what it does, it takes what is an otherwise general model and really gives you the specificity of output and performance that one needs, especially if one is taking a vertical approach or solving very, very specific problems, but for large market opportunities,” Seseri said. “If one was to be differentiated beyond even fine-tuning, I think small language models are a very viable path to do so.”
Here’s the complete video interview, part of SiliconANGLE’s and theCUBE Research’s coverage of the Supercloud 7: Get Ready for the Next Data Platform event:
THANK YOU