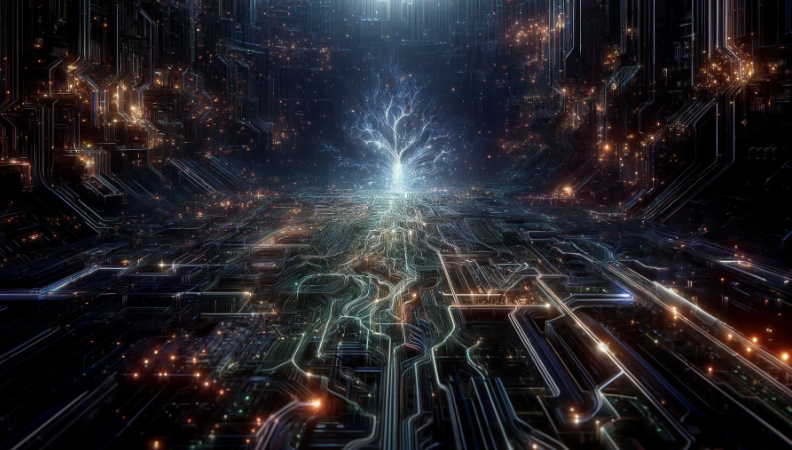
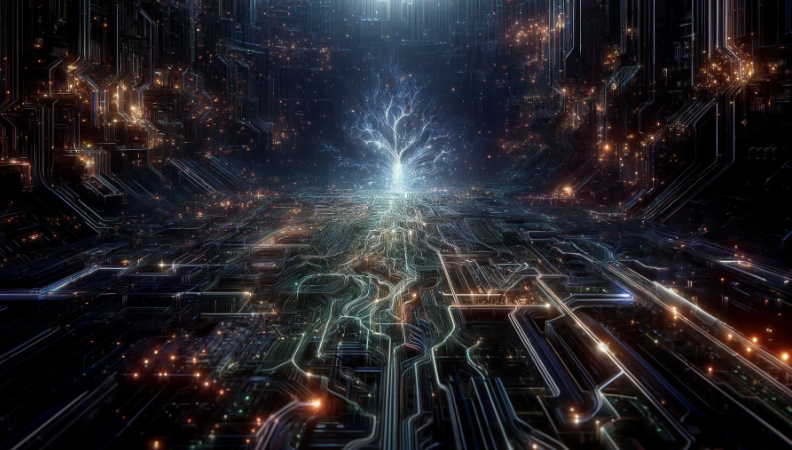
Researchers at artificial intelligence startup Anthropic PBC have published a paper that details a vulnerability in the current generation of large language models that can be used to trick an artificial intelligence model into providing responses it’s programmed to avoid, such as those that could be harmful or unethical.
Dubbed “many-shot jailbreaking,” the technique capitalizes on the expanded context windows of LLMs. They have increasingly grown from processing the equivalent of a long essay to digesting content as extensive as several novels. A context window refers to the maximum amount of text, measured in tokens, that the model can consider at one time when generating a response.
Many-shot jailbreaking involves inserting a series of fabricated dialogues into the input to exploit LLMs’ in-context learning abilities. The feature enables LLMs to understand and apply new information or instructions presented within the prompt itself without any additional training or external data.
The researchers argue that the learning method is a double-edged sword. While making models far more useful, it also makes them susceptible to manipulation through precisely crafted sequences of dialogues. The research reveals that the likelihood of eliciting a harmful response increases with the number of dialogues, raising concerns about the potential misuse of AI technologies.
The discovery could prove critical at a time when the capabilities of AI models such as Anthropic’s Claude 3 become increasingly sophisticated. The researchers said that they decided to publicize their findings due to a commitment to collective security improvement and to accelerate the development of strategies to counteract such vulnerabilities.
The researchers also explored several mitigation strategies. Limiting the context window size was found to be a restrictive solution, potentially diminishing the user experience. More nuanced approaches, such as fine-tuning models to recognize and reject jailbreaking attempts and preprocessing inputs to detect and neutralize potential threats, showed promise in significantly reducing the attack success rate.
“We want to help fix the jailbreak as soon as possible, the researchers wrote. “We’ve found that many-shot jailbreaking is not trivial to deal with; we hope making other AI researchers aware of the problem will accelerate progress towards a mitigation strategy.”
Though some have concerns about issues such as jailbreaking LLMs, what the researchers never tackle is whether broad-scale censorship of LLMs should be further examined. If someone tricks an LLM into telling it how to pick locks — an example used by the researchers — so what? It’s not as if the information can’t be found elsewhere.
It’s also arguably disturbing that researchers at a multibillion-dollar-backed startup seem to be more worried about censoring results than about the actual results provided by LLMs. And the world has seen what the result of focusing on censorship over results can deliver — the much-criticized launch of Google LLC’s Gemini.
THANK YOU