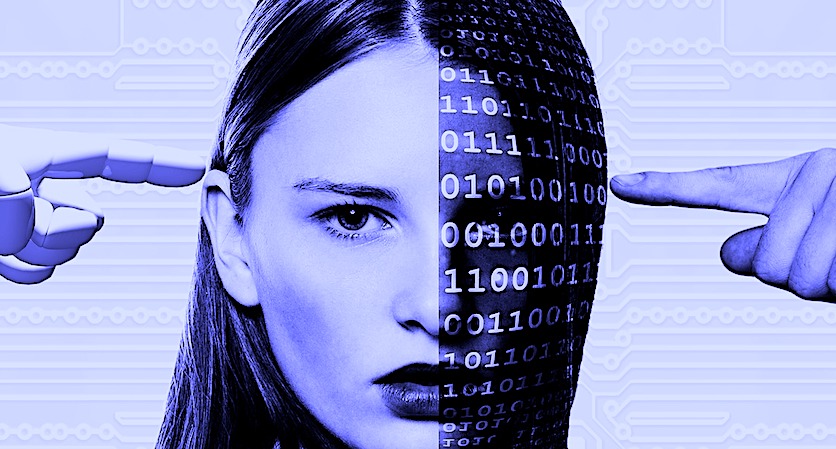
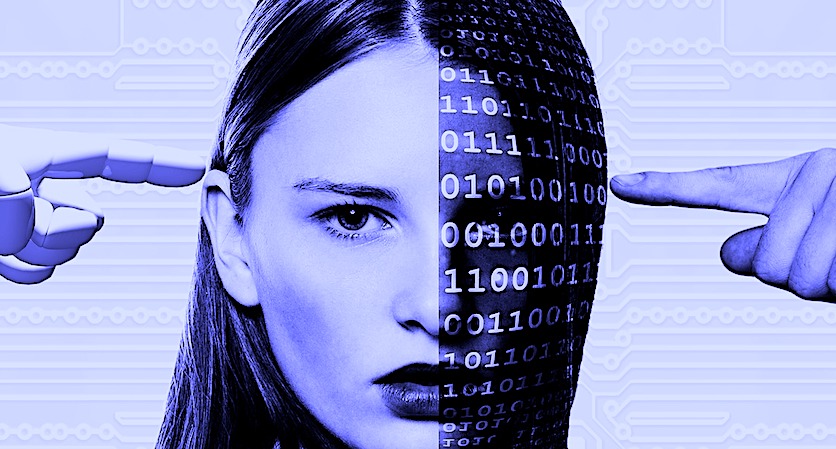
In 2018, there is finally sufficient computing, storage and network resources, as well as tools and skills for creating complex models that mimic reality to the extent that they can stand in for actual objects, processes and even people. These “Digital Twins” are computer-based models originally designed to simulate physical objects for the purpose of monitoring, anticipating issues and testing.
The discipline has now extended to mimic simulations, including those of human beings. The only impediment to devising these tools is the skill of humans to understand, in detail, how the subject operates. As the late British statistician George Box opined, “All models are wrong. Some are useful.”
Digital Twins, a concept from the “industrial internet of things” or IIoT, is the discipline of devising highly capable simulation models, especially those that consume streaming data from sensors to anticipate maintenance issues, impending failure of components and improving performance. In terms of the degree of difficulty of modeling, Digital Twins for IIoT machines such as jet engines, oil wells and wind turbines are actually at the lower end as they employ detailed knowledge of the engineering of the objects. These Digital Twins may be quite complex, but because they are well-understood, the models are more likely to be useful.
Devising a simulation model of human behavior, such as the classical propensity models, is much more difficult because humans are so unpredictable and engineering approaches obviously don’t apply. In effect, these models may do a good job of predicting propensity based many criteria, but at any given moment for any given individual, their predictive capability is quite low.
If these two types of models aren’t challenging enough, consider applying the concepts and techniques of Digital Twins for modeling an individual’s molecular/genomic makeup, physiological characteristics and even behavioral and lifestyle factors as a way to practice “personalized medicine,” an emerging practice in healthcare that treats patients as individuals, not according to some “norm” or “Standard of Care.” Just for clarity, this is much different from “precision medicine,” where doctors and researchers predict more accurately which treatment and prevention strategies for a particular disease will work in which groups of people. Though it is in contrast to a one-size-fits-all approach, which applies to the population, not an individual, it is still not “personalized.”
Digital Twin technology for modeling individual people, or “personalized medicine,” is a concept for simulating the whole human through genomics, physiology and environments/lifestyle over time. However, it is infinitely more complicated to develop a working Digital Twin of a human being than that of an engineered object, such as a jet engine or an oil well. Also, sensors can provide streaming information to a Digital Twin of an engineered object, but to provide the same for an individual, such as blood tests and scans, is prohibitively expensive, intrusive and time-consuming. In that way, the analogy of an engineering Digital Twin breaks down.
Although Digital Twins paint a scenario of data-driven healthcare, it can be a double-edged sword. For example, there is the potential of providing a social equalizer through medical enhancements. On the other hand, it can just as well drive inequality leading to discrimination by identifying traits that are not “normal” based on patterns found in a collection of digital twins. This calls for measures that ensure transparency of data usage and derived benefits, as well as data privacy. Privacy is already identified as a concern with the rapid evolution of inexpensive genomics, but patient Digital Twins will add in more aspects of biological and behavioral data that will be much more identifiable as an individual than a mapped genome alone.
Nevertheless, Digital Twins in industrial IoT and human healthcare have many similarities. A Digital Twin in engineering is code that maps as closely as possible to the aspects of the sensed object via the transmission and analysis of data streaming from sensors. The near-real-time stream of data is the key to managing preventative maintenance and even modifying the behavior of the real-world devices and processes.
A “virtual self” of a patient, at least in theory, is not that different from a Digital Twin of a machine and may be useful in addressing “Standard of Care” practices discussions about health care in general. Digital Twins therefore may provide a way to analyze practices in current debates on health care, such as wellness, disease, preventative care and enhancement. In the same way a Digital Twin of a jet engine in flight can analyze and react to inputs, a patient Digital Twin will follow “normal functioning,” “derangement,” “preventative medicine,” “performance optimization” and “enhancement.”
If only. From a purely practical aspect, it is difficult to see how a model with sufficient fidelity is within the grasp of medical science. First of all, human organisms are elaborately complex. Contrary to classical medicine, the body isn’t composed of “systems” or parts that can be modeled separately such as respiratory, immune, digestive and the like. The holistic whole is a gigantic complex of positive and negative feedback mechanisms. In fact, only recently it appeared that mapping the biome was as important as mapping the genome. As medical researcher/theorist T.S. Wiley claims, “The bacteria in your gut run everything and you are just their meat suit.”
Second, agreement on the cause and effect of things is far from universal. As Niels Bohr said many years ago, “Science proceeds one funeral at a time.” Add to that the commercial influence of the medical/pharmaceutical complex, and Digital Twins for medicine could easily devolve into a riot of subjective opinion.
For example, predicting the effect of a drug and its side effects on an individual at a point in time is nearly impossible. Clinical trials of a drug produce a median dosage based on a large sample that is either insufficient or potentially toxic. Doctors often “titrate” to try to get the right levels, a process that is often harmful to the patient. Newly approved, aggressively marketed “blockbuster” drugs very often have disastrous effects because complex multifactorial diseases are devilishly difficult to treat with existing knowledge.
In addition, as many as half the clinical trials reported in medical journals are reversed over time and unfortunately, many papers in the journals are ghost-written by interested parties. Promising drugs and therapies with little commercial potential do not even get trials. The information needed to build a human Digital Twin is, unfortunately, missing and perverted.
There are some bright spots. The viability of picking the correct medication to treat a tumor as of now has been demonstrated. Genotyping a person’s tumor tissue gives pieces of information on which medication will bring about the greatest effect with the least collateral damage. Customized medication likewise offers the guarantee to prompt remission, where infections can be anticipated and along these lines additionally defensively treated. These approaches, though useful and certainly appreciated by the patient, failed to take into consideration how and why the disease manifested itself in the first place. The practice of medicine today in laser-focused on treatment, at the expense of understanding the why. Digital Twin models may offer a glimpse here.
Digital Twin methodologies for personalized medicine will describe and monitor the healthy state of the individual, not merely the medical history of diseases captured in current practice. “Normal” for an individual will compare the current state of genomic, physical and mental aspects with those of a cohort from the population. The real trick will be devising models at high enough resolution to surpass the individual practitioner’s knowledge and insight, which will be extremely troublesome if not infeasible, given the sheer multifaceted nature of the human body and its operations. Digital Twin models will be constantly sustained with a wide range of data across the lifetime of a person.
The Digital Twins concept is fertile ground to dissect theoretical and ethical issues of future health services and human improvement. Individualized high-resolution information of individuals’ genomic, physiological, lifestyle, and dietary propensities can possibly yield better treatment and improvement. Evaluating these models in whole populations provides opportunities to detect patterns and trends of well-being versus illness. However, Digital Twins models may be oblivious to fair-mindedness. The variation between people can be transparent, giving rise to generalization and discrimination. Use of personal biological and lifestyle data will need to be subject to strict governance.
Thinking ahead, if Digital Twin models for individuals do become common practice, there is a concern that the individual will not be able to escape the conclusions of the model. “Medical Tourism,” as we know it today, where people travel among various practitioners to get the therapy they want, may become impossible as the model follows the patient. Practitioners and payers may follow recommendations of the models and limit choices between, for example, therapy versus quality of life, or choices of parents whether or not to subject their children to intrusive therapies.
Any giant leap in technology is not without its risks. But the application of a discipline that designed for engineering purposes must be tested to ensure in doesn’t adversely affect people’s lives, limit their choices and even discriminate against them.
Here’s some further reading from Wikibon Research:
A Guide to Concepts in Digital Twins, by George Gilbert
Effective Approaches to Building IoT Digital Twins: Joint Development with IBM as Case Study, by George Gilbert
What Is a Digital Twin and Why Does the Concept Matter, by George Gilbert
THANK YOU