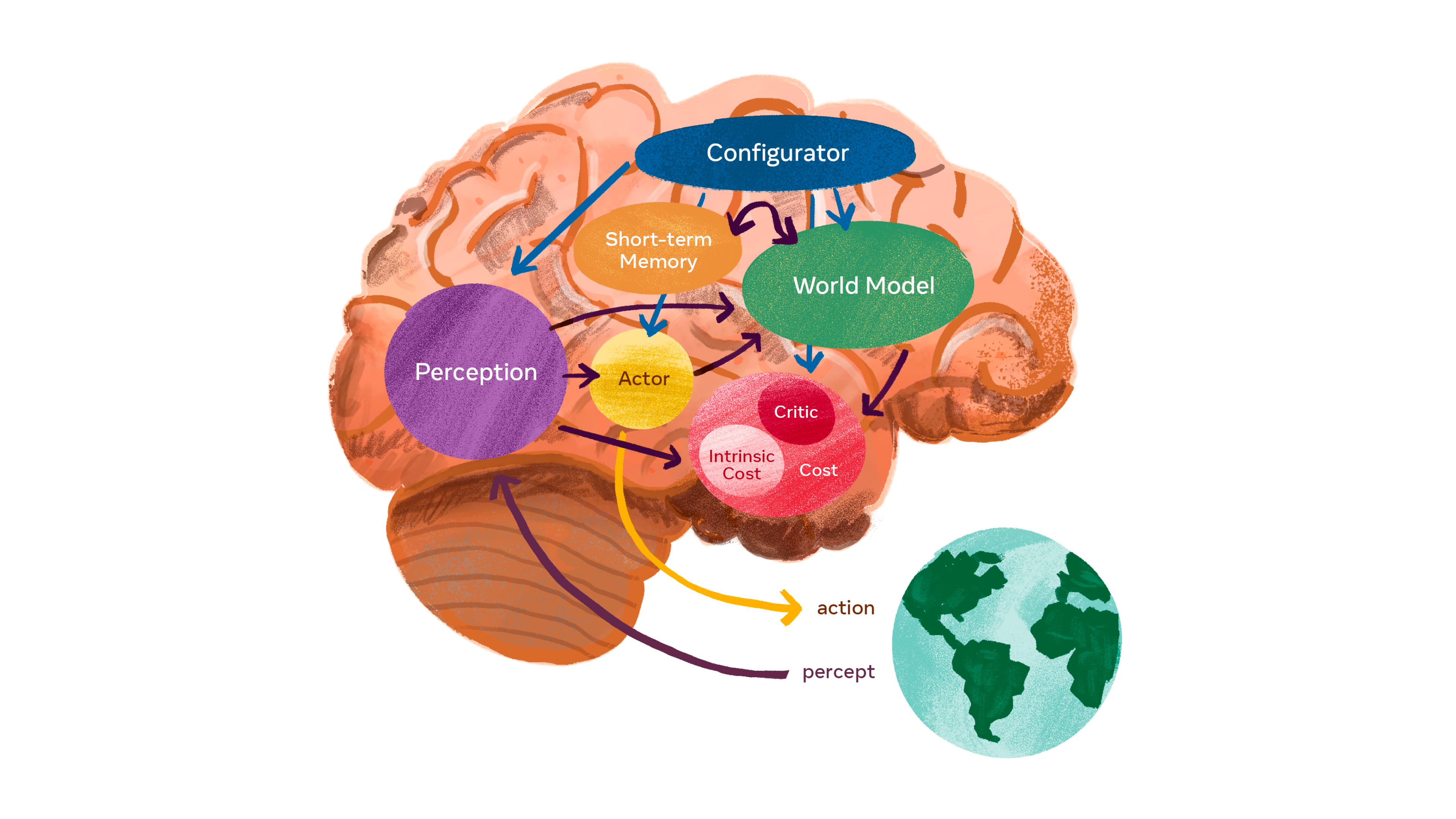
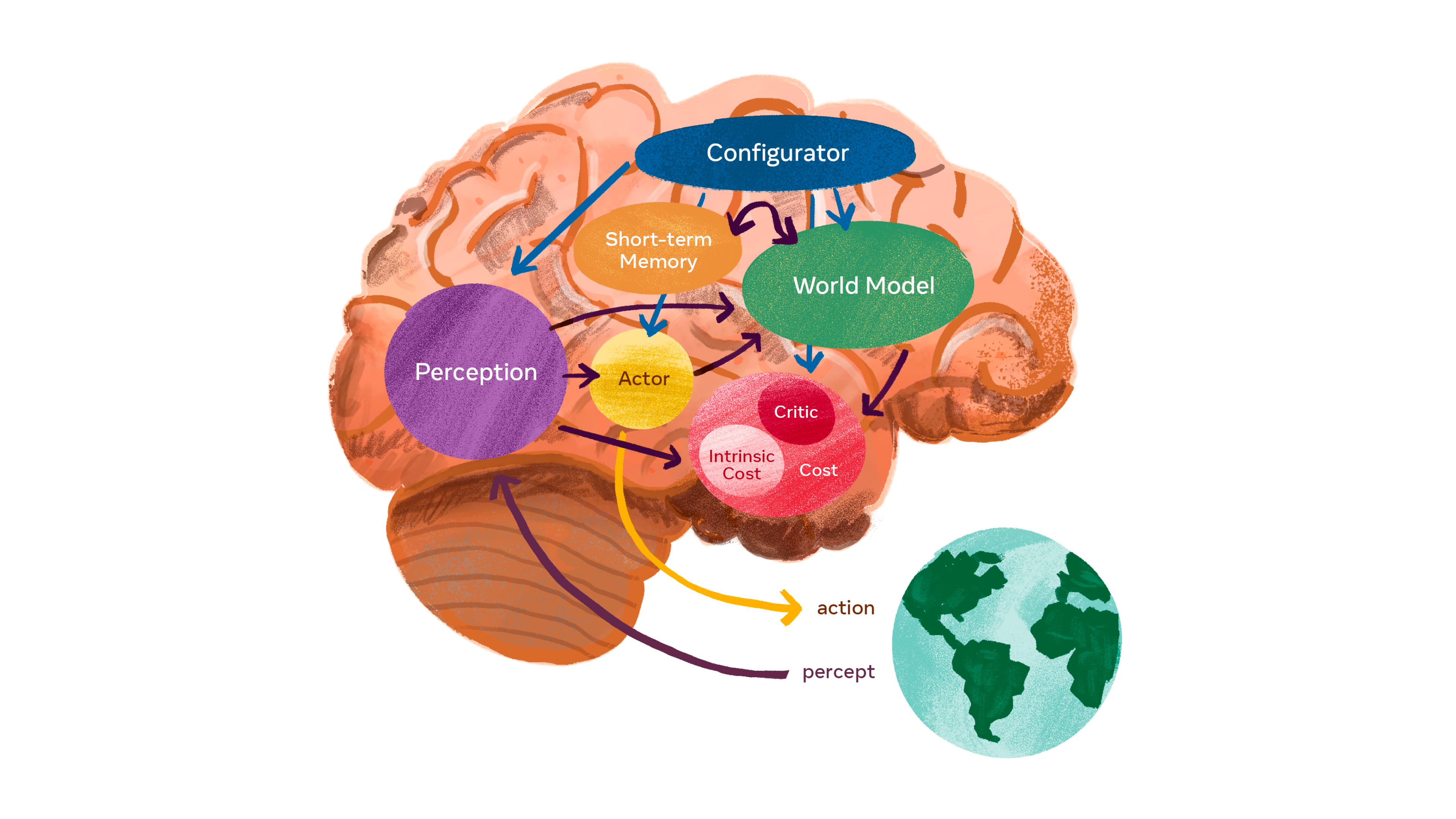
Artificial intelligence researchers from Meta Platforms Inc. say they’re making progress on the vision of its Chief AI Scientist Yann LeCun to develop a new architecture for machines that can learn internal models of how the world works.
The idea is that such an architecture would help AI models to learn faster, plan how to accomplish complex tasks and readily adapt to unfamiliar situations. Meta’s AI team said today it’s introducing the first AI model based on a component of that vision.
Called the Image Joint Embedding Predictive Architecture, or I-JEPA, it’s able to learn by creating an internal model of the outside world that compares abstract representations of images as opposed to comparing the pixels themselves. That means it learns in a way that’s much more similar to how humans learn new concepts.
I-JEPA is based on the idea that humans learn massive amounts of background information about the world as they passively observe it. It basically attempts to copy this way of learning by capturing common sense background knowledge of the world and encoding it into digital representations that can be accessed later. The challenge is that such a system must learn these representations in a self-supervised way, using unlabeled data such as images and sounds, as opposed to labeled datasets.
At a high level, I-JEPA can predict the representation of part of an input, such as an image or piece of text, using the representation of other parts of that same input. That’s different from newer generative AI models, which learn by removing or distorting portions of the input, for instance by erasing part of an image or hiding some words in a passage, then attempting to predict the missing input.
According to Meta, one of the shortcomings of the method employed by generative AI models is that they try to fill in every bit of missing information, even though the world is inherently unpredictable. As a result, generative methods often make mistakes a person would never make, because they focus too much on irrelevant details. For instance, generative AI models often fail to generate an accurate human hand, adding extra digits or making other errors.
I-JEPA avoids such mistakes by predicting missing information in a more humanlike way, making use of abstract prediction targets in which unnecessary pixel-level details are eliminated. In this way, I-JEPA’s predictor can model spatial uncertainty in a static image based on the partially observable context, helping it predict higher-level information about unseen regions in an image, as opposed to pixel-level details.
Meta said I-JEPA has displayed a very strong performance on multiple computer vision benchmarks, showing itself to be much more computationally efficient than other kinds of computer vision models. The representations it learns can also be used for other applications without needing extensive fine-tuning.
“For example, we train a 632-million-parameter visual transformer model using 16 A100 GPUs in under 72 hours and it achieves state-of-the-art performance for low-shot classification on ImageNet, with only 12 labeled examples per class,” Meta’s researchers said. “Other methods typically take 2–10 times more GPU-hours and achieve worse error rates when trained with the same amount of data.”
Meta said I-JEPA demonstrates there’s a lot of potential for architectures that can learn competitive off-the-shelf representations without the need for additional knowledge encoded in hand-crafted image transformations. Its researchers said they’re open-sourcing both I-JEPA’s training code and model checkpoints, and their next steps will be to extend the approach to other domains, such as image-text paired data and video data.
“JEPA models in future could have exciting applications for tasks like video understanding,” Meta said. “We believe this is an important step towards applying and scaling self-supervised methods for learning a general model of the world.”
THANK YOU