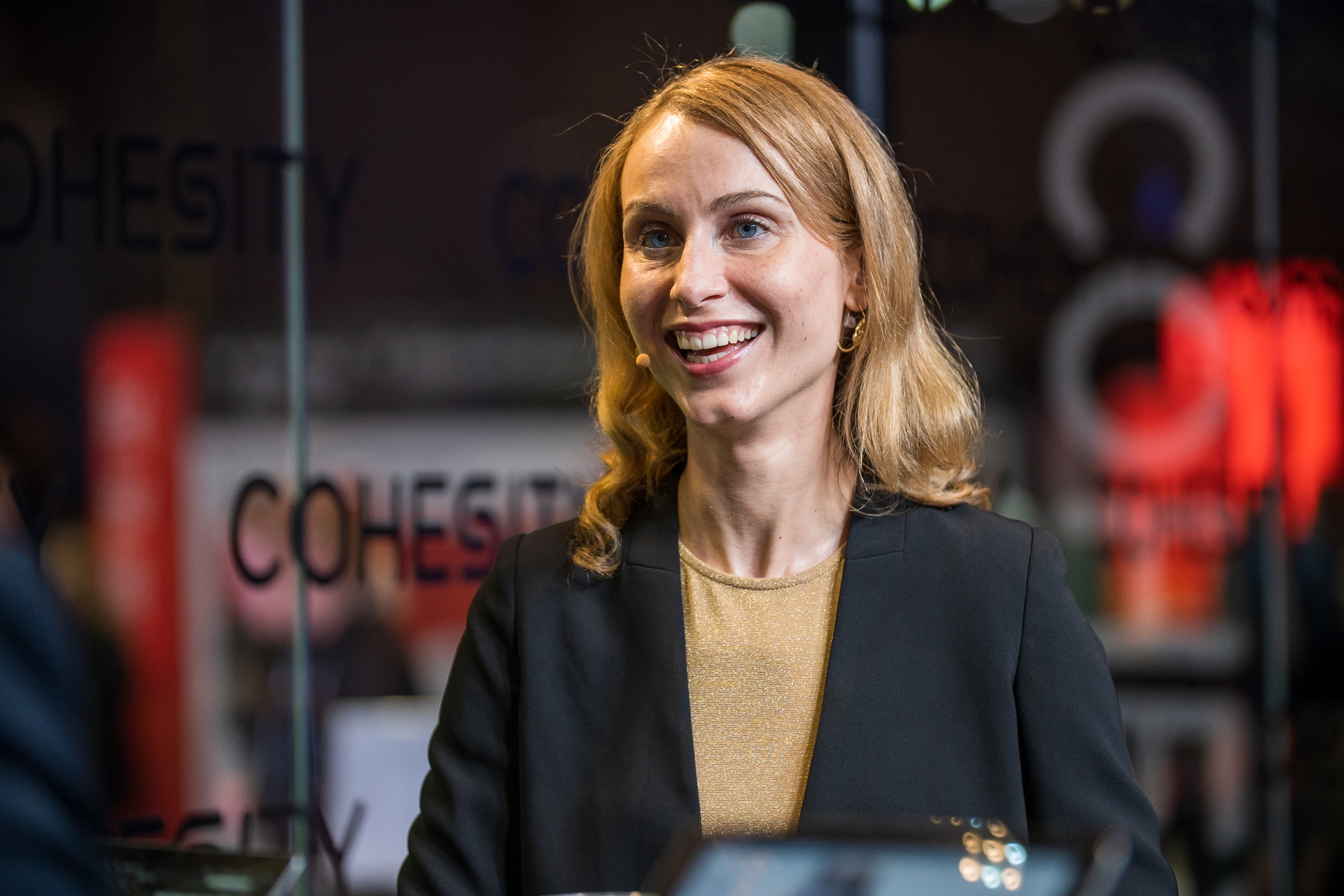
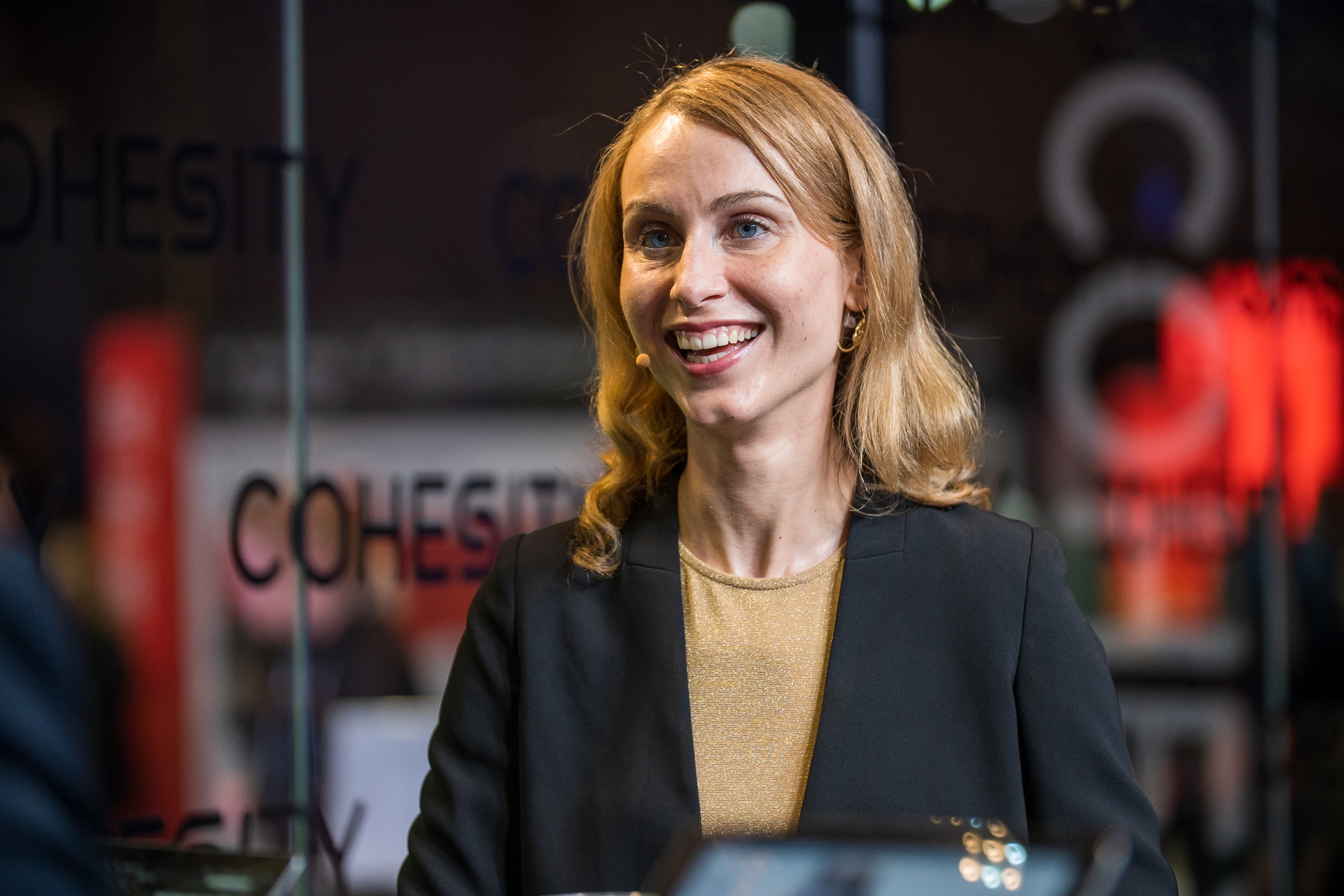
The rise of artificial intelligence has brought warnings against biased, deceptive and malicious applications.
But there’s a way to avoid bias and ensure responsible AI, according to Francesca Lazzeri (pictured), senior machine learning scientist and cloud advocate at Microsoft Corp.: visibility.
“In my team, we have a toolkit, which is called an ‘interpretability toolkit,’ and it’s really a way of opening machine-learning models and understanding what are the different relationships between different variables, different data points,” Lazzeri said. “It is an easy way you can understand why your model is giving you specific results.”
In addition to working at Microsoft, Lazzeri is also a mentor to Ph.D. and postdoctoral students at the Massachusetts Institute of Technology.
She spoke with Stu Miniman and Rebecca Knight, co-hosts of theCUBE, SiliconANGLE Media’s mobile livestreaming studio, during the Microsoft Ignite event in Orlando, Florida. They discussed the path to responsible AI and Microsoft’s recent releases in machine learning (see the full interview with transcript here).
Most bias issues found in AI applications start with the data, according to Lazzeri. “You have to make sure that the data is representative enough of the population that you are targeting with your AI applications,” she said. “Most of the time, customers just use their own data. Something that is very helpful is also looking at external type of data.”
Another way to avoid problems is to check the model with business and data experts. “Sometimes we have data scientists that work in silos; they do not really communicate what they’re doing,” Lazzeri pointed out. “You have always to make sure that data scientists, machine-learning scientists are working closely with data experts, business experts, and everybody’s talking … to make sure that we understand what we are doing.”
For companies just beginning their machine-learning journey, the first step is to identify the business question that must be answered, Lazzeri explained: “As soon as they have this question in mind, the second step is to understand if they have the right data that are needed to support this process.”
After that, it is important to be able to translate the business question into a machine-learning question. “And, finally, you always have to make sure that you are able to deploy this machine-learning model so that your environment is ready for the deployment and what we call the operational part,” Lazzeri said. “That’s really the moment in which you are going to add business value to your machine-learning solution.”
Here’s the complete video interview, part of SiliconANGLE’s and theCUBE’s coverage of Microsoft Ignite:
THANK YOU