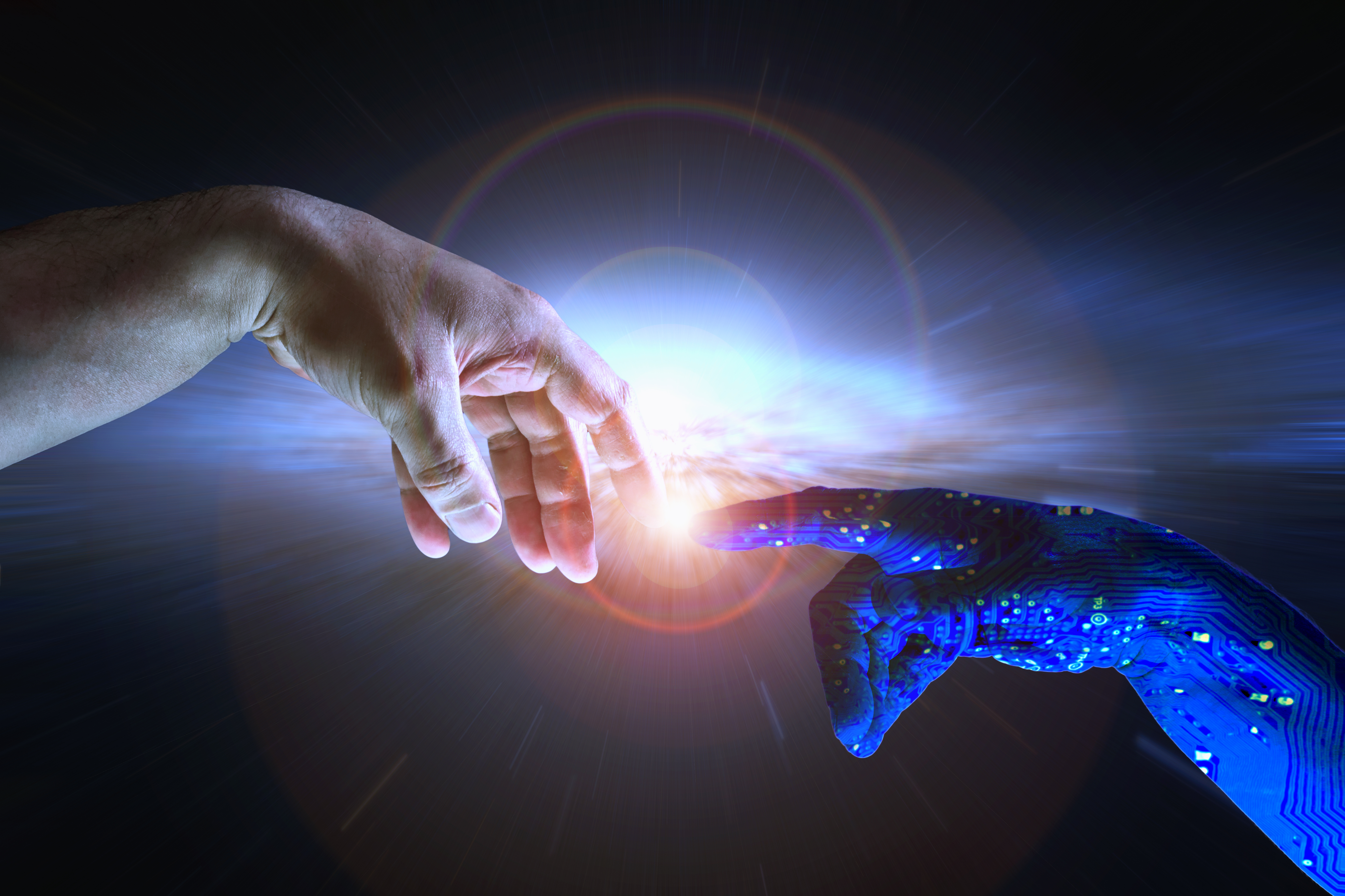
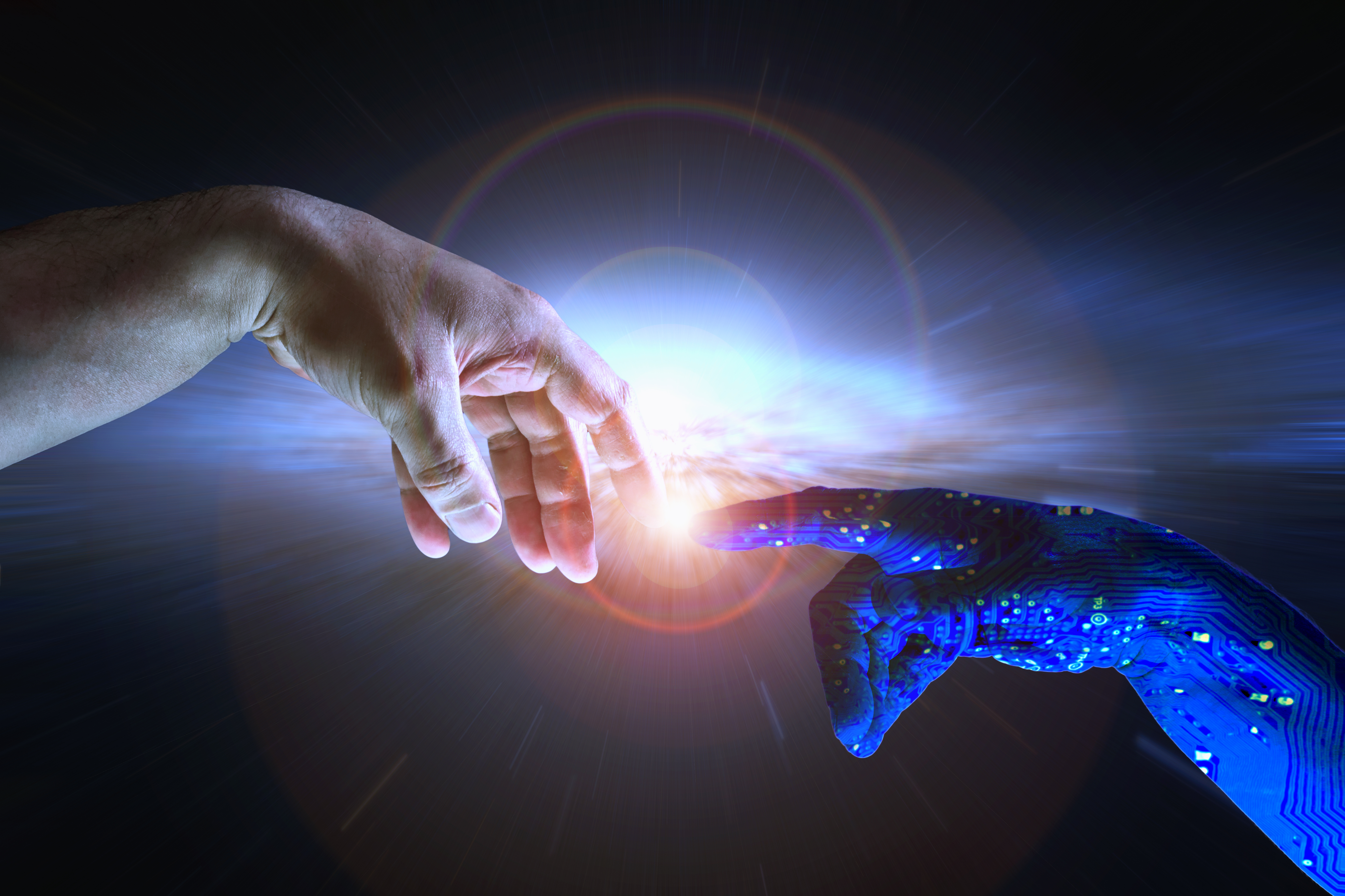
Data’s takeover of society started at the turn of the twenty-first century, and its influence has steadily increased ever since. Today, algorithms shape decisions across all areas of society, and the effects resonate in people’s personal lives.
Throughout history, people have been polarized by new technology. Luddites and early adopters alike have proclaimed fear or enthusiasm for such advancements, and the rise of data is no exception. Progress in artificial intelligence, driven by data, holds immense potential for the future of mankind. Yet inherent problems with flawed data and imperfect algorithms are further marginalizing those whom society already discounts.
“We cannot let these algorithms and these approaches of data-driven decision making really play the significant role that they are starting to play in our society at large if we do not really understand the ethics,” said Margot Gerritsen, Stanford University professor and co-founder and co-director of the Women in Data Science Worldwide initiative. Gerritsen spoke with SiliconANGLE Media in anticipation of this year’s WiDS Worldwide Conference on March 8, an event that theCUBE, SiliconANGLE’s livestreaming studio, regularly covers.
Achieving algorithm and data transparency, accountability and fairness are the ultimate goals of ethical data science. But achieving those goals is hard when the information technology workforce is struggling with the same issues.
“When it comes to building technology, right now the gatekeepers of tech are kind of a homogenous group [who] build tech solutions for the entire world,” said equality advocate Alex Qin, founder and chief executive officer of nonprofit software startup Emergent Works. Qin’s fight for empathy and equality was spotlighted in a Women in Tech feature.
The data backs Qin’s statement. A 2019 Boston Consulting Group report showed that 78% to 85% of data scientists are male.
Stanford’s Women in Data Science initiative has been in the frontlines of the battle for diversity in data science since its first conference in 2015. This year’s conference marks the launch of a workshop series taught by women. The aim is to make data science education available for everyone regardless of gender, age or ethnicity. A key aspect is teaching the importance of ethics.
“Really thinking about ethics in a broad sense around what you do is unbelievably important and should be taught right at the get go,” Gerritsen said. “Everybody who makes decisions with data, who develops algorithms, students who start learning about data in high school, all of those people need to … also understand about the human dimension of data.”
Taking that holistic view of data science means establishing an inclusive and diverse community of data scientists.
“Algorithms are only as strong as the people that have developed them,” said Natalie Evans Harris, senior advisor to the U.S. Department of Commerce and advisor to the Cloudera Foundation and HData, who spoke with theCUBE during an interview at WiDS 2019. “We need people with diverse thoughts because they’re the ones we’re going to create, those algorithms that make the machine learning and the algorithms in the technology more powerful, more diverse, and more equal.”
The problems that occur from biased or incomplete data are widely acknowledged, but there is still a misplaced trust in the algorithms themselves, according to Gerritsen. “A lot of people believe that if you build the codes, you build an algorithm it’s impartial. But it’s not,” she stated.
The idea is nothing new. In her 2017 Ted talk, Cathy O’Neil, mathematician, data scientist and author of “Weapons of Math Destruction,” stated that “algorithms are opinions embedded in code.” Despite the data scientists’ best intentions, algorithms can have deeply destructive effects, something O’Neill contrasts with more visible engineering errors.
“An airplane that’s designed badly crashes to the earth, and everyone sees it,” she said. “An algorithm designed badly can go on for a long time, silently wreaking havoc.”
Problems occur when AI algorithms are trained and then let loose to make decisions without the ability to track back and see why and on what data those decisions were based. This is a critical difference from human decision-makers, who can always be questioned to establish how and why they made a decision and if it is based on inaccurate information, biases or false assumptions, Gerritsen pointed out.
The only answer is to have those algorithms created by teams of data scientists that accurately model the true diversity of humanity culturally, racially, ethnically and sexually. But that’s a tall order, and one that can’t be magicked into being.
Making data science more open and welcoming to women and minorities is important, but it won’t solve a problem that is affecting hundreds of thousands of people today. Applications for jobs, mortgages and personal loans get rejected by machines simply because they do not tick the correct boxes — boxes that are statistically likely to have been selected by a relatively wealthy white or Asian male.
Many organizations have created ethical data ground rules in an effort to immediately address these problems. However, there is as yet no commonly agreed standard, and existing guidelines are often convoluted. For example, the United Kingdom provides a framework for government agencies to follow. The guidelines instruct data practitioners, policymakers and operational staff to rank projects on a level of zero to five on factors such as understanding of the unintended consequences, understanding of user needs, and compliance with laws and regulations. While it was created with positive intent, its actual practical application is debatable. The United States government offers seven data ethics tenets, outlined in the 28-page Data Ethics Framework document.
Non-profit organizations dedicated to data ethics have more practical means to ensure the ethical use of data. The Deon ethics checklist was created by data scientists for data scientists and is the basis of a WiDS workshop on Actionable Ethics for Data Scientists.
“Deon … is a tool that we built at [non-profit organization] DrivenData which lets you easily add an ethics checklist to your data science projects,” explained the organization’s senior data scientist Emily Miller.
The checklist doesn’t pinpoint “remove variable x from your model,” she explains in her workshop. However, it does help data scientists identify major ethical questions that can cause problems, such as revealing personally identifiable information, collections bias, and non-auditable data analysis processes.
To collate all the disparate ethics frameworks, checklists and recommendations, the German organization AlgorithmWatch created the AI Ethics Guidelines Global Inventory. The inventory currently holds 160 guidelines, which are accessible using a search and filter function, and contributors can submit missing guidelines via a form.
Combating biased and discriminatory algorithms is an urgent mission. Yet, too many people remain unaware of the affects hidden AI can have on their lives.
“Data-driven decision making is penetrating all aspects of society, so that means that people from all sorts of educational backgrounds and with all sorts of skill sets really need to be data-savvy,” Gerritsen said. “Who is going to train the politicians in data science? Who is going to train the manager, the CEO in data science? Who is going to give the humanist an entry into data science that is approachable to them?”
The answer is that we are all responsible. Achieving ethical data management is a balancing act, and the struggle for transparency, accountability and fairness will continue as long as intelligent technology does. There may never be an absolutely impartial algorithm, but a combination of education and increased diversity in data science can help tilt the balance toward the trustworthy and ensure that AI algorithms make decisions that reflect the conscientious side of their human creators.
THANK YOU