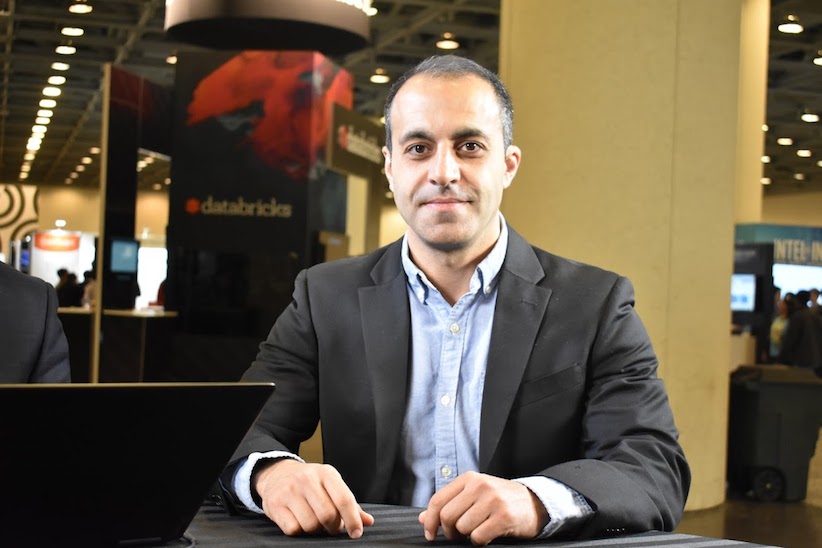
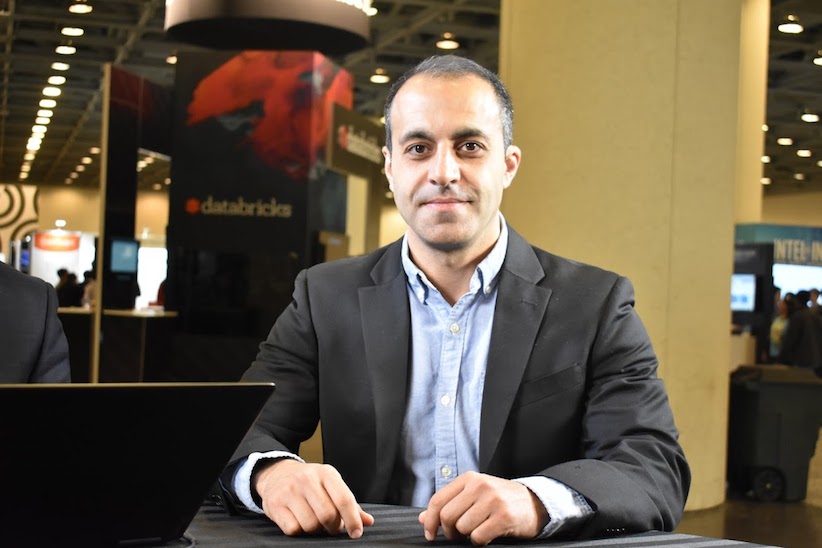
Databricks Inc., the distributed data unicorn with a $38 billion valuation, and Snowflake Computing Inc., the cloud data warehousing pioneer with the $107 billion market capitalization, have been on a collision course of late — a reckoning Databricks hopes to stoke today with the announcement that its cloud data warehousing software has set a world record for performance on a major benchmark test.
According to tests audited by the nonprofit Transaction Processing Performance Council, which oversees the TPC-DS benchmark suite, Databricks performed 2.2 times faster than the previous world record holder on a test against a 100-terabyte database. Databricks said TPC is expected to publish the results on its website today, in effect blessing them. Update: The results were published here on Wednesday.
Databricks said the tests show that the Apache Spark-based distributed architecture it calls a “lakehouse” can deliver better performance than traditional data warehouses without all the data transformation, normalization and uploading that’s required. The result, it says, is the optimal combination of flexibility and speed. “I don’t think you’ll have data warehouses in the future,” Databricks Chief Executive Ali Ghodsi (pictured) said in an interview with SiliconANGLE.
However, benchmarks have long been a controversial measure of performance for all types of computer hardware and software and Databricks’ announcement may have more symbolic value than the potential to move the needle on customer decisions.
“Take any claims based on benchmarks with a considerable grain of salt,” said Carl Olofson, a research vice president at International Data Corp., who noted that he has not seen Databricks’ published results. “When a single vendor does one, it invariably configures the test to show the vendor’s product to its best advantage. Comparing results of even a standard benchmark is problematic unless the tests are done by the same independent lab using the same technology configuration.”
Databricks said its results have been formally audited and reviewed by the TPC-DS Council and are buttressed by a separate study conducted by the Barcelona Supercomputing Center that it said showed Databricks outperforming the next-fastest cloud data warehouse by 170%. It also said a separate TPC-DS benchmark on an earlier version of its engine found the database outperformed the world record holder by 10% on price/performance, “and we didn’t use a custom version of Databricks,” Ghodsi said. “The newer versions are even faster.”
One of the tricky aspects of most benchmarks is that few of the nonprofit organizations that define them have the budget or people to perform head-to-head tests. As a result, the task is usually left to the vendors themselves using a set of approved test criteria. In the case of TPC-DS, that includes 99 queries of varying complexity run against a 100-terabyte data warehouse in a multiprocessor configuration.
This creates a natural tendency for vendors to find workarounds that reflect favorably on their products, a process Databricks acknowledged in a blog post to be published today, a draft of which was provided to SiliconANGLE.
Because of the benchmark’s complexity “many data warehouse systems, even the ones built by the most established vendors, have tweaked the official benchmark so their own systems would perform well,” the post says. That’s one reason only a few vendors have ever published their results and “most vendors seem to beat all other vendors according to their own benchmarks.”
Indeed, the list of cloud-based data warehousing engines for which TPC-DS results have not been published features nearly all of the most popular products including Amazon Web Services Inc.’s Redshift, Snowflake, Google LLC’s BigQuery, IBM’s DB2 warehouse, Microsoft Corp.’s Azure Synapse and Oracle Corp.’s Autonomous Warehouse.
Although most vendors provide sample scripts for customers to use in performing their own benchmark, few have submitted to a formal audit. Neither Google nor Snowflake responded to a request for comment.
Another common criticism of benchmarks is that they fail to reflect the complexity of the real world in which organizations operate. “To support decision-making, an architecture may require multiple types of data management technologies and it’s impossible to do an apples-to-apples comparison among them,” said Dan Vesset, group vice president for analytics and information management at IDC, who also had not seen the details of Databricks’ announcement. “For example, an organization may use a relational database for data warehousing, a graph database, time-series database and a data lake or a lakehouse” for different kinds of processing loads.
Cloud data warehouses have, to some extent, also obviated the debate over performance, said David Vellante, co-founder and chief analyst at Wikibon, a sister research firm of SiliconANGLE. “With cloud data warehouses, you can throw virtually infinite compute at the problem and shut down the compute when you’re done,” he said.
Databricks said it overcame numerous barriers to achieve its performance milestones, including the typically slow evolution of open-source projects compared to their proprietary counterparts, its product’s lack of support for massively parallel processing computers and its historically weak performance on small queries.
It responded with initiatives like Delta Lake, which addressed some of the weaknesses of the open-source Parquet storage engine, along with architectural changes that enable SQL queries to exploit cached data more efficiently. It also rewrote its engine from scratch in a new version called Photon that is optimized for parallel query processing.
“Spark is not a warehouse, so we built a new engine just for data warehousing,” Ghodsi said. “With a single compute instruction, you can operate on a lot of data in parallel.”
Although Databricks is likely to try to make hay with the benchmark results, IDC’s Olofson cautioned against writing the epitaph for data warehouses anytime soon.
“Query speed is only one characteristic of a data warehouse that makes it competitive, and not necessarily even the most important one,” he said. Data consistency, orthogonal query processing, large-scale multi-user capacity and massive schema support of hundreds of tables and thousands of foreign key references are among the strengths traditional warehouses still have, he added.
Vellante also lamented the industry’s continuing focus on raw speed when he believes more important issues need to be addressed. “The idea that a single vendor’s technology can solve your data problems is flawed thinking,” he said. Instead, vendors should be building products that enable business users “to own their own data and build data products without having to go through a highly specialized team that is more focused on managing Spark clusters than getting the data to work for the organization.”
Databricks, Snowflake and others, he said, “are so caught up in marketing and chasing valuation pressures that they’re missing the larger opportunity to work together to dramatically increase the size of the pie by creating new data standards that are open and facilitate a new model of data management.”
THANK YOU