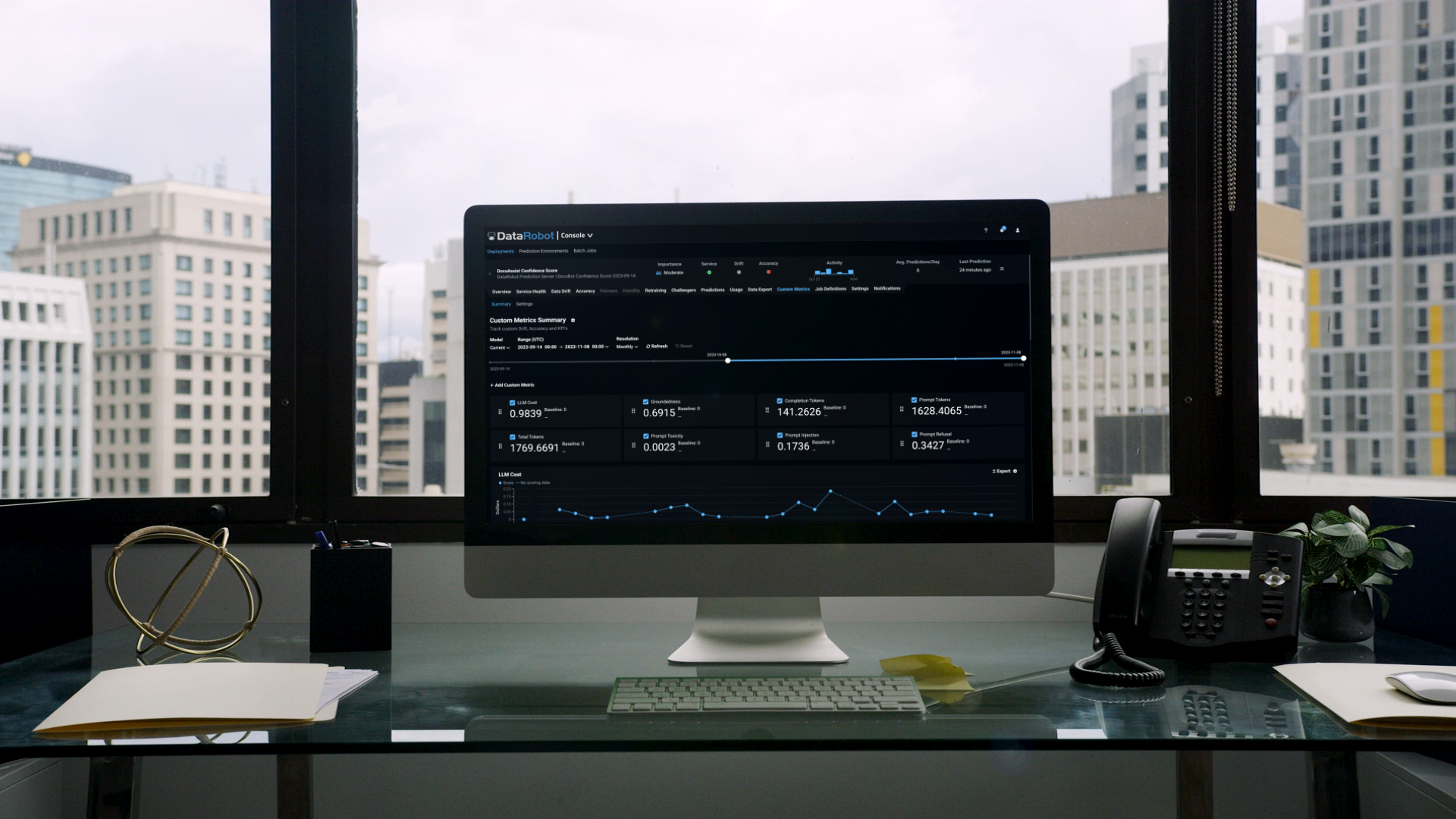
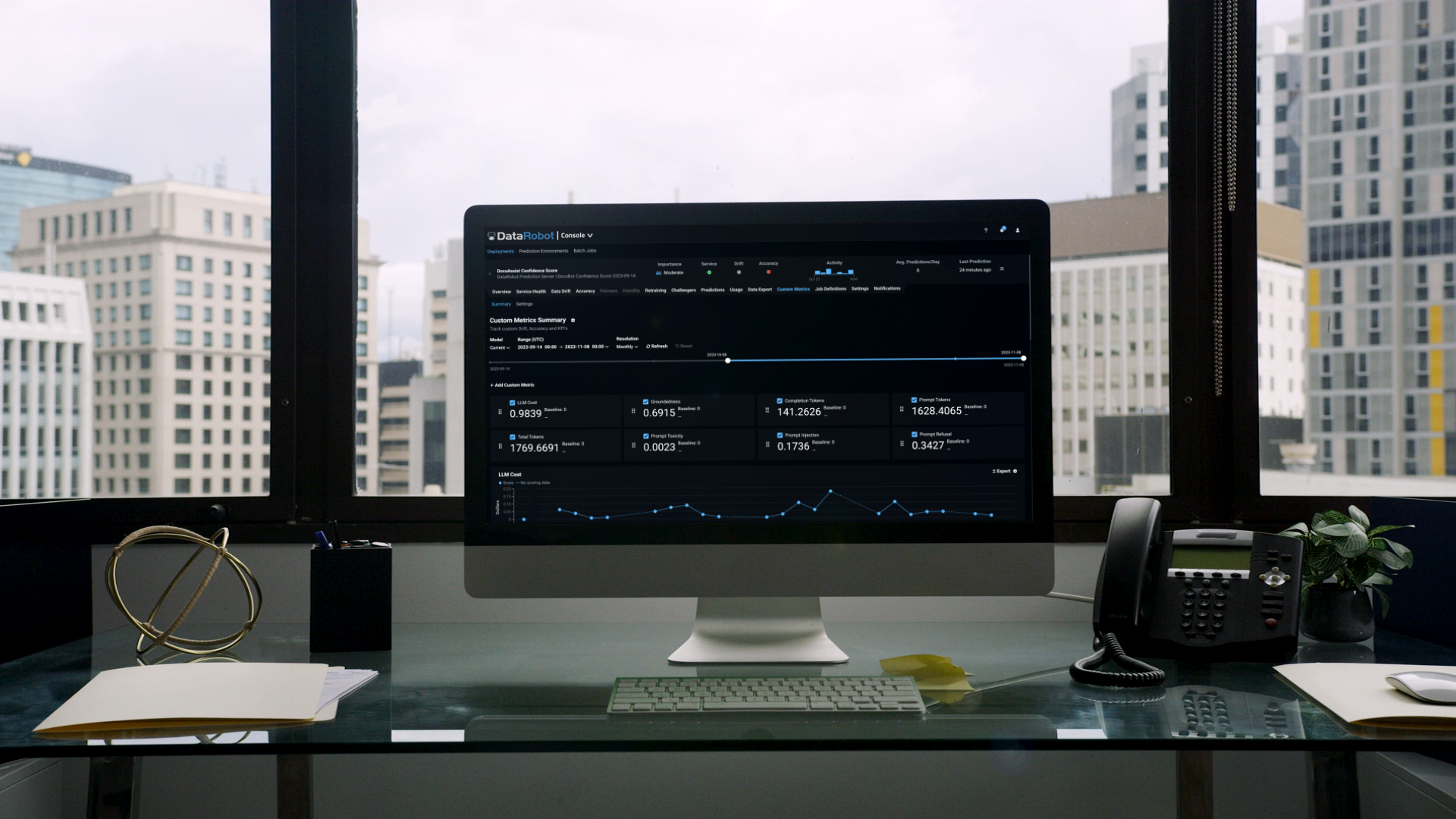
Artificial intelligence startup DataRobot Inc. is keeping up with the surge of interest in generative AI by announcing multiple updates to its enterprise-grade end-to-end AI solution today that will help companies better understand their AI models.
As part of DataRobot’s announcements today, the company added a console for AI observability and monitoring for both generative and predictive AI models, as well as cost performance monitoring. Generative AI developers will be able to test and compare models in a playground sandbox, track assets in a registry and apply guard models.
Using the company’s full-lifecycle platform, AI experts can experiment with, build, deploy, monitor and govern enterprise-grade applications that use artificial intelligence. DataRobot added a host of new capabilities in August to take advantage of the explosive interest in generative AI large language models, such as OpenAI LP’s GPT-4.
As businesses use these AI models, they want to be able to govern their behavior transparently and understand their inner workings so that if something begins to go wrong it can be caught before it affects their customers. Businesses also want to be able to control costs before breaking their budgets. This is where many of DataRobot’s new updates come into play.
“We’ve always been challenging our customers, saying that it’s not enough to build a model, but you need to set up monitoring and an end-to-end loop,” Venky Veeraraghavan, chief product officer of DataRobot, said in an interview with SiliconANGLE. “But with generative AI, I think the issue is a lot more visceral because you’re literally putting text in and getting text out. The narrative in the industry as a whole is worried about prompt injection and toxicity, so there’s a lot more nervousness around what the model’s going to do.”
Up front in the announcements is what DataRobot calls a 360-degree view observability console for the platform and third-party models across different cloud providers, on-premises or at the edge. This is a single point of truth command center where all the information about performance, behavior and health of every AI system that customers have flows, allowing them to realize and take action in real time in case of issues or anomalies.
The solution provides LLM cost and monitoring that can observe and provide cost predictions based on customizable metrics designed for high performance and on-target budgeting. Customers can now see cost per prediction and total spend by generative AI solutions, which permits them to set alert thresholds to avoid exceeding budgets and make decisions about cost-to-performance tradeoffs.
When it comes to getting the models to act in particular ways, the company has released what it calls “guard models.” These are pretrained AI models that observe the behavior of a generative AI and change how it acts, such as suppressing hallucinations, keeping it on topic, blocking toxicity or maintaining a particular reading level.
“As a customer, you can just deploy them as a ‘guard model’ over your current model and just harness this capability,” said Veeraraghavan. “It makes it very easy for someone to build a full-featured application. They don’t really need to make each one as a separate engineering project.”
If one of DataRobot’s preexisting guard models doesn’t suit for purpose, Veeraraghavan explained, a company could build a custom model, for example one that only talks about comic books from the 1980s, and then deploy that over their LLM and go on with their work.
To make comparing and experimenting with LLMs easy, the company announced a multi-provider “visual playground” with built-in access to Google Cloud Platform Vertex AI, Azure OpenAI and Amazon Web Services Bedrock. Using this service, customers can easily compare different AI pipeline and recipe combinations of model, vector database and prompting strategy without needing to build and deploy infrastructure themselves to see what solution might be best for their needs.
Users will also now be able to better track their assets with a unified AI registry that will act as a single system of record that will govern all generative and predictive AI data and models. Veeraraghavan said that the concept behind this was essentially a “birth registry,” because now there are a lot more people working on projects, especially with generative AI, and the more people touching a project means that there are more complex interactions.
“Datasets and the lineage of how you built a model, the parameters, all of those things, so that we know what changed and who changed them,” said Veeraraghavan. “So, one of the things we are announcing with the registry is the versioning of all these artifacts.”
With generative AI bots, there are more “personas,” such as a chatbot that interacts with customers as a domain expert in selling shoes on a website and there might be a different chatbot for internal employees. As a result, developers will want to track the versioning and evolution of these datasets and models to understand recent behavior changes, check modifications or roll them back.
THANK YOU