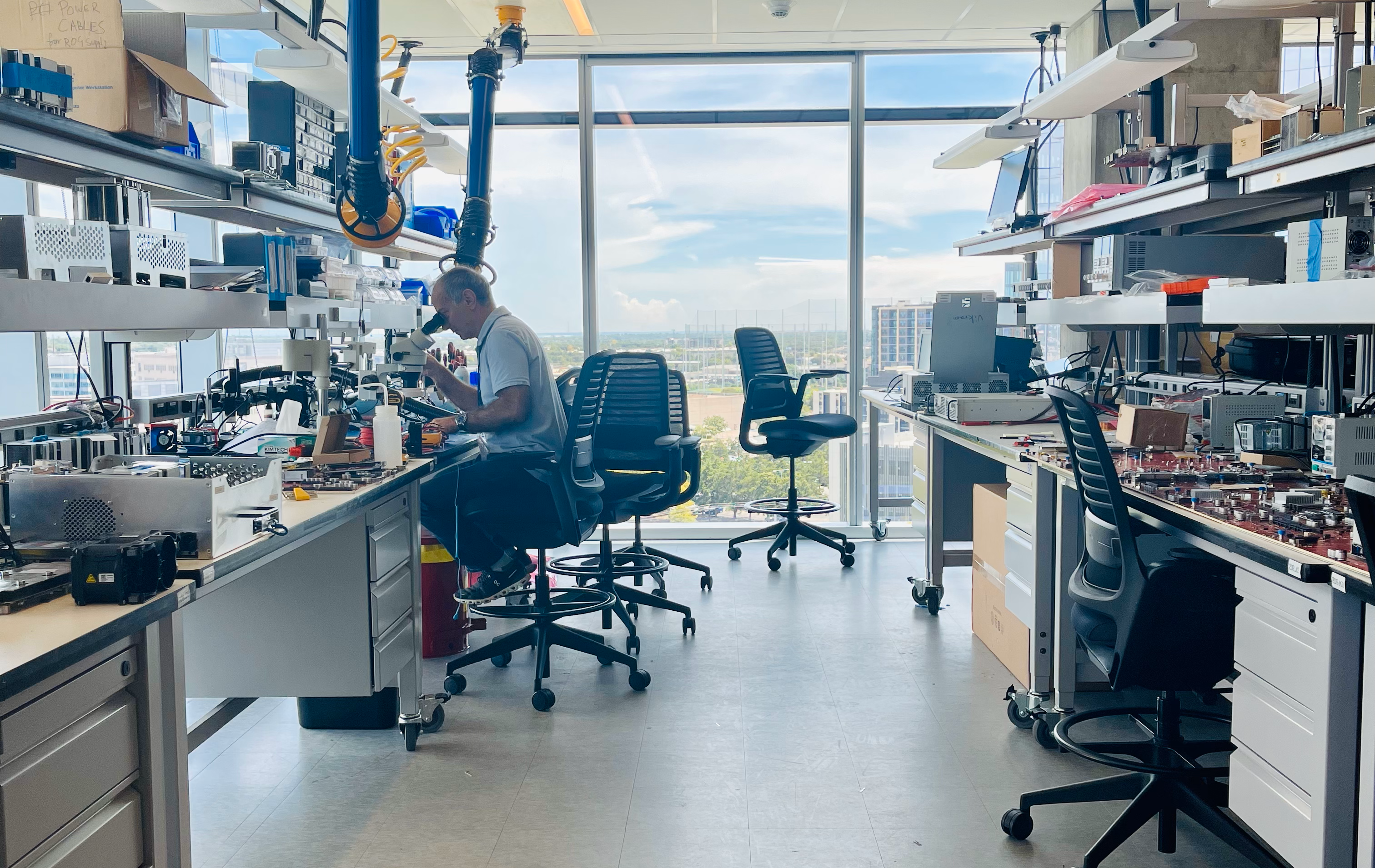
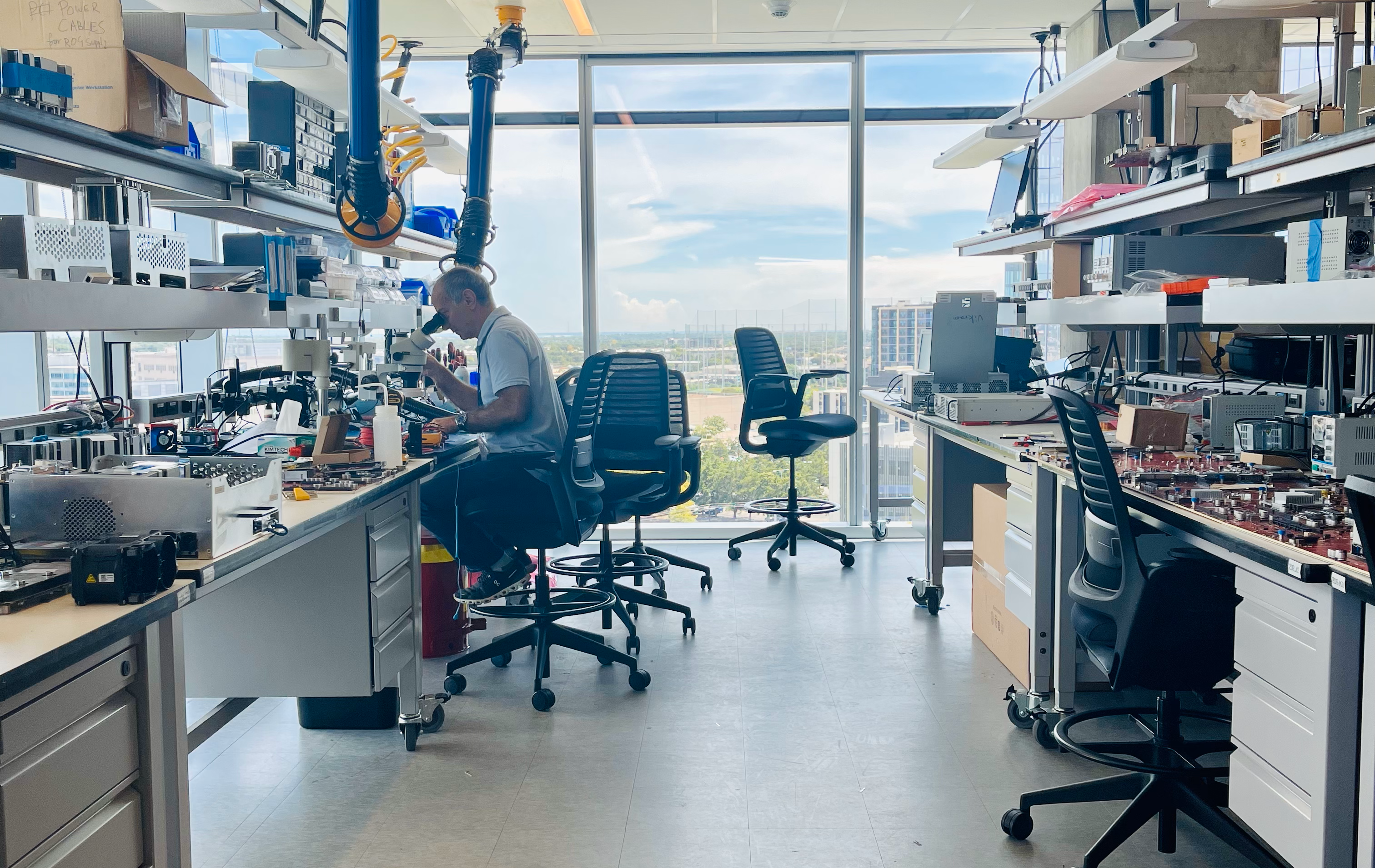
Nestled on the ninth floor of an unassuming building in an affluent area of Austin, Texas dubbed The Domain, is one of the world’s most influential research labs powering modern artificial intelligence.
SiliconANGLE got an exclusive, behind-the-scenes tour of Amazon Web Services Inc.’s Annapurna Labs, where the cloud powerhouse does the secretive work of designing and testing the upcoming generations of itsAI accelerator Trainium and custom cloud compute Graviton chips.
Upon arrival, Martin was greeted in the lobby and escorted to the Labs, a highly restricted area even for Amazon.com Inc. employees. After being checked in, given a temporary visitor’s pass and a handful of Jolly Ranchers, Martin met with Rami Sinno, director of silicon engineering at AWS. Joining AWS from Arm Holdings PLC, Sinno brought large-scale project management experience to the project since its inception, detailing the challenging work of recruiting a team of talented engineers for the Trainium chip, a completely stealth initiative at the time.
“We formed a team whose mission was to deliver the best machine learning accelerators at cloud scale,” Sinno said. “This was exciting because it was in the early days of high-performance and low-cost AI servers at scale.”
The team started the architecture from a blank sheet of paper, using the customary Amazon method of thinking backwards from what customers would want to come up with the best technology for their needs. “Our big bet on this new architecture paid off as we now have multiple generations of Inferentia and Trainium chips in the data center,” he said.
The fruits of the lab will be prominent at AWS’ upcoming annual conference, re:Invent, in Las Vegas Dec. 2-6. Considered the largest cloud computing conference of the year, it’s expected to focus, not surprisingly, on AI. AWS is widely anticipated to debut new AI chips at the event in hopes of seizing momentum in AI from others such as Google LLC and Microsoft Corp., whose efforts have been more prominent than Amazon’s to date.
Amazon has garnered some criticism for appearing to lag in AI, but theCUBE Research Principal Analyst Shelly Kramer disagrees. “Amazon is doing some impressive things with AI, and forging strategic partnerships that are already driving value,” she said. “What Amazon needs to do a better job of is more effectively telling those stories.”
A Tranium chip. Photo: Amazon
The Austin facility isn’t Annapurna’s largest lab space. That’s in Tel Aviv, Israel; employees also work out of another location in Toronto, Canada. This lab space is dedicated to housing hardware and software development engineers for machine learning servers and for the Trainium and Inferentia, AWS’ AI chips. On the same floor, engineers test and develop the software for Graviton.
Annapurna’s AI chip operation and its research play a critical role in Amazon’s strategy to maintain a competitive edge in AI. The company has fallen behind its big tech rivals when it comes to AI-powered smart assistants, with a generative AI version for its Alexa assistant reportedly repeatedly delayed. Competitors such as Google and Apple Inc. have fielded smarter AI digital assistants in the past few months.
AWS unveiled its most recent generation of Trainium2 chips at re:Invent 2023, opening up a new era of AI training that enables training new models that require less money and power than before. The new chips deliver up to four times faster training than the first generation of Tranium, making foundation model and LLM training faster, while also providing up to twice the energy efficiency.
Trainium2 is already reportedly being tested by Anthropic PBC, an OpenAI competitor. The chips are currently being used to train the company’s next-generation Claude large language model family, which takes a great deal of time and compute.
“AI training in particular takes a long time,” Sinno explained. “It doesn’t take a few seconds to train a model. It’s measured in hours, days and even weeks — uptime is extremely important for customers. You can’t afford to have effects with servers dropping while you are doing training. So, we spend a lot of energy from early design phases to scaling data center quality for uptime for our customers.”
Inside the lab, a mini-data center. Photo: Amazon
In the Austin lab, AWS also integrates, tests and prototypes the hardware that the chips are integrated with, as well as the motherboards and racks that the custom silicon interacts with. This creates a low-cost solution for developing and testing everything that goes into the data center. Centralizing efforts on a single floor of the building means an expedited development process, rapid prototyping and testing.
There are two lab rooms at the Austin location, flanking each end of the building. The “Quiet Lab” is where near-final products are tested. Chips and chipboards are connected together so that software engineers working remotely in Canada and Israel can run diagnostics.
Through two sets of doors is the Quiet Lab, a small vestibule acting as a necessary transition from the bustle of cubicles and conference rooms to the orderliness of the laboratory. There were surprisingly few people working in the lab, lined with rows of stations that were filled with hardware components for active testing. Each had shelves stretching nearly to the ceiling, holding spare parts, a bounty of tools and the private network plug-ins for running virtual tests with offsite engineers.
Surrounded by floor-to-ceiling windows overlooking The Domain, the Quiet Lab seems an inspiring place to build. The layout affords the space needed to test end-to-end, minimizing the back-and-forth shuffling of components to other engineering teams on both the hardware and software sides. From 3D printing to Dremel power tool kits, portions of the lab could be mistaken for a hobbyist’s workshop.
“We are still in the early days of machine learning,” Sinno said. “Because we are early, it is imperative for the design team to be able to have a very fast cadence in the products that we deliver to our customers. Because if it takes our team five years to deliver our server, there might be two generations of new AI workloads and our server cannot hit that mark.”
Detailing the equipment and overall setup of the Quiet Lab, Sinno emphasized the importance of having a fully equipped space. By enabling real-time collaborations across teams both onsite and offsite, he said, AWS is able to shave months, even years, off the development time. It’s a competitive edge for bringing products to market faster.
“I’m a big fan of Amazon’s real-time collaboration workflow style and this is an example of why it’s valuable,” said Kramer. “In today’s rapidly moving tech ecosystem, time is money – it’s a no-brainer that speeding development time plays a significant role in product success.”
The “Loud Lab” is where AWS is testing out its next big thing. It’s called the Loud Lab because numerous fans are required to keep machines cool. Earplugs are necessary to prevent hearing loss or damage, which made for an almost comical Q&A effort as Sinno shouted replies to questions during the tour.
The layout of the Loud Lab almost mirrors that of the Quiet Lab, though human work in the room is limited given the conditions needed to maintain the machines. Initiated during COVID-19 lockdowns, the lab buildout not only had to consider the immediate demands of working in a pandemic but future considerations for employees as well as the machines and the building in which they reside. The result is a rapidly evolving lab space intended to address the pressing demands of a hyped-up AI scene, with enterprises anxiously seeking a return on investment.
Sinno couldn’t share much publicly about the Loud Lab, but these forward-looking initiatives speak to the palpable excitement of a team working on the brink of something big. AWS appears to be rethinking nearly every aspect of the stack in order to drive the innovation necessary to realize the sci-fi dreams of artificial intelligence.
Amazon outlined a broad AI strategy last year, including a partnership with Anthropic along with a $4 billion investment in the AI startup this month following $2.75 billion in May. Amazon has also widened the scope of its AI offerings, including bringing more advanced foundation models into its managed generative AI app service Bedrock for training and deployment showing that its plans are not slowing down.
All four generations of Graviton set in a row. Image: Amazon
While Trainum provides high-performance AI and machine learning workloads, the Arm-based Graviton family of chips represents AWS’ pinnacle for energy-efficient high-performance custom chips for its Elastic Compute Cloud workloads.
Ali Saidi, senior principal engineer at AWS, said the primary hardware design and some firmware for Graviton is done in the Annapurna location in Israel, but the focus of the Austin lab is on software. There’s a lot of remote collaboration that happens between the teams to make the whole chip come together.
Graviton4, released in July, provides up to 30% better performance and 75% more memory bandwidth than Graviton3, released in May 2022. The Graviton platform started in 2018 with Graviton1, with the foundational technology behind the EC2 platform, called Nitro. It’s a lightweight hypervisor that allows for all the virtualization of compute, storage, memory and networking. At the time AWS wanted a fully integrated platform.
The cadence by which AWS has produced custom silicon in the Graviton family has been a rapid drumbeat – a rate of about one and a half years per generation. To keep that up, Saidi said, AWS has tightly integrated the hardware team and the software team from day one.
“We’re working on the software we’re going to use to deploy from the start,” said Saidi. “We have these big emulators and simulators before the physical chip that allow us to run the actual software on it. So, we can run a virtual machine in an emulator attached to a real Nitro card doing their normal transactions to prove that everything is working well and refine the software and that process. That lets us move really fast.”
With this level of integration between teams, Saidi said, teams have been able to take a chip from the planning stages and get it into one of their development data centers in a matter of weeks. “That’s super-powerful,” he added.
The evolution of Graviton in AWS cloud has greatly boosted the overall capability and availability of Amazon’s cloud compute. “We went from nothing in 2018 to over 2 million Graviton chips in our data centers,” said Saidi.
At launch, Amazon said the Graviton4 chip would be available in EC2 as part of RC2 R8g instances, which allows customers to run improved execution for high-performance databases, with improved memory for big-data analytics. Graviton4 chips are also part of the X8g memory-optimized instances, which the company said are the most energy-efficient to date, with the best price-performance of any comparable EC2 Graviton instance. Compared with the previous generation, the new instances offer triple the memory and virtual CPUs, and include double the Elastic Block Store bandwidth and double the network bandwidth.
At re:Invent, the company is expected to announce greater availability for Graviton4 chips across even more instance types as the company deploys the new chips widely across its compute cloud. AWS is also expected to announce the upcoming launch of its next generation of custom Trainium2 chips, which will be available in new Amazon Elastic Compute Cloud, or EC2, Trn2 instance clusters.
Given the company’s AI trajectory and the rapid growth of large language models, there will most likely be an emphasis on how new instances can be used to power applications using LLMs and support vector databases to fuel them. As better software and hardware design rolls out to data centers, the company will also likely unveil new energy efficiency metrics on its hardware given how its full-stack design helps reduce power consumption.
Not only does the Austin lab provide software and testing for AI chips, it also positions itself to test and trial entire data center-ready server systems before rolling them out for real. This allows Annapurna to understand how chips will work in the field alongside the actual equipment it will run on and provide diagnostics, testing and opportunities for further refinement.
Combined with the company’s AI chip design capabilities, this makes Annapurna Labs all the more central to Amazon’s high-stakes AI strategy. At re:Invent, customers will find out whether that’s enough to steal a march on the likes of Microsoft and Google.
THANK YOU