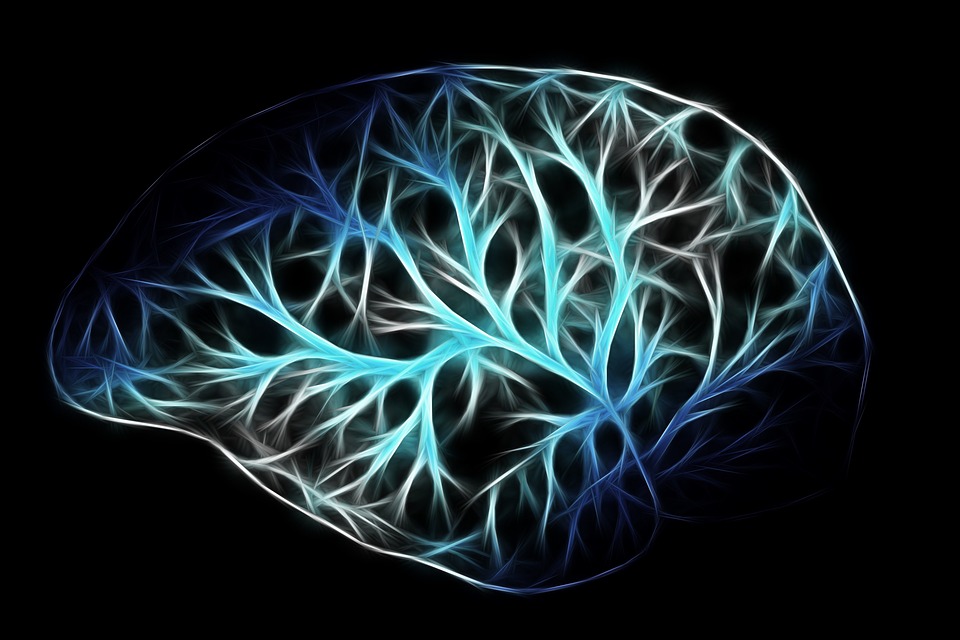
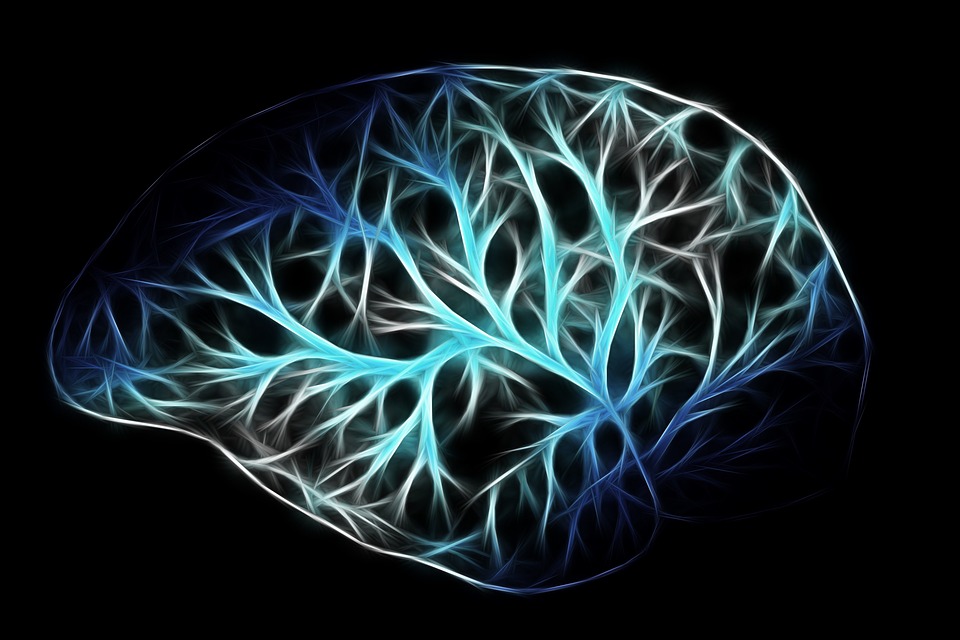
Many of the recent breakthroughs made in artificial intelligence, such as facial recognition and natural language processing systems, simply wouldn’t be possible without the help of neural networks.
Neural networks refer to the tightly bound connections of processors that can ingest massive amounts of data and make sense of it. But the growth of these neural networks is held back by their reliance on powerful hardware that can only be housed in remote data centers.
This hardware can still be accessed by almost any device, with the data being uploaded to distant servers, processed and sent back to the device. This is necessary because neural networks tend to be extremely power-hungry, making them unsuitable for low-power smartphones and other devices, such as smart speakers or industrial sensors. The downside, of course, is it takes time to stream data back and forth to the cloud, which means that neural networks are impractical for many of these applications.
Now, researchers at the Massachusetts Institute of Technology say they can address this problem with a new kind of chip that can perform all of the required data processing on site. The researchers claimed Tuesday their new chip is 95 percent more energy efficient than standard processors, and can be incorporated into small battery-powered devices to perform calculations right at the network edge.
In order to achieve this huge energy reduction, Avishek Biswas, an MIT graduate student in electrical engineering and computer science, who led the new chip’s development, manipulated a feature in neural network processing known as the “dot product.”
“The general processor model is that there is a memory in some part of the chip, and there is a processor in another part of the chip, and you move data back and forth between them when you want to do these computations,” Biswas explained. This movement of data back and forth is the main reason why neural networks are so power intensive, Biswas said.
“But the computation these algorithms do can be simplified to one specific operation, called the dot product,” he said. “Our approach was, can we implement this dot-product functionality inside the memory so you don’t need to transfer this data back and forth?”
Biswas and his colleagues achieved that by building a processor that mimics the human brain more faithfully than earlier designs. The prototype chip can calculate 16 dot products simultaneously, while losing just 2 to 3 percent of its accuracy compared with traditional neural networks.
Dario Gil, vice president of artificial intelligence at IBM Corp., who was involved in the project, said the results of the experiment “certainly will open the possibility to employ more complex convolutional neural networks for image and video classifications in the ‘internet of things’ in the future.”
THANK YOU