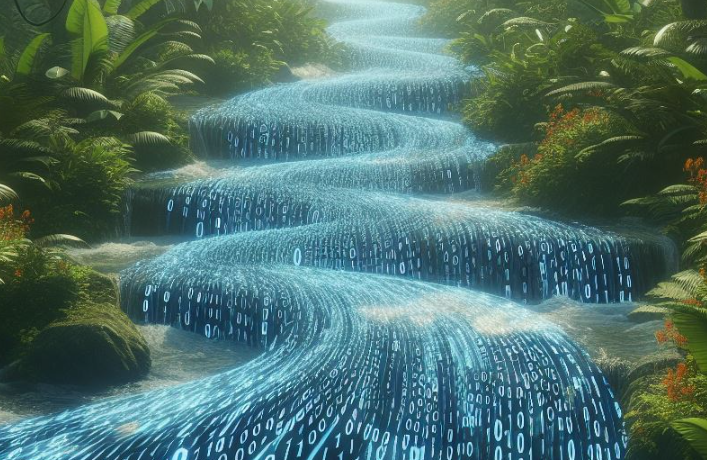
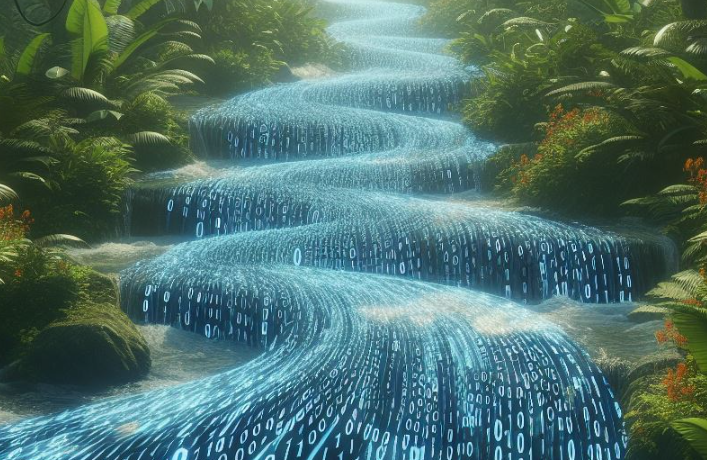
Confluent Inc. today announced expanded capabilities for its managed service for Apache Flink, the open-source big data processing framework.
Unlike the regular open-source Flink, it comes with a novel AI Model Inference feature that organizations can use to clean and process real-time streaming data for artificial intelligence and machine learning applications. In addition, the company announced an auto-scaling cluster type for use cases such as logging and telemetry data without time requirements.
Founded in 2014, Confluent is the company that leads the development of Apache Kafka, the popular open-source big data streaming platform that enables companies to move information quickly from one computing system to another. Its main offering is Confluent Cloud, which is based on Apache Kafka but is faster and more cost-efficient, without the deployment hassles and other overheads, according to the company.
With the launch of Confluent Platform for Apache Flink today, the company is giving customers the ability to self-manage Apache Flink in on-premises and hybrid cloud environments. Apache Flink is an open-source big data processing tool that makes it simple for companies to process large volumes of real-time information. Confluent Cloud customers can not only stream data from one system to another in real time, but also modify and process that data as it’s traveling.
For instance, companies can filter data such as purchase logs that might contain incorrect information, as it’s being streamed from an on-premises system to the cloud. It also supports the merger of multiple data streams into a single stream, so users can enrich their information with data from external sources.
The company made Confluent Cloud for Apache Flink generally available in March. Apache Flink is often used alongside Apache Kafka, but it’s considered fairly complicated to set up and deploy.
According to Confluent, the new service will automate much of the manual work involved in deploying and maintaining the software. As a result, processing real-time data should become less complicated for customers.
Confluent explained that AI Model Inference can be useful for enhancing generative AI workloads such as chatbots and deliver more personalized customer experiences. The issue it tackles is that AI chatbots need fresh, context-rich data to generate more accurate outputs and results.
The AI Model Inference feature in Confluent Cloud for Apache Flink is available in early access now, and is said to provide customers with the ability to analyze the information they process with Confluent Cloud using large language models. According to the software maker, the upcoming feature will lend itself to tasks such as extracting the most important records from a data stream and summarizing text. It makes it possible for developers to use simple Structured Query Language statements to make calls to remote model endpoints, including OpenAI, AWS Sagemaker, Google Cloud’s Vertex AI and Microsoft Azure’s OpenAI Service, so as to orchestrate data cleaning and processing tasks in real time.
Confluent said this capability can vastly simplify the AI development process, allowing developers to work with the more familiar SQL syntax to interact with their models, instead of relying on more specialized tools and programming languages. By streaming more data to generative AI models in this way, the company said, it will enable more accurate, real-time decision making that leverages fresh, contextual data.
It also supports better coordination between data processing and AI workflows to improve efficiency and reduce operational complexity, the company said.
There are more general advantages to be had from combining Apache Kafka with Apache Flink too, with Confluent saying customers will benefit from streamlined support, better integration and compatibility with the two technologies.
As for the new auto-scaling clusters, they’re aimed at providing greater cost-efficiency for high-throughput use cases that have more flexible latency requirements. For instance, many organizations use Confluent Cloud to produce and consume lots of logging and telemetry data. These use cases have high throughput but the latency requirements are more relaxed, as such streams are typically fed into indexing or batch aggregation engines.
The auto-scaling clusters are powered by elastic CKUs, which specify the maximum ingress, egress, connections and requests supported by a cluster. This enables them to auto-scale to meet demand with no manual sizing or capacity planning required, so organizations have an easier way to minimize their operational overheads and optimize costs, only paying for the resources they need.
The new auto-scaling clusters are available now in early access in select Amazon Web Services regions.
THANK YOU