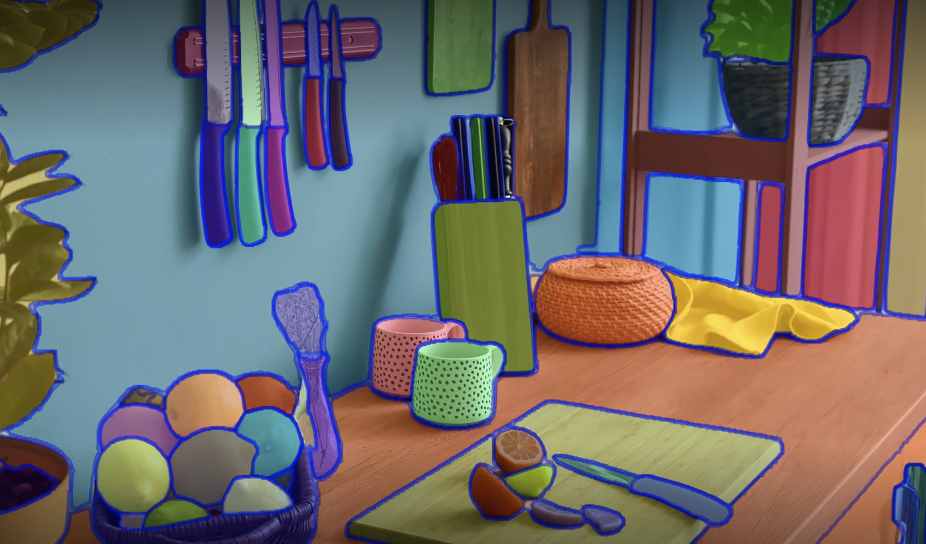
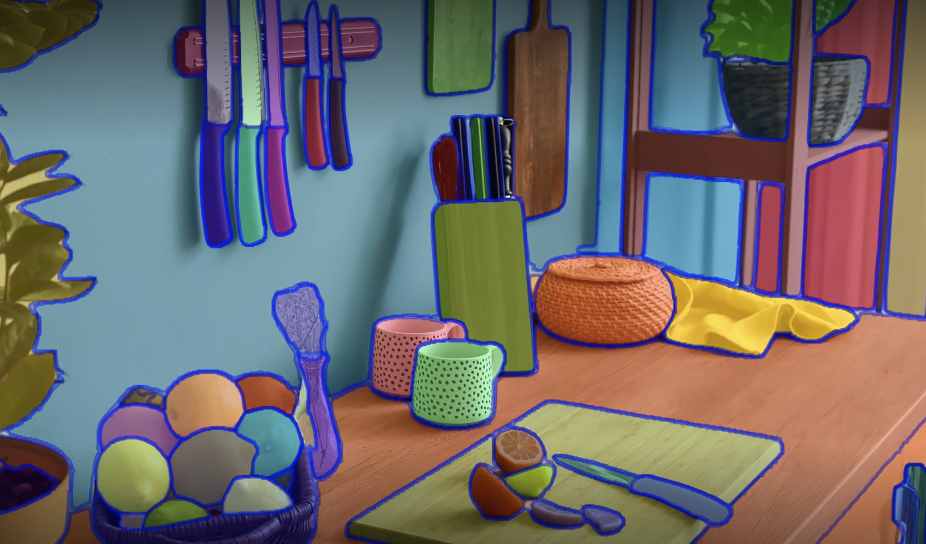
Artificial intelligence researchers at Meta Platforms Inc. said today that they’re hoping to democratize a key aspect of computer vision.
It’s known as “segmentation,” which refers to the ability to identify which pixels in an image belong to a specific object. The company said it’s releasing both its new Segment Anything Model, known as “SAM,” and a Segment Anything 1-Billion mask dataset, called SA-1B, to the research community. The intention is to encourage further research into the foundation models required to make computer vision.
Segmentation is a core task within computer vision that enables AI models to recognize objects they’re looking at in a given image. It’s used in a wide range of applications, from analyzing scientific imagery to editing photos. However, the task of creating an accurate segmentation model for specific use cases is something that’s beyond most AI researchers, since it requires highly specialized work by technical experts and access to extremely powerful AI training infrastructure and enormous volumes of annotated and domain-specific data.
These are the problems that Meta says it can overcome with SAM and SA-1B, which is the largest ever segmentation dataset to be released. The SA-1B dataset is being made available for research purposes, while SAM is being released under a permissive open license.
The Segment Anything project, as the initiative is known, is all about helping researchers by reducing the need for task-specific modeling expertise, training compute and custom data annotation. SAM was built to serve as a foundational model for image segmentation.
It’s a promptable model that’s trained on diverse data and can be adapted to many different tasks, similar to how prompts are used with natural language processing models. The challenge with segmentation is that the data required to train such a model is not available online or anywhere else, unlike with text, images and videos, which are abundant.
The SAM model has already learned a general notion of what objects are. It can generate “masks” for any object in any image or video, even for objects and images that it has not previously encountered. Masking is a technique that involves identifying an object based on the changes in contrast at its edges, and separating it from the rest of the scene. Meta’s researchers said SAM is general enough to cover a wide range of use cases and can be used out-of-the-box on any kind of image domain, whether that’s cell microscopy or underwater images or something else, with no additional training required.
As Meta explained in a blog post, SAM combines two common approaches to segmentation. They are interactive segmentation, where a human guides the model by iteratively refining a mask, and automatic segmentation, where the model does it by itself after being trained on hundreds or thousands of annotated objects.
The SA-1B image dataset used to train SAM contains more than 1.1 billion segmentation masks collected from 11 million licensed and privacy-preserving images, meaning it has 400 times more masks than any existing dataset. The extensive nature of the dataset is what enables SAM to generalize new types of objects and images beyond what it was trained on. As a result, AI practitioners no longer will need to collect their own segmentation data to fine-tune SAM for specific use cases.
Meta’s researchers say SAM can be useful in any domain that requires finding and segmenting any object found in any image. For example, it could be used as a component of larger AI systems that attempt to understand the world, such as a model that can identify both the visual and text content of a webpage.
Another application might be virtual and augmented reality, where SAM could select an object based on the user’s gaze, then lift it into the 3D domain. Other use cases include extracting parts of images for creative purposes and scientific studies.
“SAM can become a powerful component in domains such as AR/VR, content creation, scientific domains, and more general AI systems,” Meta’s AI researchers said. “As we look ahead, we see tighter coupling between understanding images at the pixel level and higher-level semantic understanding of visual content, unlocking even more powerful AI systems.”
THANK YOU