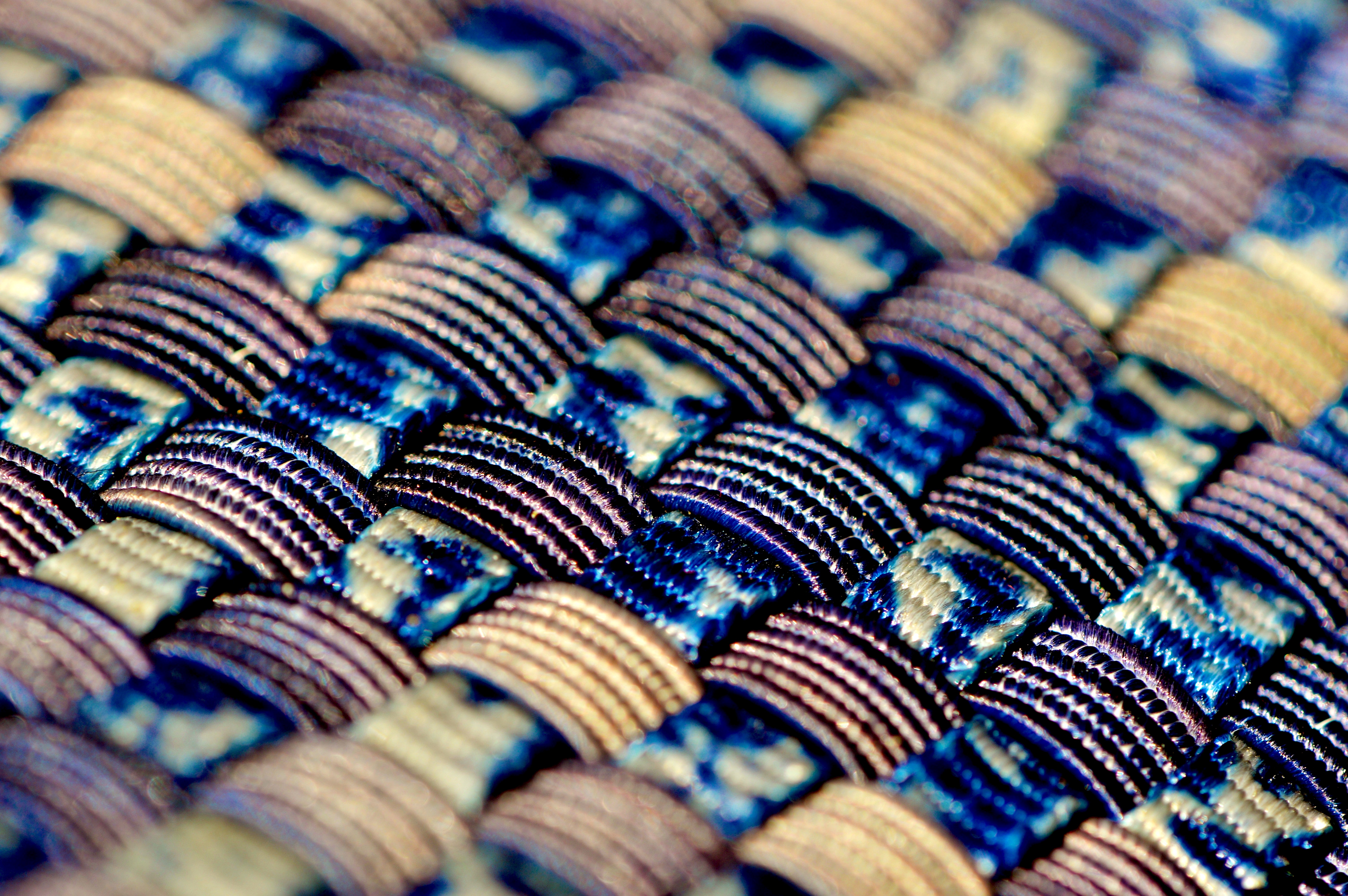
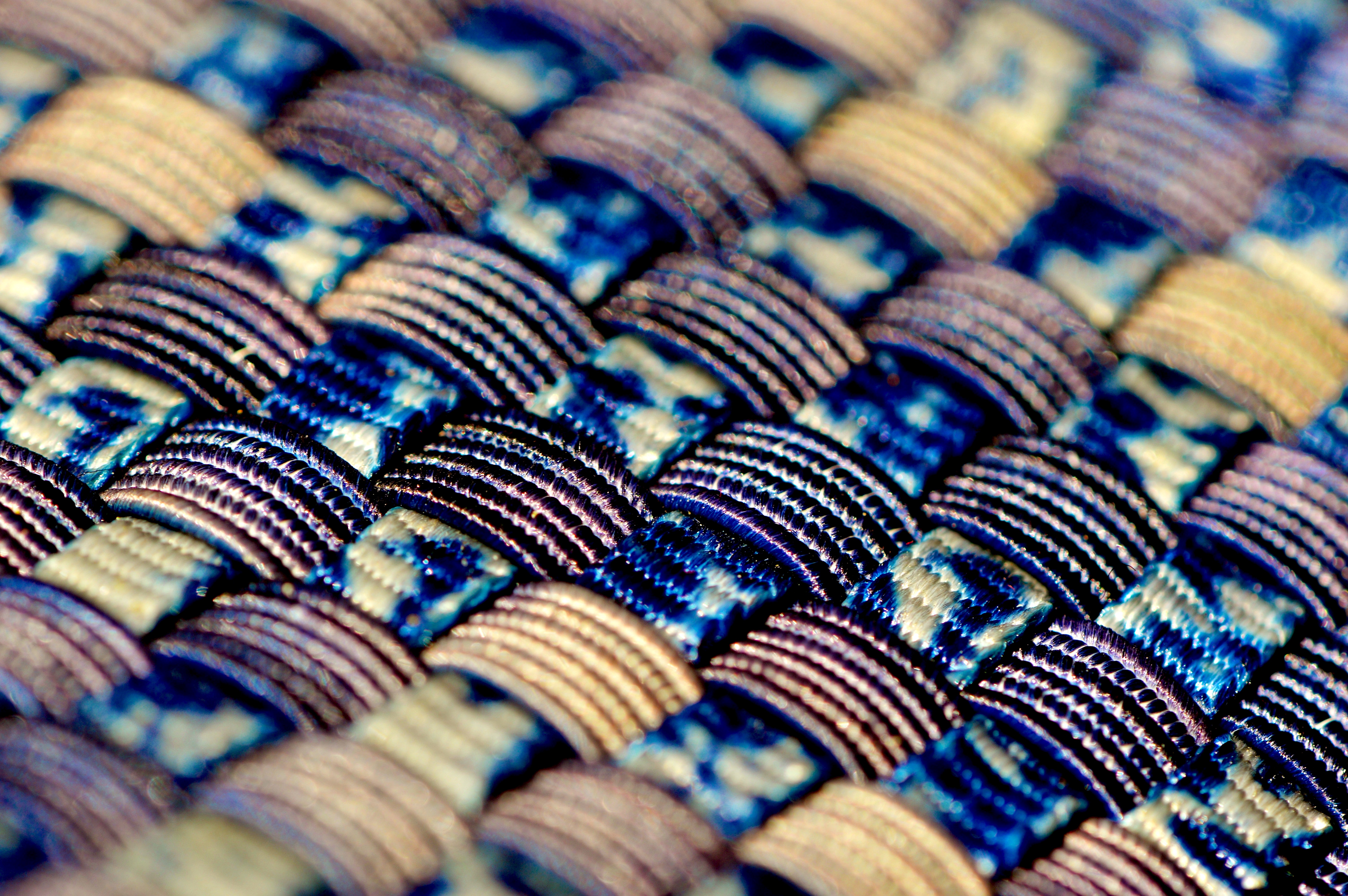
Almost any business, large or small, that uses technology typically has a strategic supplier that is, in effect, first among equals. It becomes the platform that drives choices for third-party applications, tools or databases. In small businesses, that strategic platform supplier is likely to be Microsoft Corp. or Apple Inc., with the choice of Google LLC’s Android or Apple’s iOS on the mobile side. In midsized to large enterprises, platforms are more likely to be multipolar, reflecting the fact that few if any of them are likely to standardize on any single core supplier.
As preeminent enterprise application provider, SAP SE is often thrust into the role of strategic supplier. There are lots of fun facts supporting this, with one of the most common being that 77% of the world’s transaction revenue touches an SAP system. Use of SAP very much shapes the choices they make for databases, analytics and supporting applications.
But in those same organizations, there are also likely to be groups working outside the SAP environment. Maybe parts of the organization use Oracle Corp.’s e-Business Suite or Microsoft Dynamics, or it’s groups of business analysts working with analytics, or it’s data scientists building model from data lakes. More often than not, the views of data may be shaped by whether you’re working inside the walled garden of the enterprise application or outside it.
Hold that thought.
For data management, the most pressing issues that we’re seeing are about enterprises getting a better handle on their vast and growing sprawls of data. Data is not simply becoming more diverse, but increasingly, becoming more distributed. The perfect storm of cloud computing, connectivity and the reach of 5G has extended the reach of data. And with ubiquitous connectivity come concerns over privacy and data sovereignty that are, literally, setting the boundaries for what data can be consumed by whom, in what form and where. For SAP customers, the world of data has exploded outside their SAP applications.
One byproduct of this has been interest in data mesh, where ownership and lifecycle management are sharply delineated to the business units, subject matter experts or domains that have the most knowledge of and stake in the data. At the other end of the spectrum is building a logical infrastructure for ensuring that the right data is discovered and delivered, and from that we’ve seen rising interest in data fabric. In our view, the two should complement each other, not cancel each other out.
The challenge is defining what a data fabric is. As we’ve seen with some analyst firm reports, a data fabric is what we used to term a data integration portfolio that encompasses catalog, data transformation and orchestration tools, data quality, data lineage and so on. That functional definition is a bit too loosey-goosey for us.
For us, a data fabric must start with a common metadata backplane. At minimum, it crawls data sources and harvests metadata. More advanced data fabrics use machine learning to enrich metadata based on inferences detected from patterns activity of source and target systems, such as which data sets or entities are frequently accessed together. The fabric should bury under the hood the complexities of discovering, accessing, transforming, governing and securing data.
The data fabric doesn’t necessarily perform those tasks, but it provides the logical superstructure to orchestrate the toolchain that exposes the data, regulates access, cleanses data, transforms it, masks it at run time and determines how data is accessed: Is data brought to the query engine (via replication) or vice versa (through virtualization)? A data fabric is needed, not when you’re simply sourcing data from a single transaction system, but from a variety of sources.
SAP is not new to the data integration game, as it has offered a number of tools and cloud services for data virtualization and replication. But the notion of going outside the SAP walled garden of data might be new for much of the installed base. Today, SAP is taking the wraps off what we view as a journey to building a data fabric: the new SAP Datasphere cloud service.
Datasphere combines and builds on two existing SAP offerings, including Data Warehouse Cloud, which was used for analytics, and Data Intelligence Cloud, which was a data integration hub. It capitalizes on the business semantic layer, which was what originally set apart SAP Data Warehouse Cloud from other cloud data warehousing services. Atop the existing combined technology stack, Datasphere adds a data catalog for data discovery along with new data quality, data pipeline orchestration, and data modeling capabilities. The result is a unified experience for data integration, data cataloging, semantic modeling, data warehousing, data federation and data virtualization.
SAP’s goal is not simply pairing a data transformation factory with a data warehouse, but instead delivering a service that preserves the context of source data. As you would guess, maintaining context relies on metadata. The challenge is that when you use existing tools for replicating, moving and transforming data, the metadata typically does not usually go along with it.
Admittedly, while schema might be implicit in moved data, business-level metadata or semantics will likely not be obvious. Add to the fact that SAP’s applications are a rich treasure store for business data and the process semantics that go with them. So, it’s logical that SAP has expanded on the business semantic layer of its DW cloud to deliver a data fabric that surfaces the metadata in business terms.
Another key design goal is an engine that should provide a guided experience, or guardrails for the best way to access data, such as whether it’s best to move data or virtualize it. That’s where having intelligence built into the fabric comes into play, where priorities for cost vs. service level, considered along with permissions on whether the data can be moved, comes in. Traditional data integration tools require the choice to be in the head of the user or data engineer.
Admittedly, within its own portfolio, SAP can exert control over the flow of metadata. For instance, modern suites such as S/4HANA have already unified the metadata. Across SAP’s enterprise application portfolio, metadata unification is a work in progress given the company’s long string of acquisitions, from Ariba to Qualtrics and others. What’s interesting is looking at its NextGen apps that are bridging some of those silos, such as Buying 360 that unifies overlapping workflows spanning some of those legacy apps. For instance, when onboarding a new hire in SuccessFactors, a workflow might kick in for office equipment through Ariba or business travel through Concur.
Preserving context gets tougher when dealing with external systems. That’s where you have to depend on the kindness of strangers, and for SAP, it’s where a new thrust for partnerships begins. SAP is launching partnerships with four household names in the analytics, data governance and data science space: Databricks Inc., which will integrate SAP data with its Delta Lake lakehouse; Collibra NV, for data governance; DataRobot Inc., for managing the life cycle for data science and AI projects; and Confluent Inc., for integration with streaming data.
The key benefit comes in preserving metadata context when working in partner environments. For instance, Collibra, which positions itself as a catalog of data catalogs, will surface governance and lineage metadata in Datasphere, and make sure that questions such as chain of custody over data are carefully tracked and enforced. Or with DataRobot, a data scientist who has built a model and then has it run in SAP should have a bidirectional connection that feeds model performance and data characteristics back to the data science tool.
At this point, we do not yet have the details of what SAP is delivering under the covers on day one with Datasphere, but rest assured that data fabrics are not built overnight. This will be a journey that will involve significant development of an intelligent orchestration engine that, for instance, recommends based on parameters such as cost, performance and response time, and data sovereignty as to how and where to run the query, and whether at run time the data needs to be dynamically masked.
The success of SAP Datasphere, like any data fabric, will rest on the depth and breadth of partner ecosystem support. And in the battle for mindshare, it will be about persuading non-SAP users that working within Datasphere will not curtail their ability to explore and model data wherever it is.
But let’s return to the question of complexity. When Datasphere was unveiled before a group of analysts, one of our colleagues from the application side asked whether this new data layer would complicate life for ERP users. We jokingly thought of the metaphor of application folks being from Venus and data folks being from Mars.
For application users, this is a real concern. For the heads-down enterprise resource planning or business warehouse user, the data catalog is an added layer. Ideally, you would like to see analytics embedded within your environment so you wouldn’t have to switch screens to a data catalog. SAP BW was developed precisely for those concerns, as it was conceived as a data warehouse for SAP enterprise application users. The original SAP Data Warehouse Cloud was conceived as the analytics tier of S/4HANA and, with its business semantic layer, it enabled S/4 users to work in their native language.
But this is about SAP connecting to the rest of the world of data. While the majority of world’s transaction revenue touches an SAP system, that doesn’t mean that a majority of the world’s data touches it. SAP’s challenge with its new data fabric is threefold. The first is building out the logical infrastructure that simplifies connecting users to data. The second is about recruiting a partner ecosystem to get, not only visibility to data, but a two-way exchange of metadata to keep the data in connect. And thirdly, it will be to make data not look like foreign territory to SAP’s vast application end-user case.
Tony Baer is principal at dbInsight LLC, which provides an independent view on the database and analytics technology ecosystem. Baer is an industry expert in extending data management practices, governance and advanced analytics to address the desire of enterprises to generate meaningful value from data-driven transformation. He wrote this article for SiliconANGLE.
THANK YOU