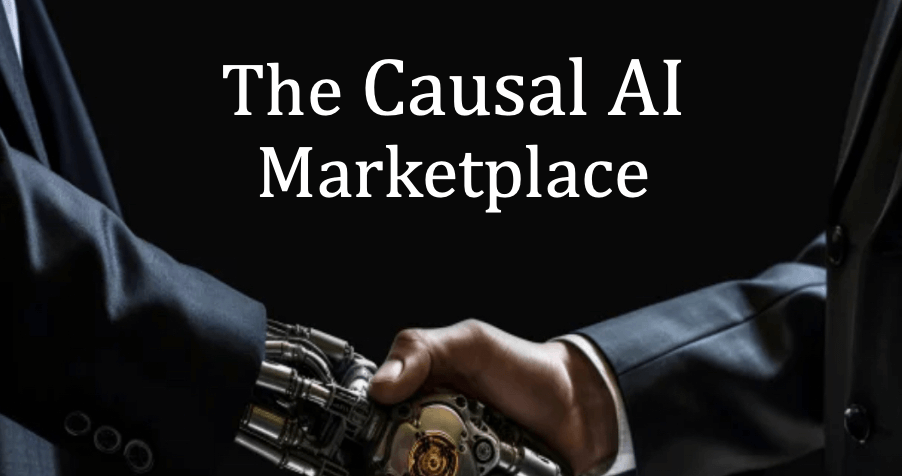
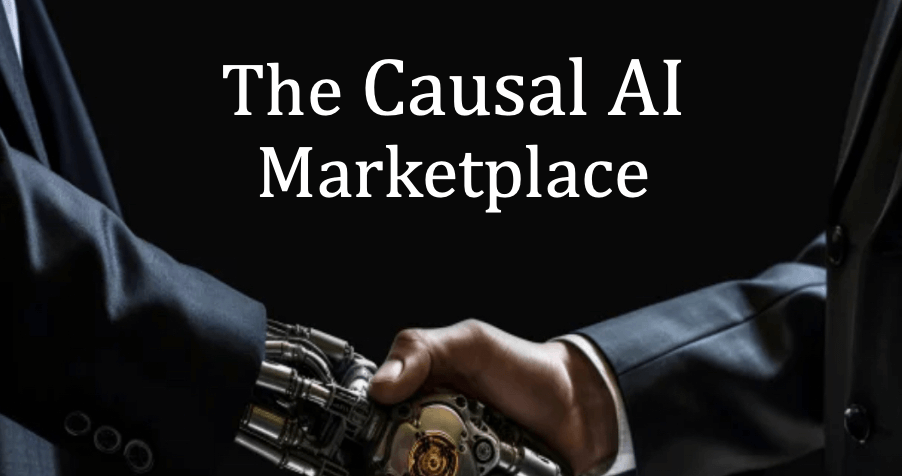
There is a missing ingredient in today’s artificial intelligence, which, when added, will make AI a truly indispensable partner in business and increase return on investment over the long haul.
The missing ingredient is causality and the science of why things happen.
Here at theCUBE Research, we are focused on informing you about the latest developments shaping AI’s future and helping you prepare now rather than later.
This research note is the first in a series exploring the emerging impact of causal AI. It will focus on the growing marketplace of products and services related to causal AI. Stay tuned for future notes that will progressively explain what you need to know and why.
We believe that the rise of causal AI will play a vital role in enabling new high-ROI use cases within existing AI systems and future AI architectures. Overall, we are confident that integrating agentic AI, causal reasoning and interactive explainability, supported by a network of large language models and small language models, will define the next frontier of AI in business.
These future-state architectures will orchestrate an ecosystem of collaborating AI agents and models that will help humans problem-solve and make better decisions. They will also continuously teach each other to improve outcomes learned from real-world, outcome-based experiences. Causal AI will play a critical role in these architectures.
Furthermore, in the near term, the causal toolkit will help businesses progressively improve the outcomes rendered from today’s correlative AI models — generative AI, LLMs, predictive AI and the like — which operate based on statistical probabilities but often lack any genuine causal understanding. As a result, they cannot truly understand the dynamic nature of how a business operates, creates value and engages the world.
Despite that, today’s LLMs and generative AI are as incredibly impressive as they are complex. However, those in the middle of implementing causality in AI would tell you that it’s complex on a whole other level, involving the algorithmic application of some super-eloquent math and statistical theory.
Fortunately, as we will discuss, an increasing number of software companies is making significant strides in simplifying the use of causal AI for the masses. So, despite the almost unimaginable complexity behind the scenes, incorporating causality into today’s AI environment may be simpler than we realize and will likely become even easier over time.
For the purposes of this research note on the marketplace, let’s define causal AI as a branch of machine learning that emphasizes understanding cause-and-effect relationships rather than solely processing patterns in data. Though traditional AI models typically forecast or predict potential outcomes based on historical data, causal AI goes a step further by elucidating why something happens and how various factors influence each other.
In addition, causal AI can understand not only statistical probabilities but also how those probabilities change when the world around them changes, whether through intervention, creativity or evolving conditions. This is crucial because non-causal models perform well only if the future resembles the past. However, they struggle in a world of dynamic conditions, which is an inherent attribute of business.
Simply put, people are naturally inclined to understand “why” before taking action, making them causal by nature. It’s important for AI to also be causal by nature. The impact of causal AI on business could be enormous, as businesses rely on people to make decisions and act. After all, businesses don’t do things; people do.
There is little doubt that AI represents the transformative force of our time. This is why nine in 10 businesses are accelerating their spending on AI, with the vast majority declaring it their No. 1 investment priority. As a result, many organizations have already reported gains in productivity nearly three times above the overall 3% reported by the Bureau of Labor Statistics in 2023.
Though today’s AI capabilities are super-impressive, AI is only in its infancy.
To start, AI is an umbrella term. Many of today’s deployments are expert systems on steroids — the programmatic transfer of knowledge into rules-based systems. They do not employ the more capable algorithmic “brains” known as machine learning.
Of those who have, 80% rely on the least sophisticated techniques and can only perform narrowly defined tasks. This will change in the years ahead.
The global market for machine learning platforms and tools is projected to see substantial growth through 2030. According to estimates from theCUBE Research, the market is expected to reach $420 billion by 2030, with a compound annual growth rate of 35%. Cloud-based ML services, explainable AI, agentic AI and causal AI are anticipated to be major contributors to this expansion.
New Enterprise Technology Research survey data among 1550-plus decision makers supports the rapid adoption of ML and AI technologies within enterprise accounts. Half are already adopting ML, representing the highest spending trajectory among all technology categories.
This investment trajectory will likely continue as new tools and platforms democratize ML’s utilization across broader skill sets and, importantly, speed up the delivery of new ML advancements to the masses.
Despite the increased investment in AI, businesses still face limitations with today’s AI technology. According to a 2023 survey conducted by Rexer Analytics, fewer than half of enterprise machine learning projects make it into full production because of their inability to adapt to changing conditions, as well as a lack of trust and explainability.
However, there’s good news. Other studies, including a May 2024 survey by Dataiku, show that nearly seven in 10 AI projects that have made it into production experience a positive return on investment.
The emerging causal AI marketplace aims to address the limitations of current LLMs and generative AI solutions. This is particularly crucial for high-ROI use cases that depend on root cause detection, decision intelligence, problem-solving and autonomous action. AI professionals working on these cases recognize that integrating causality into the AI model lifecycle is essential for accurately modeling the dynamic business world and improving AI trust, transparency and explainability.
The marketplace is speaking louder and louder.
A survey of 400 senior AI professionals provided by Databricks Inc. showed that among AI pioneering companies, 56% were already using or experimenting with causal AI. In addition, among the total population of the survey, causal AI was ranked as the No. 1 AI technology “not using, but plan to next year.” The study reported that 16% are already actively using causal methods, 33% are in the experimental stage, and 25% plan to adopt. Overall, seven in 10 will adopt causal AI techniques by 2026.
We are also seeing more and more companies go public with their causal AI success stories, pilot projects, and lessons learned. Many of these are highly encouraged by its game-changing potential, with some already reporting quantified ROI.
For example:
This surge in interest has driven the 2024 Gartner AI Hype Cycle to predict that causal AI will become a “high-impact” technology in the two- to five- year timeframe, a shift forward from the 2022 AI Hype Cycle that had it in the 5-10 year timeframe.
As per Gartner, causal AI is considered “crucial” for scenarios where it’s necessary to do more than just predict an outcome, but also understand the reasons behind the outcome and how to enhance it. Gartner also highlights that causal AI is essential for developing time-sensitive use cases that must function independently when human intervention is not possible. This entails AI understanding how to take action and the repercussions of its actions.
Furthermore, Gartner believes that the growing demand for increased trust, transparency, and explainability in AI results is fueling a rising interest in causal AI techniques because of their capacity to comprehend how outcomes are produced. Gartner concludes by stating that “The next step in AI requires causal AI.”
TheCUBE Research’s perspective on the report is that decision intelligence and causal AI should be combined in their hype cycle because decision intelligence without causality is simply business intelligence. We would also like to add that implementing causal models can be incremental, where no “rip and replace” is necessary. Lastly, the broadest application of causal AI is likely to occur within the context of SLMs (smaller, specialized, secure and sovereign) and as a component that powers agentic AI networks. However, we fully agree with Gartner on the critical importance of causal AI in future AI systems.
The resulting commercial marketplace is somewhat ill-formed, but we expect it to solidify and rapidly expand from here. The consensus view of six independent market studies complied by theCube Research indicates a projected 41% compound annual growth rate through 2030 to around a $1 billion marketplace.
Our view is that when assessing this marketplace, we must consider the following:
For these reasons, we believe the aggregate market impact of causal AI on business will be far greater than the $1 billion marketplace indicated by today’s studies. In fact, we would not be surprised to see a similar dynamic to how generative AI well outperformed its initial market growth projections and became the darling of the AI world.
It’s worth noting that numerous companies are already in this market, with hundreds, if not thousands, of customers. They are committed to providing both general-purpose and domain-specific causal AI tools and platforms, making AI causality’s high-ROI potential accessible to everyday businesses.
For example:
These are just a handful of solution providers in the marketplace today, and we fully anticipate that many more new entrants will soon emerge.
Causal AI is a significant advancement in the ongoing progression of AI. The current correlative-based designs have limitations that will eventually hinder the development of new innovations. As Microsoft Research has recently stated, “Causal machine learning is poised to be the next AI revolution.” Thugh it may not be a revolution, it is certainly necessary and inevitable. It’s just a matter of time before it becomes mainstream.
Perhaps the time is now to start preparing for this new frontier in AI. As we have witnessed in past technological evolutions, some will lead, some will lag and some will eventually fail. It’s never too early to start the evaluation process and run experiments.
We’d recommend you:
We recommend watching the recent Breaking Analysis with Dave Vellante segment titled From LLMs to SLMs to SAMs: How Agents Are Redefining AI, which provides a holistic perspective on the value of causal AI. You can also listen to this on your favorite podcast channel.
Also, if you are a user of OpenAI’s ChatGPT, you may also find our recent research note OpenAI Advances AI Reasoning, But The Journey Has Only Begun of interest.
Finally, stay tuned for the next in this series of research notes on the advent of causal AI.
THANK YOU