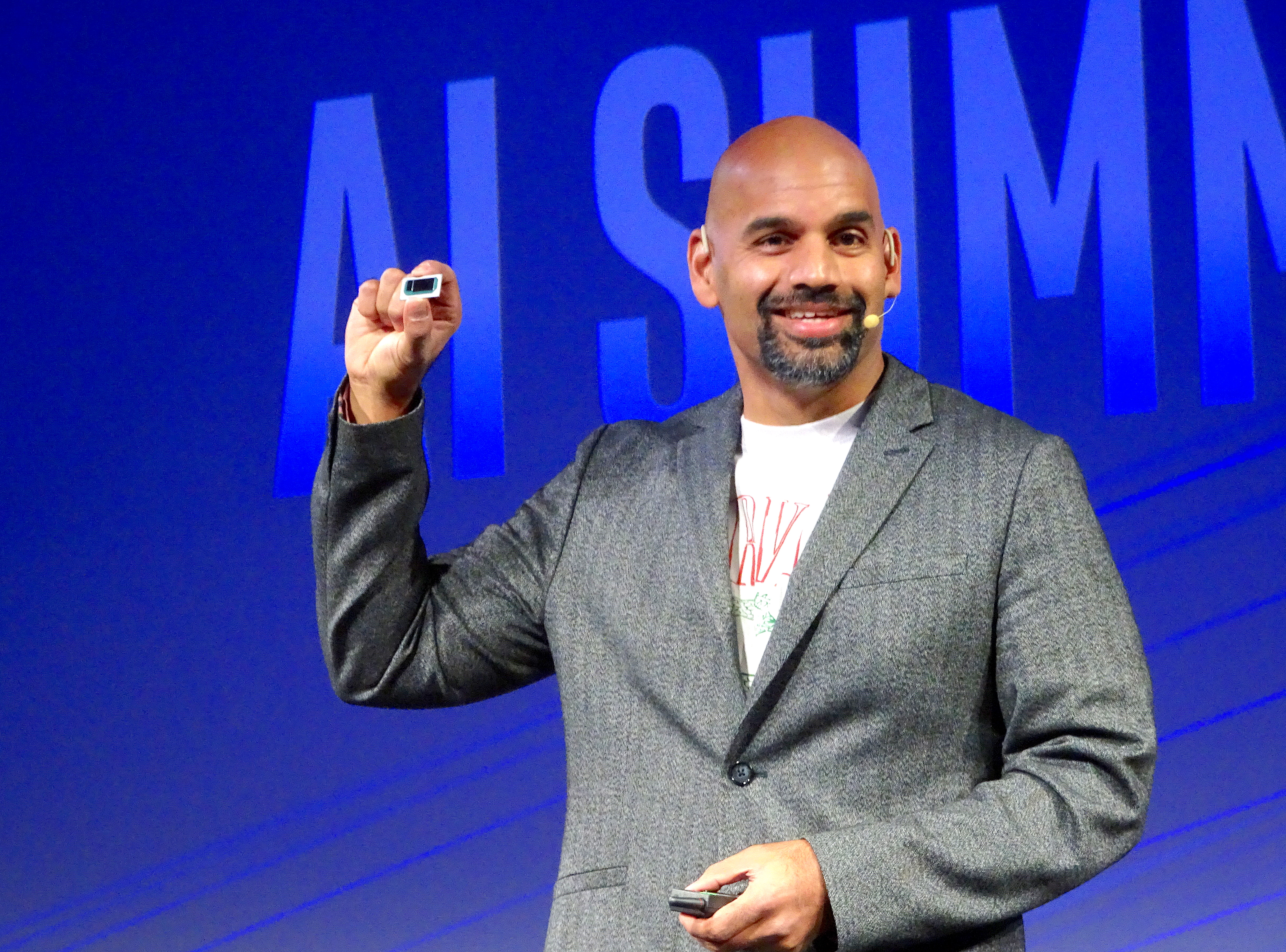
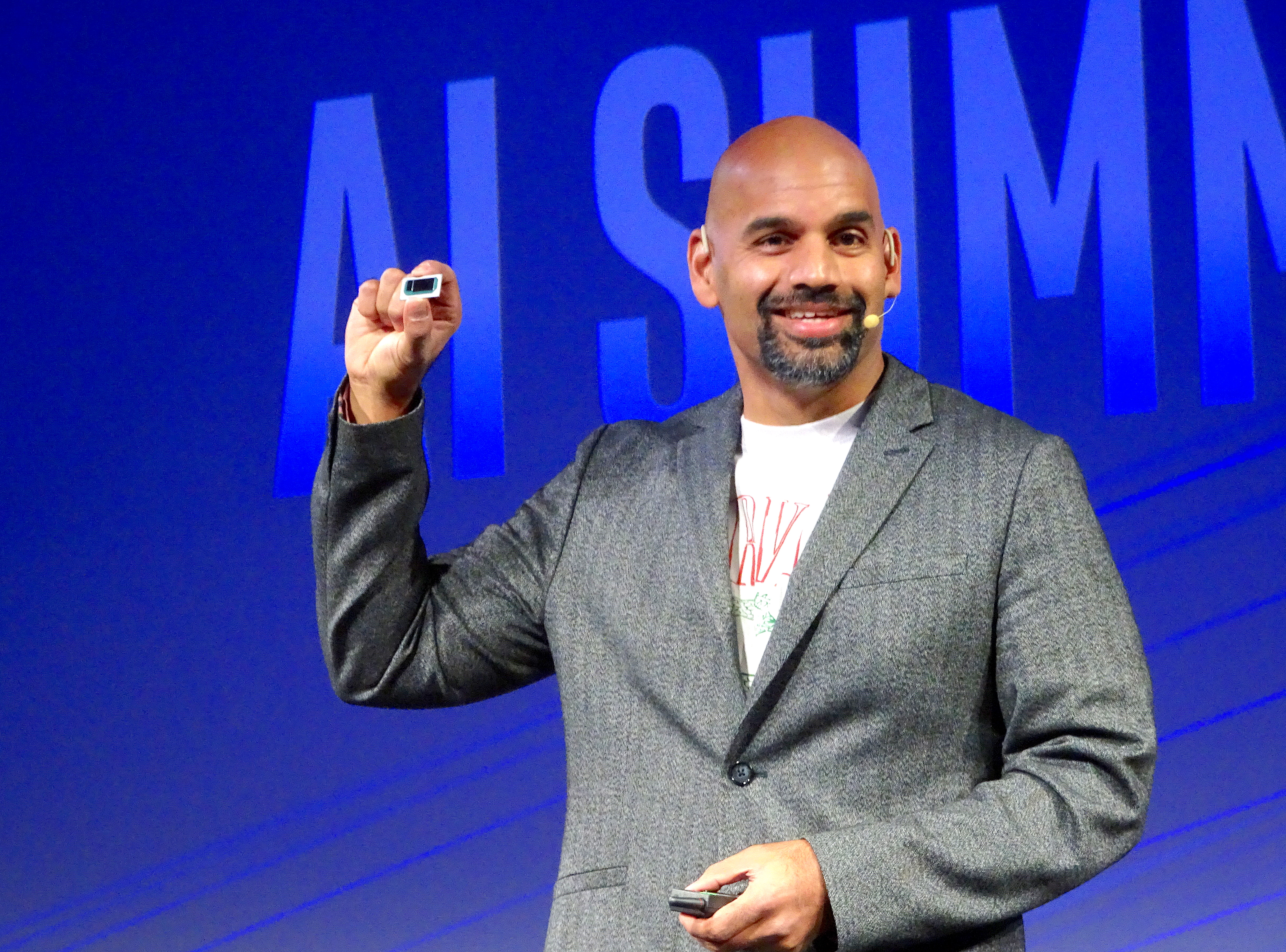
Intel Corp. today introduced a trio of chips for training and deploying artificial intelligence models that will allow it to step up the competition against Nvidia Corp., whose graphics processing units dominate the market.
The chips, unveiled at a press event in San Francisco this morning, grew out of Intel’s 2016 acquisitions of two machine learning companies, Nervana Systems and Movidius Ltd. Collectively they represent Intel’s first big reveal for specialized AI chips that expand beyond its mainstream Xeon and Core chip families.
According to Naveen Rao (pictured, with one of the new Nervana chips), a Nervana founder who’s now corporate vice president and general manager of Intel’s Artificial Intelligence Products Group, the power the processors bring is critical because “we’re reaching a breaking point” in computational hardware and software. That’s a reality that’s also driving a new generation of AI-oriented chip startups recently, including one called Blaize Inc. just today.
The product that takes the most direct aim at the GPU maker is the Nervana NNP-T1000 neural network processor. It’s an integrated circuit optimized for the hardware-intensive task of training AI models with sample data. This process, which is necessary to ensure models produce accurate results, is today carried out using Nvidia chips in the vast majority of AI projects.
Intel said in an August preview that the NNP-T1000 can manage up to 119 trillion operations per second. The company won’t ship the processor on its own, but rather as part of accelerator cards that enterprises will be able to plug into their servers. The cards are designed such that a large number of them can be linked together relatively easily, which Intel said allows them to support even supercomputer-scale AI training workloads.
Intel has gone off the beaten track with the NNP-T1000. Instead of handling production at its own facilities, the company has opted to entrust the task to TSMC Ltd., an external semiconductor maker. The 27 billion transistors that make up the NNP-T1000 are fabricated using the Taiwanese firm’s 16-nanometer manufacturing process and are organized into 24 processing cores.
The second addition to the company’s AI chip line is the Nervana NNP-I1000. Also delivered as an accelerator card, it’s optimized not for training but rather inference, the calculations that models perform on live data once development is over and they’re deployed in production.
The NNP-I1000 is based on Intel’s latest 10-nanometer chip architecture. The company essentially took a 10-nanometer central processing unit, stripped it down to just two processing cores and added on 12 “interference engines” engineered to run AI software.
The result is a chip that Intel said can perform as many as 3,600 inferences per second. That performance was logged with ResNest50, an AI model commonly used to assess processor performance. The benchmark result breaks down to 4.8 trillion operations per watt, which Intel claimed makes the NNP-I1000 the most power-efficient chip in the category.
Already, companies such as Facebook Inc. and Baidu Inc. are itching to deploy both the Nervana chips, according to Intel. Misha Smelyanskiy, director of AI at Facebook, said the chips are key to speeding up the social network giant’s AI work, such as processing 6 billion translations a day.
Capping off today’s hardware announcements is a new version of Intel’s Movidius Myriad vision processing unit, code-named Keem Bay. The chip is designed to power AI image and video processing systems inside low-power devices, such as a drone that relies on machine learning to maneuver around obstacles.
Intel said the latest Movidius VPU provides more than 10 times the inference performance of the previous-generation chip. At the same time, it’s up to six times more power-efficient than products from competitors such as Nvidia’s TX2 chip.
“Purpose-built hardware like Intel Nervana NNPs and Movidius Myriad VPUs are necessary to continue the incredible progress in AI,” Rao said. “There is no single approach for AI.”
Indeed, said Jonathan Ballon, vice president of Intel’s Internet of Things Group, in a dig at Nvidia’s GPUs, emphasized the importance of creating chips that at “purpose-built” for AI and machine learning. GPUs were originally and still are used for speeding up computer graphics.
Moreover, Rao underscored the importance of associated software to use the chips’ capabilities. Nvidia’s CUDA, a software platform and set of application programming interfaces for parallel computing and neural networking, for instance, has been a key to its AI ascendance. In particular, Intel announced Dev Cloud for the Edge, which enables developers to test algorithms on Intel hardware before buying it.
All that said, Rao spent a lot of time this morning talking up Intel’s traditional processors, saying they’re the basis for most AI work and remain so, accounting for the bulk of what he said is already a $3.5 billion AI business this year, up from $1 billion in 2017.
“It all starts with the CPU,” he said. “Xeon is the backbone of AI and it’s also the backbone of the data center.”
Intel is investing a lot into training and inference as many workloads shift machine learning, noted Patrick Moorhead, president and principal analyst at Moor Insights & Strategy. “It’s good to see these parts finally make it to market, and I’m looking forward to see how they operate in production workloads and how the tool chain looks in terms of effort versus the incumbent,” he said.
With reporting from Robert Hof
THANK YOU