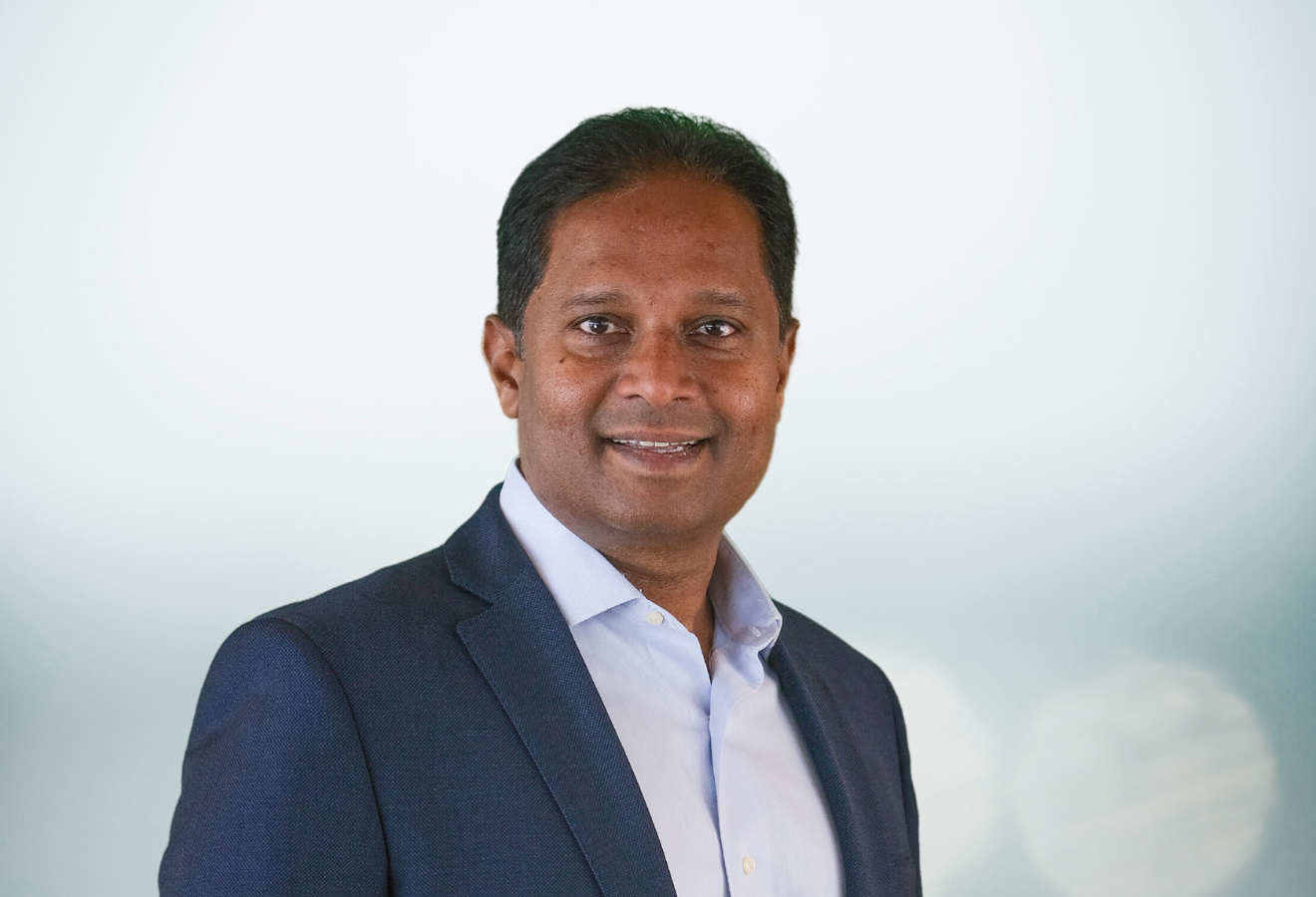
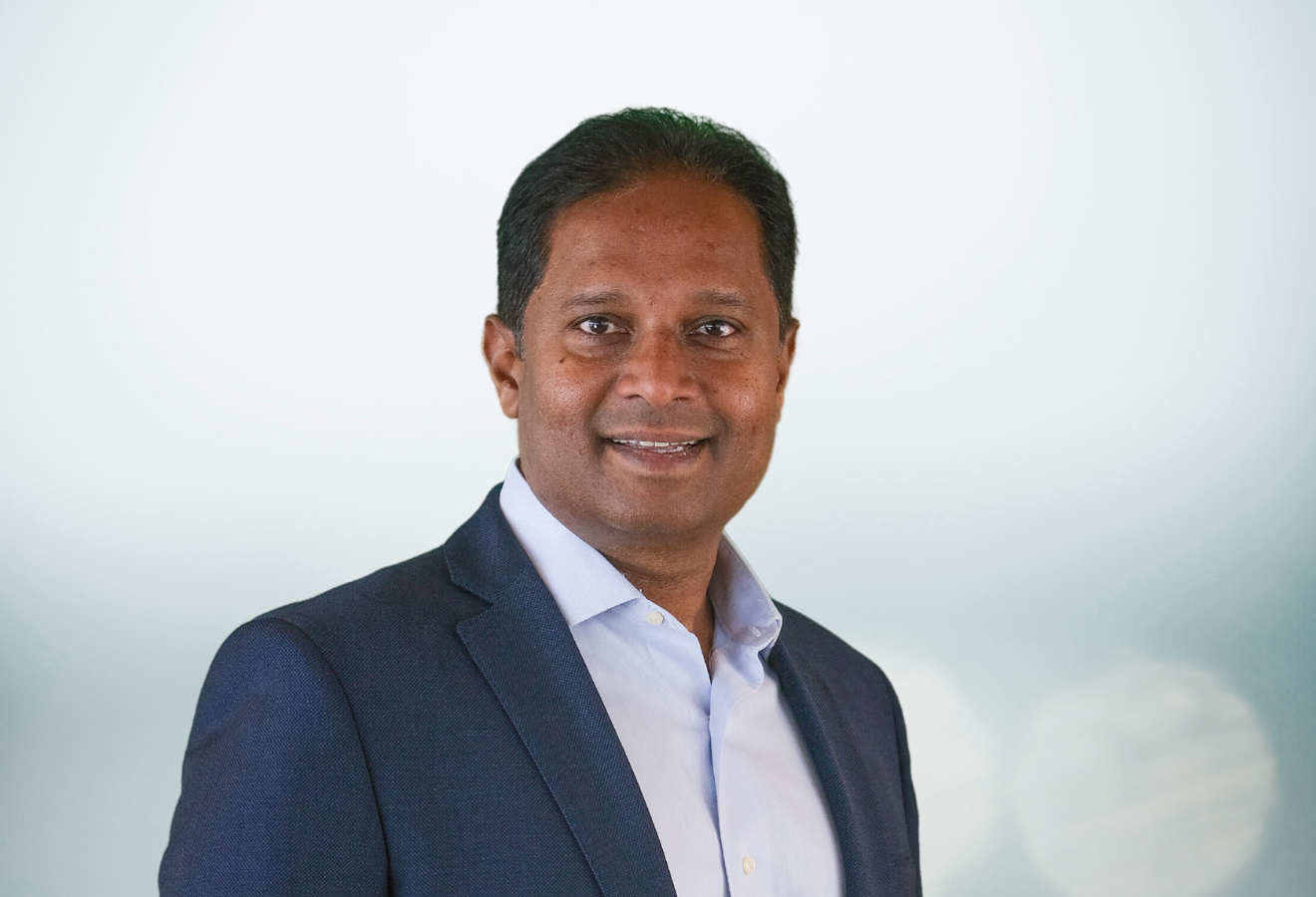
In the process of completing 25 acquisitions and building a trove of information on more than 1 billion consumers across 30 countries over the past 56 years, TransUnion LLC had created a lot of data siloes.
Now it’s attacking that problem with a massive data lake intended to support all of its analytics, application development and artificial intelligence use cases around the globe. OneTru, which was officially launched earlier this month, combines data and analytic assets from the firm’s credit risk, marketing and fraud prevention businesses into a single, layered and unified environment with a consistent set of formats, tools, and governance constructs.
Built on a hybrid cloud infrastructure, OneTru enables the company to shift workloads easily between clouds with governance rules that can be applied flexibly depending on where the workload lives. That’s important in a highly regulated financial services industry.
“You can think of it as a data lake with very specific product views and the appropriate compliance rules embedded that a family of products can leverage,” said Venkat Achanta (pictured), TransUnion’s chief technology, data and analytics officer.
TransUnion will spend the next 18 months migrating data into the platform. “That is a big transformation,” Achanta said. “People will work from different data sets, but they will be managed consistently.”
Part of the impetus for building OneTru was to unite the data housed in the company’s three principal lines of business into a massive common data lake that can yield a better understanding of consumer behaviors, improve credit scoring and fraud detection and open up new lines of business.
For example, the consolidated platform will enable graphs to be built that marry structured data such as offline identities with unstructured images and behavioral data. That will let the company’s 65,000 business customers identify fraudsters more accurately with fewer false positives.
Early indications are that OneTru is delivering on its promise. “The resources and effort to create a marginal new product went from months to weeks,” Achanta said. “We think we can double innovation capacity.”
OneTru’s architecture consists of four layers. The data management layer will eventually house all the company’s public, proprietary, online, offline, credit and noncredit data. The identity layer consolidates fragmented data elements scattered around different data stores into a single digital identity.
The analytics layer supports data analysis and machine learning applications across credit, marketing and fraud mitigation. The delivery layer serves up a unified data governance framework and the permission-based access controls essential in a regulated industry.
Building OneTru was a multiyear project that consolidated thousands of standards and pipelines. TransUnion built much of the plumbing that automatically transforms and validates data in real time as it enters the data lake.
“The platform takes care of compliance, security, governance and data ingestion,” Achanta said. “We can determine if input data is a phone number or an address, automatically inspect the schema and suggest validations to attach. This gives us the ability not only to ingest the data but to check the quality.” The common architecture with granular metadata tags and provenance information allows compliance rules to be applied automatically.
“Eighty percent of checks and validations are suggested by the platform with a human in the loop to say the data has been sampled and inspected,” he said.
The multicloud architecture allows the global company to deploy functionality customized to different regional and regulatory environments.
“You can think of it as a data lake with very specific product views and the appropriate compliance rules that a family of products can leverage,” Achanta said. In India, for example, where strict data residency rules apply, he said, “we instantiate a new instance of this platform within the cloud providers in the India region so they can manage data locally. They use the exact same platform capabilities and provenance, but it’s a different instance.”
Developers will work with a common set of tools for data management, application development and machine learning model training. TransUnion has already trained several large language models in its private cloud with company-specific information.
A natural language interface replaces menus and query generators for many daily analytics tasks. TransUnion is phasing out all of its legacy business intelligence platforms. “Users only have to focus on the use case and what [application program interfaces] they need,” Achanta said. “We have a very low-code platform for data management, governance and analytics. As a result, we can innovate faster.”
He demonstrated how TransUnion’s generative AI can simplify complex analysis. When asked to identify the most common reasons customers take out a loan in a particular region, the LLM first interprets the intent of the person submitting the query, maps that to another LLM trained on the metadata and data dictionaries used for fine-tuning, converts the query into an SQL statement, and returns the results: 537 home loans, 75 debt consolidation loans, and 40 home improvement loans.
“We can control the input and output to reduce the risk of hallucinations,” he said. “We verticalized the model for our specific purpose and created a low-code platform so you can point and click to do many things and actually talk to it.”
Another model is trained on a large corpus of documentation, including user guides, text documents in various formats and videos. Finding an important piece of information used to involve a lot of manual searching, but an AI user guide has assumed most of the grunt work.
“It pulls images from the documentation on how to do that work, pulls the page references and even writes a natural-language step-by-step process,” Achanta said. The model also explains its reasoning and documents each step for compliance purposes. In TransUnion’s business, he said, “it is very important to have high explainability for regulatory reasons. Nothing can be a black box.”
The migration also allows the company to integrate acquired systems that have sometimes operated autonomously for years. “Prior to this transformation, we let the core platforms continue to run and integrated what needed to be integrated,” he said. “Now we’re deeply leveraging the same platform to migrate the data and shut down the platforms from the acquisitions.”
In building its own platform from scratch, TransUnion is taking a similar approach to Walmart Inc., which built a full-fledged, multicloud and large language model-independent machine learning platform that will anchor its analytics and AI development activities across the company.
That’s no accident. Prior to joining Neustar Inc., the identity resolution company TransUnion acquired in 2021, Achanta was the retail giant’s chief data officer.
THANK YOU