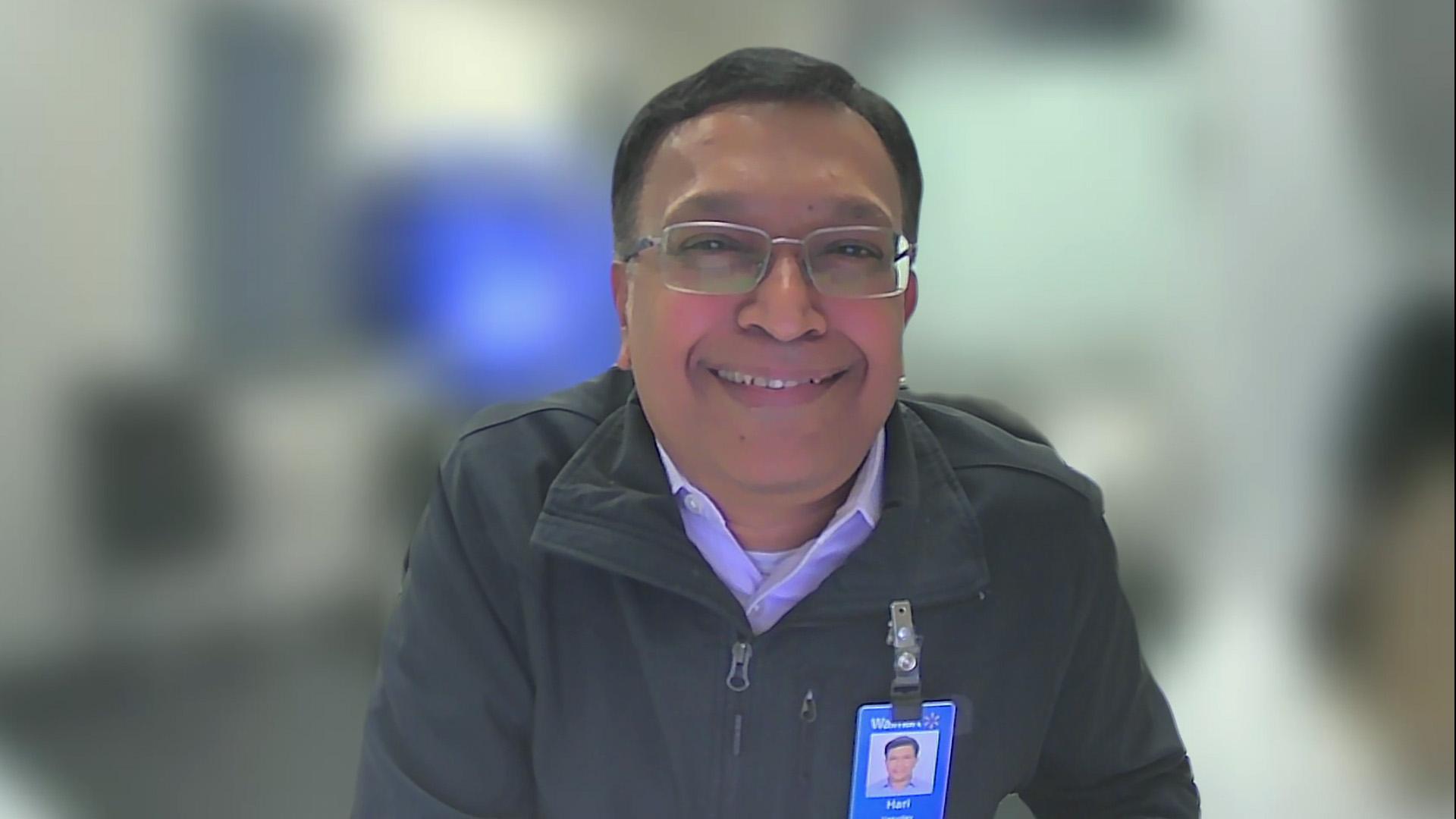
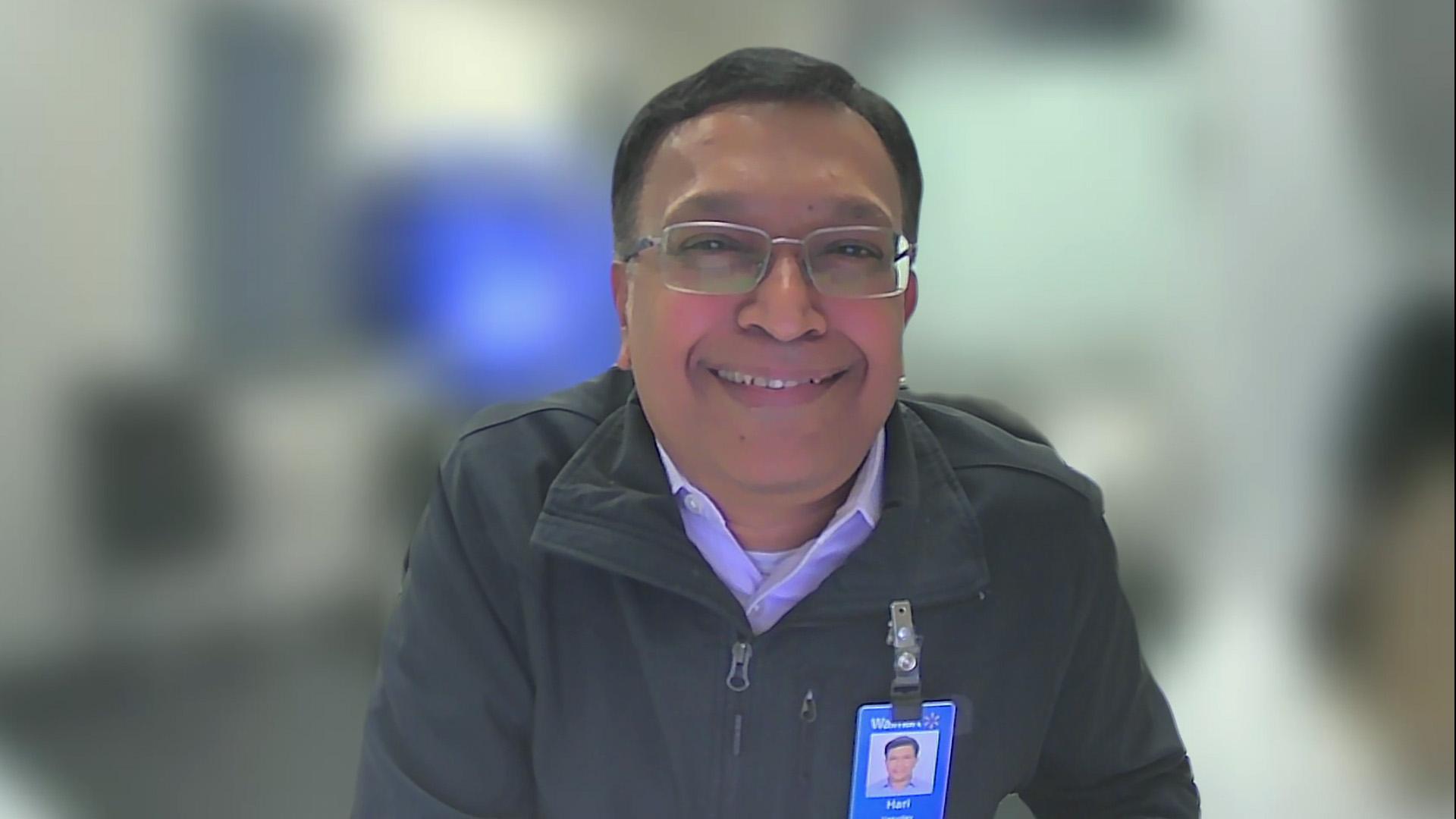
Not many enterprises can tackle the complexity and expense of building a full-scale machine learning platform from the ground up, but not many are Walmart Inc.
The retail giant intends to infuse artificial intelligence throughout its operations and wants the maximum flexibility to use whatever models, toolkits and cloud resources its developers prefer. The result is Element, a full-fledged machine learning platform that is cloud- and large language model-independent.
The technology enables automated prompt training and engineering in an accurate, low-cost manner, according to Hari Vasudev (pictured), executive vice president of the global tech platform at Walmart Global Tech, the technology and business services organization within Walmart.
“Building our own platform allows us to adopt best-of-breed technologies from open source and cloud providers,” Vasudev said. “We can put in our own governance layer because, as the world’s most trusted retailer, we want to make sure that we’re being transparent about how we use people’s data. Ultimately, it allows us to develop AI sustainably, ethically and responsibly.”
Vasudev described Element as having “a classic layered architecture.” At the base is a connector to managed LLMs. Above that is an LLM gateway “that allows us to do large-scale distributed model training and inferencing,” Vasudev said. “It allows you to route requests to any of the LLMs, whether managed LLMs or open source with intelligence for optimized cost and performance tuning and inferencing.”
A graphics processing unit recommender automates the use of GPUs and models. “It allows us to do low-cost, high-accuracy prompt training and automated prompt engineering,” Vasudev said. On top of that is a governance layer that detects errors and biases and guards against hallucinations.
Security guardrails ensure that content is moderated appropriately and sensitive content is filtered. The top layer supports standardized interfaces for developers.
The architecture allows Walmart to customize AI processing according to the use case. For example, a platform called Converse is built for chat bots and other conversational applications. Other platforms can support tasks like machine vision and generative search.
Vasudev outlined Element at the “Supercloud 6: AI Innovators” event, during an exclusive broadcast on theCUBE, SiliconANGLE Media’s livestreaming studio.
Element isn’t just another AI platform — it’s a comprehensive ecosystem designed to revolutionize machine learning and AI development, according to Vasudev. Element ensures high-accuracy model training while prioritizing governance and security.
“The governance layer allows us to manage things like fairness monitoring, mitigate the effects of hallucination and so on,” Vasudev said. At the security layer, “it’s all about having keyword blocks, making sure that we are moderating content appropriately and making sure that we are filtering sensitive content out.”
Walmart’s motivation to build Element from scratch lies in the company’s increasing AI reliance and usage over the years. With hundreds of data scientists scattered across various geographies and business units, the company faced the challenge of streamlining AI development for speed and sustainability. By building Element to span multiple public clouds and its own private cloud, Walmart aims for cloud vendor agnosticism, model flexibility and enhanced governance, paving the way for sustainable and ethical AI development at scale, according to Vasudev.
With thousands of developers worldwide, Walmart prioritizes flexibility and reuse. “We have had hundreds of data scientists and machine learning engineers in different geographies,” Vasudev said. “It becomes very hard to develop and execute AI and ML projects with speed and sustainability.”
The multicloud architecture frees developers to use whatever cloud resources they need with automated provisioning and cost optimization. Its multicloud strategy, called the “triplet model,” strategically positions combinations of public cloud, private cloud and edge nodes to give it optimal flexibility in workload deployment.
“Each region has private and public clouds,” Vasudev said. “A triplet is deployed across the west, east and south-central regions to allow us to seamlessly integrate and run cloud-agnostic ML workloads across native clouds and regions.”
The company uses the open-source OneOps cloud management platform to standardize virtual machine management. The internally developed Walmart Cloud Native Platform provides an orchestration layer based on Kubernetes “that allows us to scale applications across a private and public cloud essentially,” Vasudev said.
A data abstraction layer automates workload placement and data migration across different cloud providers and extends into the global network of stores, distribution centers, fulfillment centers and clubs. “Effectively, what we have done by leveraging the triplet cloud is enable nearly 10,000 edge cloud nodes at all of our facilities,” he said.
The result is an MLOps deployment framework that enables data scientists to “tap into on-demand infrastructure, whether that’s GPUs, CPUs or TPUs [tensor processing units] to very quickly deploy multiple models in parallel on a multicloud regional infrastructure in a very short time,” he said.
Walmart also built a gen AI playground, “where our own developers can play with different use cases, applications and pilots built on top of Element,” he said. “We are hoping that can spark innovation at scale by also reducing the overall cost and essentially making the process of developing applications a whole lot faster.”
Model deployment is never a one-and-done proposition. Performance tends to degrade over time and factors like seasonality and fashion trends influence results. “If I’m searching for hats in the summer it should provide me a very different set of choices than if I searched in the winter,” Vasudev said. “We’re continuously testing our algorithms to remove cognitive dissonance between the search results we provide our customers and what they expect to see.”
Element anchors several AI initiatives that are already in production. My Assistant is an in-house generative AI tool that can be used to write job descriptions, summarize data, write emails, guide interviews and generate ideas, among other tasks. It’s trained on company data so that “rather than giving you generic answers about healthcare, it’ll give you very specific answers highlighting the options available within Walmart benefit plans,” Vasudev said.
The Element-based Developer Experience application enables developers to find information curated by other developers quickly. It’s part of a companywide effort to use standardized tools, frameworks, development processes and libraries to maximize code reuse.
And while most enterprises have tread cautiously in exposing generative AI to customers, Walmart is forging ahead with the search application currently available on its IoS app. Customers can enter props like “help me plan a March Madness watch party” and get a customized basket of recommendations fine-tuned to their preferred menu items and brands.
“It will go through literally hundreds of millions of catalog items and create a highly customized basket for you,” Vasudev said. “It may even have your own personal brand preferences based on the knowledge we have gathered about your shopping experiences.”
Ultimately, platforms like Element will transform customer and employee experience, Vasudev said. “You’re going to be able to do personalization at a very large scale,” he said. The environment will become increasingly media-rich and “you or I will get exactly what we want with experiences super-tailored for us.”
Here’s the complete video interview, part of SiliconANGLE’s and theCUBE Research’s coverage of the “Supercloud 6: AI Innovators” event:
THANK YOU