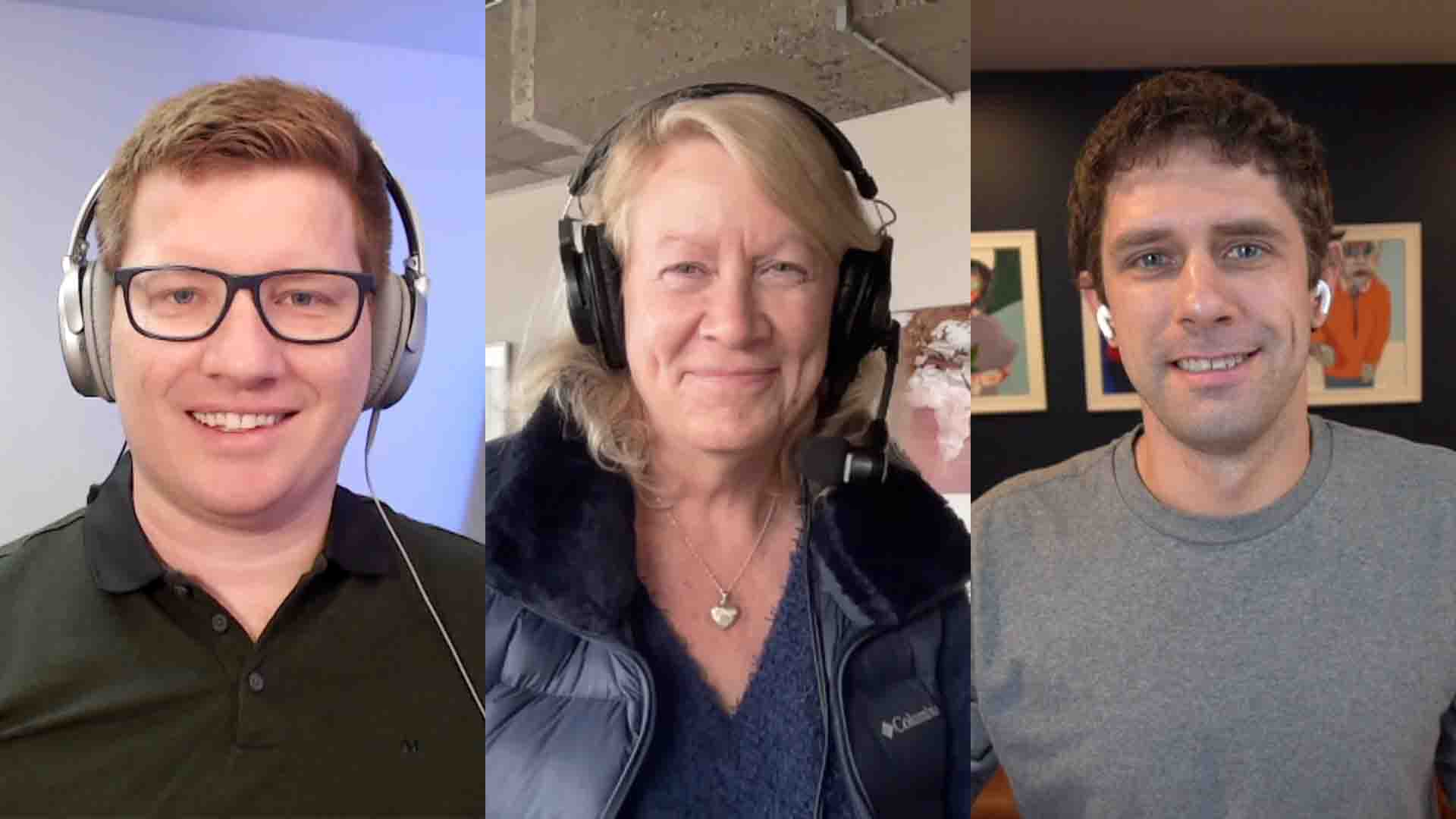
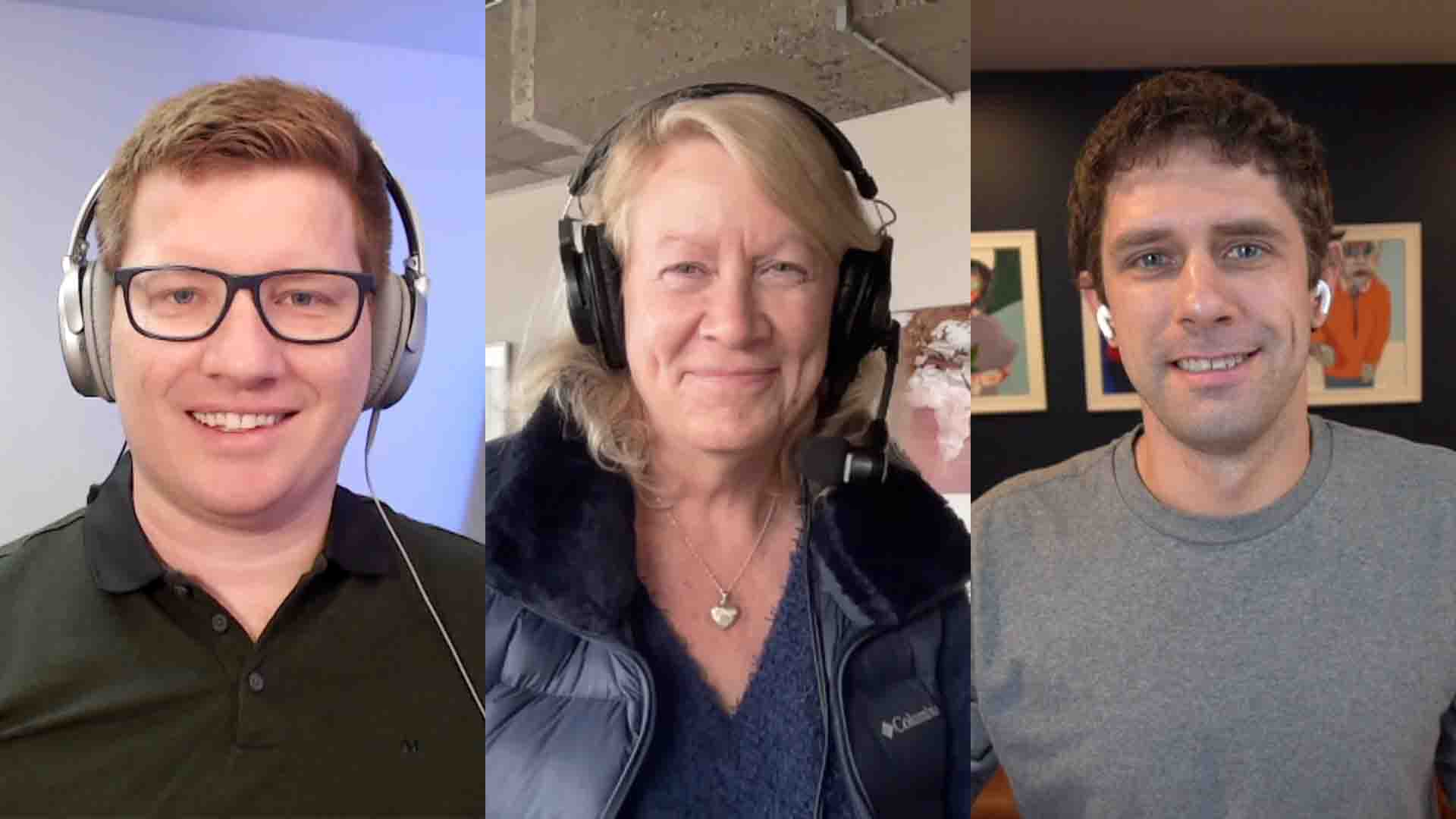
The use of genomics for drug discovery and personalized treatment has allowed scientists to develop more targeted therapies. It has also created enormous quantities of data.
One human genome sequence produces approximately 200 gigabytes of raw data, and 100 million sequenced genomes will ultimately account for 20 billion gigabytes. Cloud computing has become an important factor in the ability to process genomic analyses, but scientists and computing researchers have found that even the adoption of a distributed cloud model presents its own set of challenges when it comes to genomic sequencing.
“There’s big data, and then there’s genomic data,” said Lynn Langit (pictured, center), chief executive officer of Lynn Langit Consulting LLC. “I’ve worked with clients that have broken datacenters, broken cloud provider datacenters because of the daily volume they are putting in. You’ve got all of these bioinformatics researchers that are used to single machine, and suddenly they have to deal with distributed compute. It’s a wild time to be in this space.”
Langit spoke with theCUBE industry analyst John Furrier during the AWS Startup Showcase: “Data as Code — The Future of Enterprise Data and Analytics” event, an exclusive broadcast on theCUBE, SiliconANGLE Media’s livestreaming studio. She was joined by Peter Hanssens (pictured, left), founder of Cloudseeder Group Pty. Ltd., and Alex DeBrie (pictured, right), founder and principal at DeBrie Advisory, and they discussed how organizations are taking advantage of a new cloud environment, the rise of interest in a data mesh, leveraging advances in quantum computing, and a continued focus on managing data teams. (* Disclosure below.)
The compute challenges presented by the genomic sequencing industry are part of a broader story that centers on how technology is reshaping enterprise IT infrastructure for a cloud-native world.
“You’re seeing these cool databases come out that really take advantage of this new cloud environment,” DeBrie said. “You’re paying for capacity, you’re paying for throughput, you’re able to scale up and down and you’re not managing individual instances. You have scalability, and you have elasticity of the cloud.”
This elasticity has led to the development of new models in the cloud for handling vast amounts of data, such as the massive stores generated by genomic sequencing. One of these models involves the data mesh, an architectural and organizational model designed to address the shortcomings caused by the challenges of handling vast amounts of data.
“What we’re seeing is that data engineering teams and data teams more broadly are this organizational bottleneck,” Hanssens explained. “Data mesh is all about breaking down that bottleneck and decentralizing that work, shifting that work back onto development teams, who often have more of the context. There are still a lot of challenges around the transformation layer, getting data from raw, landed data into business domains. That talks to what data mesh is all about.”
There has also been a perceptible increase in use cases within the quantum computing field as IT organizations seek to find ways for leveraging advanced technologies in the handling of computationally intensive workloads.
In 2020, AWS announced general availability for its Amazon Braket quantum computing service. The fully managed service allows developers and researchers to explore new solutions with quantum machines from three different hardware providers.
“I’ve been doing quite a bit of experimentation around Amazon Braket for quantum processing units,” Langit said. “It’s not for everybody and the learning curve is pretty daunting, but there are some use cases out there. The QPU algorithm pipeline performed more accurately and faster. I think bursting to quantum is something to pay attention to.”
The growth of cloud computing has fostered a new dynamic in which multiple databases must be managed and coordinated cross-organizationally, requiring a team approach.
“Gone are the days where you have a single relational database that is serving operational queries for your users and analytics queries for internal teams; it’s now split up into purpose-built databases,” DeBrie noted. “Now you’ve got two different teams managing it, and they are designing their data model for different things. You need to suck that data out and get it elsewhere so your business analyst can crunch through some of that. Building empathy across those teams is helpful.”
Watch the complete video interview below, part of SiliconANGLE’s and theCUBE’s coverage of the AWS Startup Showcase: “Data as Code — The Future of Enterprise Data and Analytics” event:
(* Disclosure: TheCUBE is a paid media partner for the AWS Startup Showcase: “Data as Code — The Future of Enterprise Data and Analytics” event. Neither AWS, a sponsor for theCUBE’s event coverage, nor other sponsors have editorial control over content on theCUBE or SiliconANGLE.)
THANK YOU