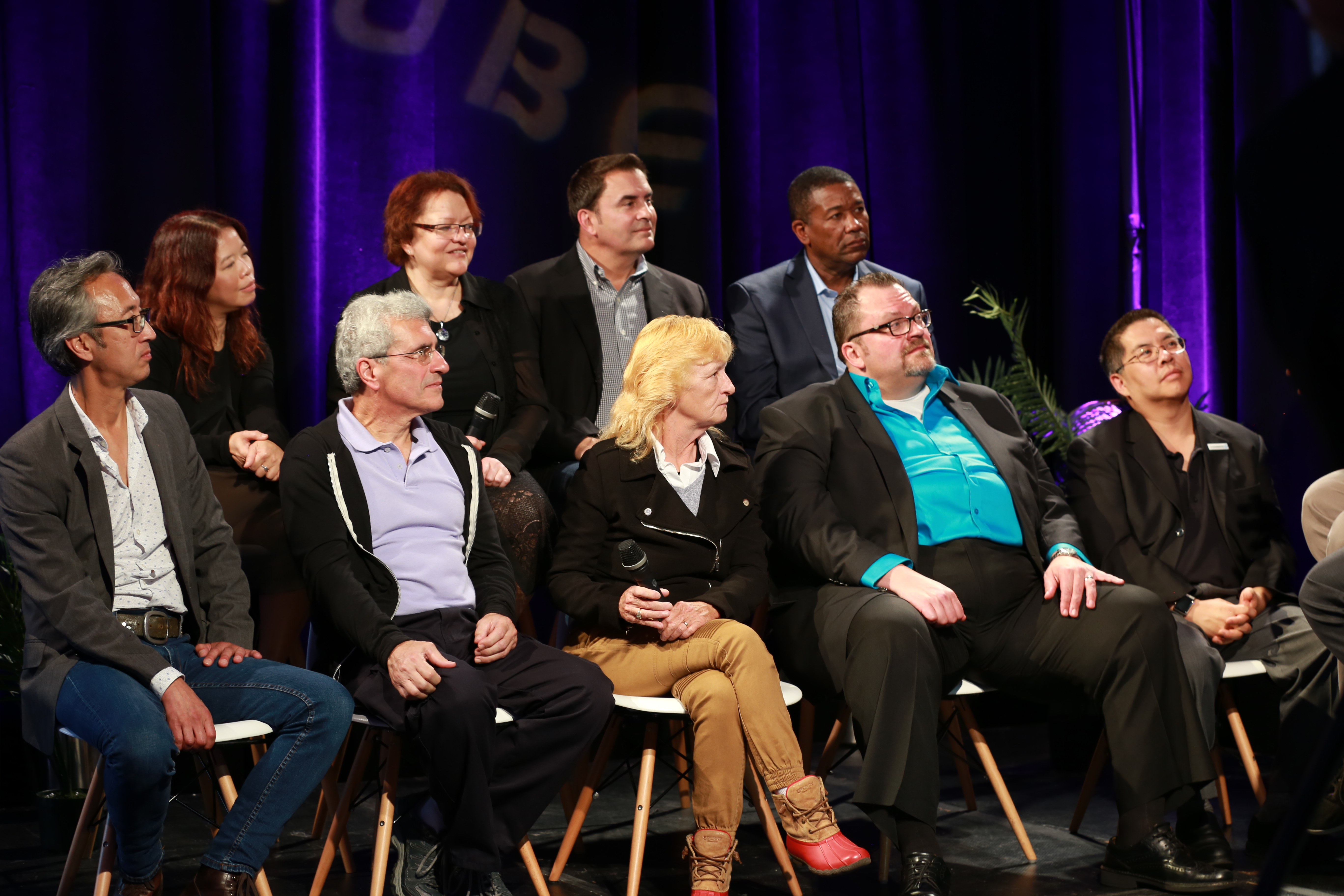
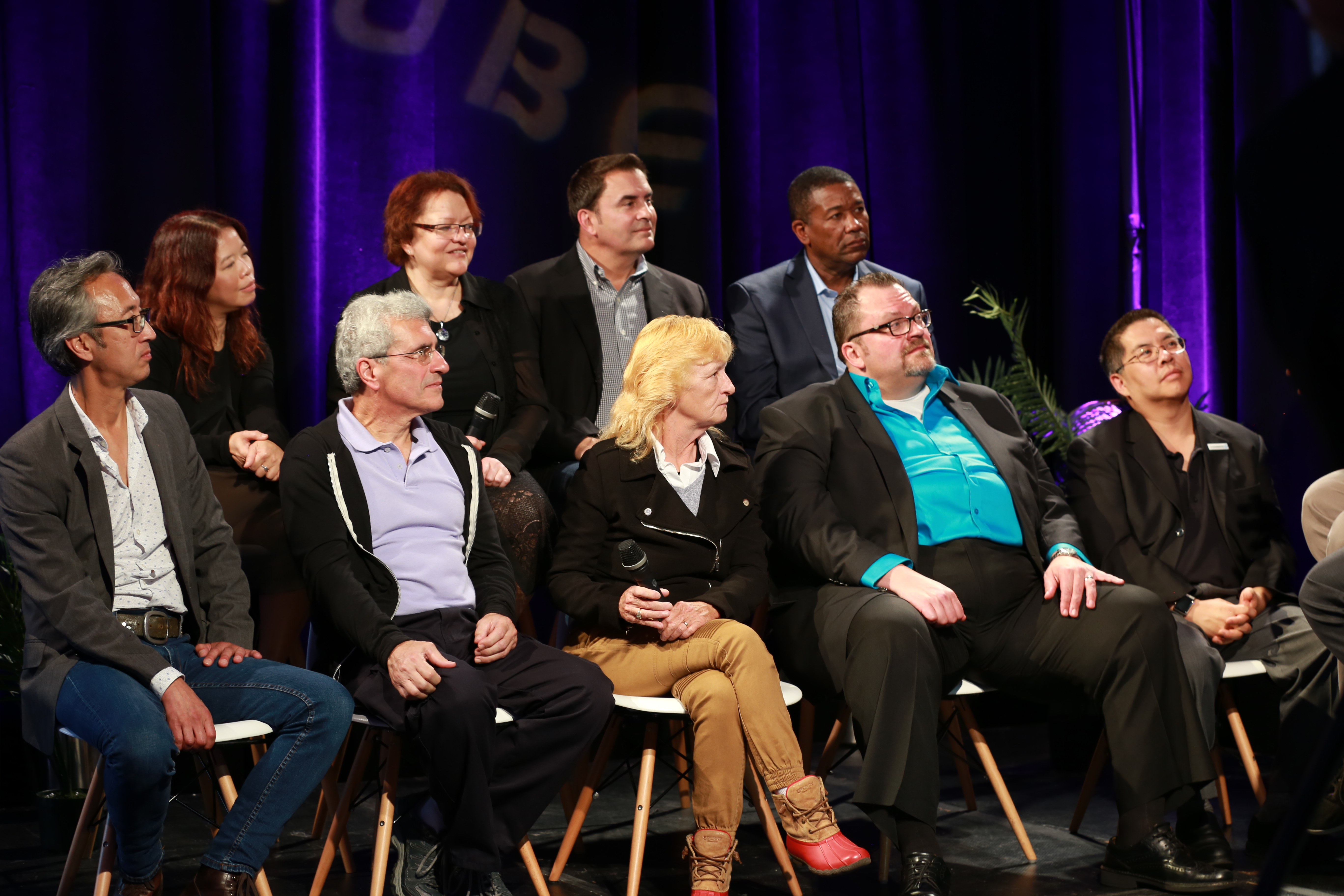
Can we take stock of the state of artificial intelligence for a moment? Vendors are slapping the label on all kinds of products with pretty measly predictive potential. This isn’t just a ripoff; it could have disastrous business and societal impacts if users put too much faith in them. Hypothetically, perfect AI could be trusted to act on behalf of a business or individual. Analysts seem to agree that no one’s brought perfect AI to market yet. What is available can aid human decision making — but users need to know to what extent.
The fact that so few companies are making real profits with big data and AI might cast doubt on the now-stale phrase, “Data is the new oil.” However, it’s actually quite apt, according to Chris Penn, co-founder and chief innovator of Trust Insights.
“I love that expression because it’s completely accurate,” he said. “Crude oil is useless; you have to extract it out of the ground, refine it, and then bring it to distribution.” Refining it requires the work of data scientists, software, training and retraining of models, etc. There people and products exist, but their actual abilities vary widely, he explained.
To choose among the barrel full of tools and thingamajigs for refining it, chief information officers have to assume the role of Inspector Gadget.
“As I attend briefings or demos, everyone is now claiming that their product is AI- or ML-enabled, or blockchain-enabled,” said Karen Lopez, senior project manager and architect of InfoAdvisors, speaking of the revolutionary distributed ledger technology. “And when you try to get answers, what you really find out is that some things are being pushed because they have if/then statements somewhere in their code, and therefore that’s artificial intelligence or machine learning.”
Stretching definitions like saltwater taffy isn’t anything new. As soon as a technology gets hot, vendors will start stamping the word on anything that even slightly resembles the real thing. Look at the hyperconverged infrastructure providers; all they talk about these days is cloud this and cloud that.
Another company Lopez recently spoke with sells something with “blockchain” on the labeling — but she couldn’t actually find the distributed ledger anywhere in the product.
“I couldn’t figure it out. And it turns out they use [globally unique identifiers] to identify things. And that’s not blockchain — it’s just an identifier,” she said.
Image recognition is another one to watch for, according to Lopez. “I don’t really consider it visual recognition services if they just look for red pixels. I mean that’s not quite the same thing,” she said. “This is also making things much harder for your average CIO or worse, CFO, to understand whether they’re getting any value from these technologies.”
In the case of AI, where the expectation is that the technology can act on behalf of humans, companies must know what the tool is really doing and where they need to pick up the slack.
During last week’s theCUBE NYC event in New York, a special Influencer Panel came together to discuss all things big data and AI, as well as the pitfalls of reliance on biased or incomplete data and imperfect analytics models. The panel, led by Dave Vellante (@dvellante) and Peter Burris (@plburris), co-hosts of theCUBE, SiliconANGLE Media’s mobile livestreaming studio, included: Penn, Lopez; Bob Hayes, president of Business Over Broadway; Steve Ardire, AI startup advisor; Carla Gentry, data scientist; Tony Flath, tech and media consultant, cloud and cybersecurity; Kevin L. Jackson, founder of GovCloud Network and author; Mark Lynd, founder of Relevant Track; and Theodora Lau, founder of Unconventional Ventures.
The panel all things big data and AI, and the pitfalls of reliance on biased or incomplete data and imperfect analytics models. (* Disclosure below.)
It’s evident that many enterprises are gung-ho to try out all the hyped-up AI software hitting the market. Revenue from AI technologies will hit $1.2 trillion by the end of this year, up 70 percent from the year before, according to a report from research firm Gartner Inc. These tools leverage the latest advances in machine learning and deep learning.
“Deep neural networks allow organizations to perform data mining and pattern recognition across huge datasets not otherwise readily quantified or classified, creating tools that classify complex inputs that then feed traditional programming systems,” wrote John-David Lovelock, a research vice president at Gartner. “Such capabilities have a huge impact on the ability of organizations to automate decision and interaction processes.”
But simply buying a tool — even a good one — and juicing whatever data a company has on hand through it doesn’t guarantee success. “There’s still the need for humans to look at the data and realize that there is the bias in there,” Lynd said. Sometimes the data left out of a set results in the worst skews, he added.
Models themselves can take on the imperfections of poorly selected data, according to Gentry.
“We have to think about things being biased, being fair, and understand that this data has impacts on people’s lives,” she said. AI models are like pets that data scientists are never done training. And poorly-trained models can do more than make crumby swivel-chair recommendations on Ikea.com.
A few high-profile stories have made the news recently, sounding alarm over use of AI algorithms in the justice system. A tool called COMPAS (which stands for Correctional Offender Management Profiling for Alternative Sanctions), made by Northpointe Inc., is supposed to help judges predict likelihood of recidivism in defendants. The software has been implicated in a number of controversies around criminals who were released and re-offended and others who challenged the algorithms decision in court and lost.
With the state of AI as it is, humans can’t be taken totally out of the loop, according to Ardire.
“Credible AI does the who, what, where, when and how — but not the why,” he said. Explainable AI is a new moniker that refers to the synergy of AI and human intelligence for drilling down to root causes. It’s necessary to drill to the root cause in order to make decisions — otherwise, people are just making correlations.
Those correlations that AI algorithms make might add to an insight that is harmful to businesses. Just ask loads of small businesses that would rather have profiles on popular rating sites.
“But data’s being collected about them. It’s being put on Yelp; they’re being rated; they’re being reviewed,” Lynd stated. “The success of their business is out of their hands.”
Some are looking into technologies like blockchain that will give people and businesses more personal control over their data.
For now, the best advice for everyone is to use the AI — on websites, in enterprise software, and algorithms, etc. — as an assist, but not the be-all, end-all, Lynd pointed out. So act on the recommendation — in the measured way that only a human being can.
“I think you should do it, but you should use it for what it is. It’s augmenting; it’s assisting you to make a good decision. And, hopefully, it’s a better decision than you would have made without it,” he concluded.
Watch the complete video interview below, and be sure to check out more of SiliconANGLE’s and theCUBE’s coverage of theCUBE NYC event. (* Disclosure: IBM Corp. sponsored this segment of theCUBE. Neither IBM nor other sponsors have editorial control over content on theCUBE or SiliconANGLE.)
THANK YOU