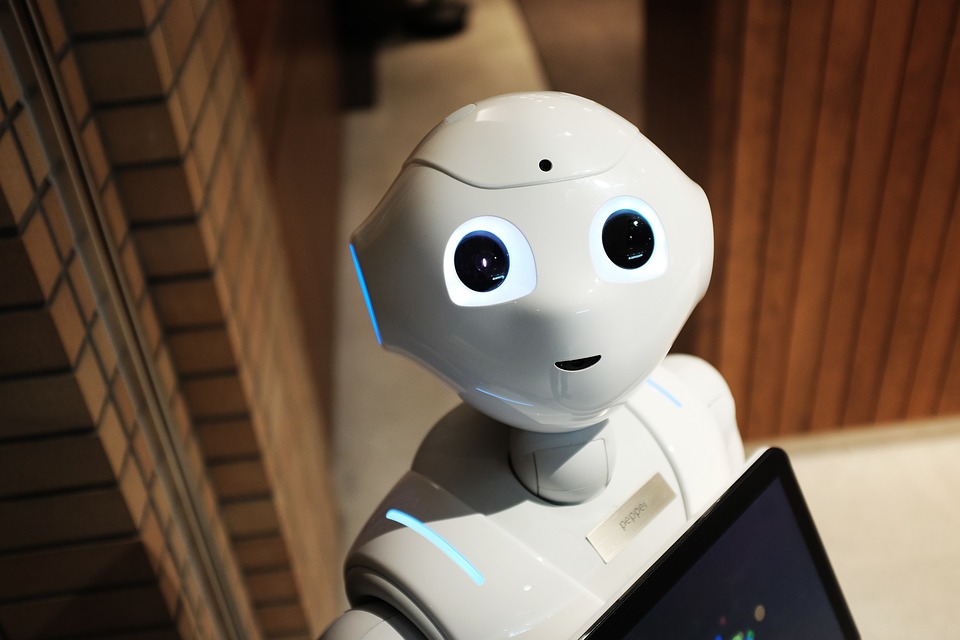
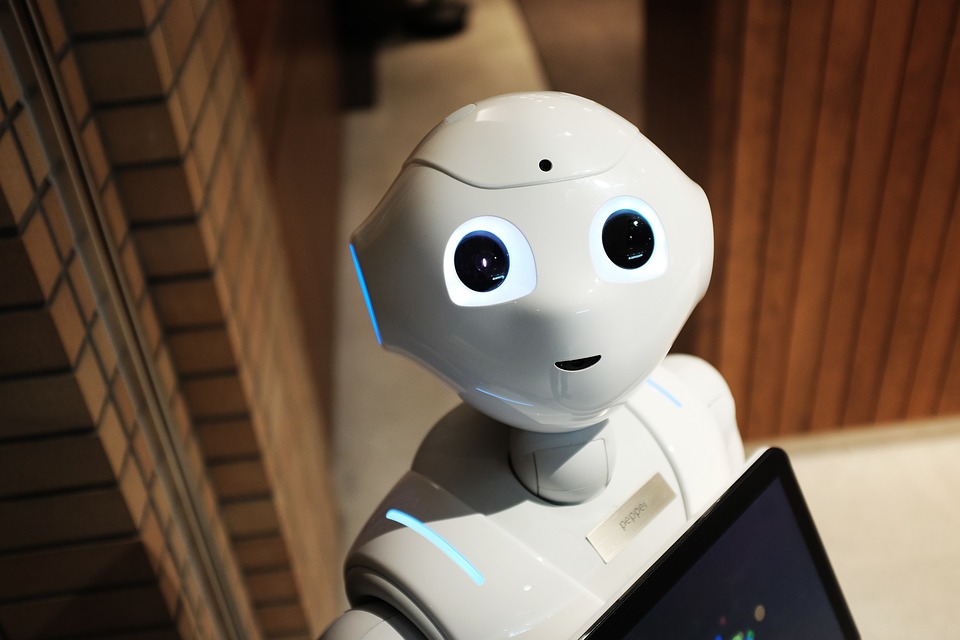
Meta Platforms Inc.’s artificial intelligence unit said today it has made rapid progress in its efforts to teach AI models to navigate through the physical world more easily and with less training data.
The research could dramatically reduce the time it takes to teach AI models the art of visual navigation, which has traditionally been possible only through “reinforcement learning” that requires massive data sets and repetition.
Meta AI’s researchers said their work on visual navigation for AI will have big implications for the metaverse, which will be made up of continuously evolving virtual worlds. The idea is to help AI agents navigate these worlds by seeing and exploring them, just as humans do.
“AR glasses that show us where we left our keys, for example, require foundational new technologies that help AI understand the layout and dimensions of unfamiliar, ever-changing environments without high-compute resources, like preprovided maps,” Meta AI explained. “As humans, for example, we don’t need to learn the precise location or length of our coffee table to be able to walk around it without bumping into its corners (most of the time).”
To that end, Meta has focused its efforts on “embodied AI,” which refers to training AI systems via interactions in 3D simulations. In this area, Meta said it has created a promising new “point-goal navigation model” that can navigate through new environments without any map or GPS sensor.
The model implements a technique known as visual adometry that allows AI to track its location based on visual inputs. Meta said this data augmentation technique can be used to train effective neural models rapidly without human data annotations. It has been successfully tested in Meta’s Habitat 2.0 embodied AI training platform that runs simulations of virtual worlds with a 94% success rate on the Realistic PointNav benchmark task, Meta said.
“While our approach does not yet completely solve this data set, this research provides evidence to support that explicit mapping may not be necessary for navigation, even in realistic settings,” Meta said.
To boost AI navigation training further without relying on maps, Meta has created a training data collection called Habitat-Web that features more than 100,000 different human demonstrations for object-goal navigation methods. That connects the Habitat simulator running in a web browser to Amazon.com Inc.’s Mechanical Turk service and allows users to teleoperate virtual robots safely at scale. AI agents trained with imitation learning on this data can achieve “state-of-the-art results,” Meta said, by learning efficient object-search behavior from humans such as peeking into rooms, checking corners for small objects ad turning in place to get a panoramic view.
In addition, Meta’s AI team has developed what it calls a “plug and play” modular approach to helping robots generalize from a diverse set of semantic navigation tasks and goal modalities through a unique “zero-shot experience learning framework.” The idea is to help AI agents adapt on the fly without resource-intensive maps and training. The AI model is trained to capture the essential skills for semantic visual navigation just once, before applying them to different tasks in a 3D environment without any additional retraining.
Meta explained that agents are trained by searching for image goals. They receive a picture taken from a random location in their environment and then they must travel and try to locate it. “Our approach requires up to 12.5x less training data and has up to 14 percent better success rate than the state-of-the-art in transfer learning,” Meta’s researchers said.
Constellation Research Inc. analyst Holger Mueller told SiliconANGLE that Meta’s latest advancements could play a key role in the company’s metaverse ambitions. If virtual worlds are to become the norm, he said, AI needs to be able to make sense of them and do so in a way that’s not too cumbersome.
“The scaling of AI’s ability to make sense of physical worlds needs to happen through a software-based approach,” Mueller added. “That’s what Meta is doing now with its advancements in embodied AI, creating software that can make sense of its surroundings on its own, without any training. It will be very interesting to see the first real-world use cases in action.”
Those real-world use cases could happen soon. Meta said the next step is to push these advancements from navigation to mobile manipulation, so as to build AI agents that can carry out specific tasks such as locating a wallet and bringing it back.
THANK YOU