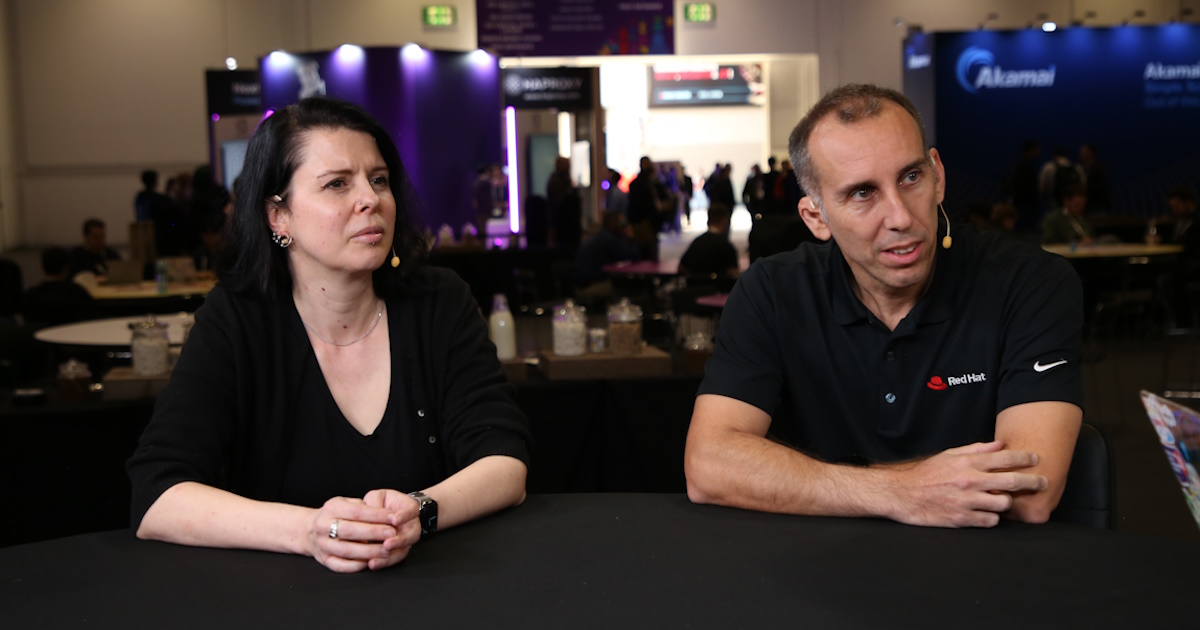
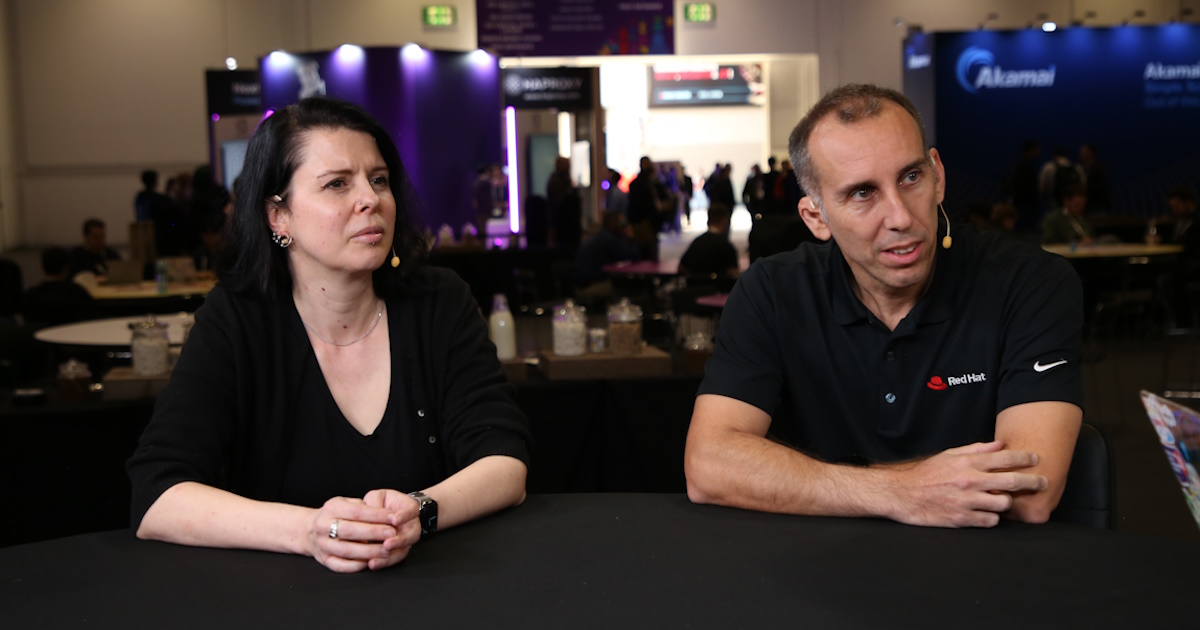
Kubernetes is emerging as a critical backbone in the race to operationalize artificial intelligence at scale, even as its stateless foundation collides with AI’s deep reliance on data context and state.
As AI moves beyond the lab into real-world enterprise systems, the demand for distributed, resilient and orchestrated infrastructure is transforming cloud-native strategies. Organizations are rethinking everything — from how they deliver AI applications to where they run them, often balancing edge deployment, platform engineering and sustainability with the need for flexibility and cost control, according to Holly Cummins (pictured, left), senior principal software engineer for Quarkus at Red Hat Inc.
Red Hat’s Holly Cummins and Vincent Caldeira talk with theCUBE about Kubernetes and AI.
“All of these patterns, they almost look like the same patterns that we figured out with Kubernetes,” she said. “When we were doing cloud-native computing, the mental model that we had and the paradigm was, we want to be stateless. The more stateless we can make the more things, the more scalable we’re going to be … the more cloud-native we’re going to be.”
Cummins and Vincent Caldeira (right), chief technology officer for APAC at Red Hat, spoke with theCUBE’s Savannah Peterson and Rob Strechay at KubeCon + CloudNativeCon Europe, during an exclusive broadcast on theCUBE, SiliconANGLE Media’s livestreaming studio. They discussed how Kubernetes is playing a pivotal role in scaling and operationalizing AI, despite challenges in aligning its stateless architecture with AI’s stateful requirements. (* Disclosure below.)
Organizations are no longer satisfied with theoretical AI capabilities — they need real-world performance that balances cost, sustainability and security. This is where Kubernetes is proving to be essential, not just as an orchestrator, but as a foundation for experimental and enterprise-grade AI deployment, Caldeira explained.
“To support our customers, we have to make sure that they are able to run in a cloud-native and distributed way, data Stacks and enterprise grade as well,” he said. “There is this concept of resilience that is extremely important for people to manage. Kubernetes is very good at this, at orchestration, resilience, recovery, but it’s not necessarily natively good at handling the recovery of the state.”
Kubernetes remains central to the effort of democratizing AI infrastructure, especially at the edge. Enterprise interest is shifting from massive centralized models toward lightweight, domain-specific tools that can be iterated and optimized. This leads not only to better performance but also to more manageable costs and energy footprints, according to Cummins.
“What we’re actually doing is we’re seeing better results with lower costs which is really cool,” she said. “For example, with something like a smaller model I is often you know it’s faster, the costs are lower and it’s more tailored to your domain. It actually gives you better results.”
Red Hat sees the CNCF as a vital force in standardizing operations across cloud-native ecosystems. By supporting projects such as Kepler for energy efficiency metrics, Red Hat helps drive convergence on shared benchmarks. Without common standards, meaningful comparisons — and real progress in sustainability and performance — would remain fragmented and inconsistent across the stack, Caldeira added.
“To me, the biggest role of the CNCF is to help us converge on standards, way to actually operate,” he said. “Having, for example, a single CNCF product that is able to provide me energy efficiency of the system and promoting this across the stack is a supremely important aspect of it. If we all have different metrics to compare how efficient we are, we are never going to converge.”
Here’s the complete video interview, part of SiliconANGLE’s and theCUBE’s coverage of KubeCon + CloudNativeCon Europe:
(* Disclosure: Red Hat Inc. sponsored this segment of theCUBE. Neither Red Hat nor other sponsors have editorial control over content on theCUBE or SiliconANGLE.)
THANK YOU